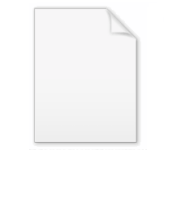
Dimension (data warehouse)
Encyclopedia
In a data warehouse
, a dimension is a data element
that categorizes each item in a data set
into non-overlapping regions. A data warehouse dimension provides the means to "slice and dice" data in a data warehouse. Dimensions provide structured labeling information to otherwise unordered numeric measures. For example, "Customer", "Date", and "Product" are all dimensions that could be applied meaningfully to a sales receipt. A dimensional data element
is similar to a categorical variable in statistics.
The primary function of dimensions is threefold: to provide filtering, grouping and labeling. For example, in a data warehouse where each person is categorized as having a gender of male, female or unknown, a user of the data warehouse would then be able to filter or categorize each presentation or report by either filtering based on the gender dimension or displaying results broken out by the gender.
Each dimension in a data warehouse may have one or more hierarchies applied to it. For the "Date" dimension, there are several possible hierarchies: "Day > Month > Year", "Day > Week > Year", "Day > Month > Quarter > Year", etc.
structure, attributes, domain values, definitions and concepts in each implementation. A conformed dimension cuts across many facts.
Dimensions are conformed when they are either exactly the same (including keys) or one is a perfect subset of the other. Most important, the row headers produced in the answer sets from two different conformed dimensions must be able to match perfectly.
Conformed dimensions are either identical or strict mathematical subsets of the most granular, detailed dimension. Dimension tables are not conformed if the attributes are labeled differently or contain different values. Conformed dimensions come in several different flavors. At the most basic level, conformed dimensions mean exactly the same thing with every possible fact table to which they are joined. The date dimension table connected to the sales facts is identical to the date dimension connected to the inventory facts.
A Junk Dimension is a dimension table consisting of attributes that do not belong in the fact table or in any of the existing dimension tables. The nature of these attributes is usually text or various flags, e.g. non-generic comments or just simple yes/no or true/false indicators. These kinds of attributes are typically remaining when all the obvious dimensions in the business process have been identified and thus the designer is faced with the challenge of where to put these attributes that do not belong in the other dimensions.
One solution is to create a new dimension for each of the remaining attributes, but due to their nature, it could be necessary to create a vast number of new dimensions resulting in a fact table with a very large number of foreign keys. The designer could also decide to leave the remaining attributes in the fact table but this could make the row length of the table unnecessarily large if, for example, the attributes is a long text string.
The solution to this challenge is to identify all the attributes and then put them into one or several Junk Dimensions. One Junk Dimension can hold several true/false or yes/no indicators that have no correlation with each other, so it would be convenient to convert the indicators into a more describing attribute. An example would be an indicator about whether a package had arrived, instead of indicating this as “yes” or “no”, it would be converted into “arrived” or “pending” in the junk dimension. The designer can choose to build the dimension table so it ends up holding all the indicators occurring with every other indicator so that all combinations are covered. This sets up a fixed size for the table itself which would be 2^x rows, where x is the number of indicators. This solution is appropriate in situations where the designer would expect to encounter a lot of different combinations and where the possible combinations are limited to an acceptable level. In a situation where the number of indicators are large, thus creating a very big table or where the designer only expect to encounter a few of the possible combinations, it would be more appropriate to build each row in the junk dimension as new combinations are encountered. To limit the size of the tables, multiple junk dimensions might be appropriate in other situations depending on the correlation between various indicators.
Junk dimensions are also appropriate for placing attributes like non-generic comments from the fact table. Such attributes might consist of data from an optional comment field when a customer places an order and as a result will probably be blank in many cases. Therefore the junk dimension should contain a single row representing the blanks as a surrogate key that will be used in the fact table for every row returned with a blank comment field
A dimension key, such as a transaction number, invoice number, ticket number, or bill-of-lading number, that has no attributes and hence does not join to an actual dimension table. Degenerate dimensions are very common when the grain of a fact table represents a single transaction item or line item because the degenerate dimension represents the unique identifier of the parent. Degenerate dimensions often play an integral role in the fact table's primary key.
