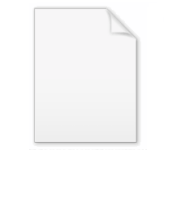
Decomposing of time series
Encyclopedia
The decomposition of time series is a statistical method that deconstructs a time series
into notional components. There are two principal types of decomposition which are outlined below.
. It seeks to construct, from an observed time series, a number of component series (that could be used to reconstruct the original by additions or multiplications) where each of these has a certain characteristic or type of behaviour. For example, monthly or quarterly economic time series are usually decomposed into:
An example of statistical software for this type of decomposition is the program BV4.1 that is based on the so-called Berlin procedure
.
Kendall shows an example of a decomposition into smooth, seasonal and irregular factors for a set of data containing values of the monthly aircraft miles flown by UK airlines.
.
Time series
In statistics, signal processing, econometrics and mathematical finance, a time series is a sequence of data points, measured typically at successive times spaced at uniform time intervals. Examples of time series are the daily closing value of the Dow Jones index or the annual flow volume of the...
into notional components. There are two principal types of decomposition which are outlined below.
Decomposition based on rates of change
This is an important technique for all types of time series analysis, especially for seasonal adjustmentSeasonal adjustment
Seasonal adjustment is a statistical method for removing the seasonal component of a time series that is used when analyzing non-seasonal trends. It is normal to report un-adjusted data for current unemployment rates, as these reflect the actual current situation...
. It seeks to construct, from an observed time series, a number of component series (that could be used to reconstruct the original by additions or multiplications) where each of these has a certain characteristic or type of behaviour. For example, monthly or quarterly economic time series are usually decomposed into:
- the Trend Component
that reflects the long term progression of the series (secular variation
Secular variationThe secular variation of a time series is its long-term non-periodic variation . Whether something is perceived as a secular variation or not depends on the available timescale: a secular variation over a time scale of centuries may be part of a periodic variation over a time scale of millions of...
) - the Cyclical Component
that describes repeated but non-periodic fluctuations, possibly caused by the economic cycle
- the Seasonal Component
reflecting seasonality
SeasonalityIn statistics, many time series exhibit cyclic variation known as seasonality, periodic variation, or periodic fluctuations. This variation can be either regular or semi regular....
(Seasonal variation) - the Irregular Component
(or "noise") that describes random, irregular influences. Compared to the other components it represents the residuals of the time series.
An example of statistical software for this type of decomposition is the program BV4.1 that is based on the so-called Berlin procedure
Berlin procedure
The so-called Berlin procedure is a mathematical procedure for time series decomposition and seasonal adjustment of monthly and quarterly economic time series. The mathematical foundations of the procedure were developed in 1960's at the Technical University of Berlin and the German Institute...
.
Kendall shows an example of a decomposition into smooth, seasonal and irregular factors for a set of data containing values of the monthly aircraft miles flown by UK airlines.
Decomposition based on predictability
The theory of time series analysis make use of the idea of decomposing a times series into deterministic and non-deterministic components (or predictable and unpredictable components). See Wold's theorem and Wold decompositionWold decomposition
In operator theory, the Wold decomposition, named after Herman Wold, or Wold-von Neumann decomposition, after Wold and John von Neumann, is a classification theorem for isometric linear operators on a given Hilbert space...
.