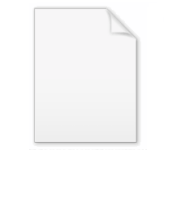
Censored regression model
Encyclopedia
Censored regression models commonly arise in econometrics in cases where the variable of
interest is only observable under certain conditions. A common example is labor supply. Data are frequently available on the hours worked by employees, and a labor supply model estimates the relationship between hours worked and characteristics of employees such as age, education and family status. However, the results of such estimates, undertaken using linear regression
will be biased by the fact that for people who are unemployed it is not possible to observe the number of
hours they would have worked had they had employment. Still we know age, education and family status for those observations.
A famous model to deal with censored data is the Tobit model
as well as variations such as the
tobit type II-IV model.
These and other censored regression models are often confused with truncated regression model
s.
Truncated regression models are used for data where whole observations are missing
so that the value for the dependent and the independent variable is unknown.
Censored regression models are used for data, where only the value for the dependent variable
(hours of work in the example above)
is unknown while the value of the independent variable (age, education, family status) is still available -- just like a censored letter
or newspaper where some words are blackened out but other words are still readable.
Censored regression models are usually estimated using likelihood estimation.
The general validity of this approach has been shown by Schnedler (2005) who also provides
a method to find the likelihood for a broad class of applications.
interest is only observable under certain conditions. A common example is labor supply. Data are frequently available on the hours worked by employees, and a labor supply model estimates the relationship between hours worked and characteristics of employees such as age, education and family status. However, the results of such estimates, undertaken using linear regression
Linear regression
In statistics, linear regression is an approach to modeling the relationship between a scalar variable y and one or more explanatory variables denoted X. The case of one explanatory variable is called simple regression...
will be biased by the fact that for people who are unemployed it is not possible to observe the number of
hours they would have worked had they had employment. Still we know age, education and family status for those observations.
A famous model to deal with censored data is the Tobit model
Tobit model
The Tobit model is a statistical model proposed by James Tobin to describe the relationship between a non-negative dependent variable y_i and an independent variable x_i....
as well as variations such as the
tobit type II-IV model.
These and other censored regression models are often confused with truncated regression model
Truncated regression model
Truncated regression models arise in many applications of statistics, for example in econometrics, in cases where observations with values in the outcome variable below or above certain thresholds systematically excluded from the sample...
s.
Truncated regression models are used for data where whole observations are missing
so that the value for the dependent and the independent variable is unknown.
Censored regression models are used for data, where only the value for the dependent variable
(hours of work in the example above)
is unknown while the value of the independent variable (age, education, family status) is still available -- just like a censored letter
or newspaper where some words are blackened out but other words are still readable.
Censored regression models are usually estimated using likelihood estimation.
The general validity of this approach has been shown by Schnedler (2005) who also provides
a method to find the likelihood for a broad class of applications.
See also
- Linear regressionLinear regressionIn statistics, linear regression is an approach to modeling the relationship between a scalar variable y and one or more explanatory variables denoted X. The case of one explanatory variable is called simple regression...
- Nonlinear regressionNonlinear regressionIn statistics, nonlinear regression is a form of regression analysis in which observational data are modeled by a function which is a nonlinear combination of the model parameters and depends on one or more independent variables...
- Nonparametric regressionNonparametric regressionNonparametric regression is a form of regression analysis in which the predictor does not take a predetermined form but is constructed according to information derived from the data...