, representation term
s such as Indicator (a boolean true/false value), Code (a set of non-overlapping enumerated values) are typically used as dimensions. For example using the National Information Exchange Model
(NIEM) the data element name would be PersonGenderCode and the enumerated values would be male, female and unknown.
is frequently composed of both dimensions and measures
. These can then be placed into dimension
and fact table
s in a relational database
.
Since many fact tables
in a data warehouse are time series of observations, one or more date dimensions are often needed. One of the reasons to have date dimensions is to place calendar knowledge in the data warehouse instead of hard coded in an application. While a simple SQL date/timestamp is useful for providing accurate information about the time a fact was recorded, it can not give information about holidays, fiscal periods, etc. An SQL date/timestamp can still be useful to store in the fact table, as it allows for precise calculations.
Having both the date and time of day in the same dimension, may easily result in a huge dimension with millions of rows. If a high amount of detail is needed it is usually a good idea to split date and time into two or more separate dimensions. A time dimension with a grain of seconds in a day will only have 86400 rows. A more or less detailed grain for date/time dimensions can be chosen depending on needs. As examples, date dimensions can be accurate to year, quarter, month or day and time dimensions can be accurate to hours, minutes or seconds.
As a rule of thumb, time of day dimension should only be created if hierarchical groupings are needed or if there are meaningful textual descriptions for periods of time within the day (ex. “evening rush” or “first shift”).
If the rows in a fact table are coming from several timezones, it might be useful to store date and time in both local time and a standard time. This can be done by having two dimensions for each date/time dimension needed – one for local time, and one for standard time. Storing date/time in both local and standard time, will allow for analysis on when facts are created in a local setting and in a global setting as well. The standard time chosen can be a global standard time (ex. UTC
), it can be the local time of the business’ headquarter, or any other time zone that would make sense to use.
Data warehouse
In computing, a data warehouse is a database used for reporting and analysis. The data stored in the warehouse is uploaded from the operational systems. The data may pass through an operational data store for additional operations before it is used in the DW for reporting.A data warehouse...
, a dimension is a data element
Data element
In metadata, the term data element is an atomic unit of data that has precise meaning or precise semantics. A data element has:# An identification such as a data element name# A clear data element definition# One or more representation terms...
that categorizes each item in a data set
Data set
A data set is a collection of data, usually presented in tabular form. Each column represents a particular variable. Each row corresponds to a given member of the data set in question. Its values for each of the variables, such as height and weight of an object or values of random numbers. Each...
into non-overlapping regions. A data warehouse dimension provides the means to "slice and dice" data in a data warehouse. Dimensions provide structured labeling information to otherwise unordered numeric measures. For example, "Customer", "Date", and "Product" are all dimensions that could be applied meaningfully to a sales receipt. A dimensional data element
Data element
In metadata, the term data element is an atomic unit of data that has precise meaning or precise semantics. A data element has:# An identification such as a data element name# A clear data element definition# One or more representation terms...
is similar to a categorical variable in statistics.
The primary function of dimensions is threefold: to provide filtering, grouping and labeling. For example, in a data warehouse where each person is categorized as having a gender of male, female or unknown, a user of the data warehouse would then be able to filter or categorize each presentation or report by either filtering based on the gender dimension or displaying results broken out by the gender.
Each dimension in a data warehouse may have one or more hierarchies applied to it. For the "Date" dimension, there are several possible hierarchies: "Day > Month > Year", "Day > Week > Year", "Day > Month > Quarter > Year", etc.
Conformed dimension
A conformed dimension is a set of data attributes that have been physically implemented in multiple database tables using the samestructure, attributes, domain values, definitions and concepts in each implementation. A conformed dimension cuts across many facts.
Dimensions are conformed when they are either exactly the same (including keys) or one is a perfect subset of the other. Most important, the row headers produced in the answer sets from two different conformed dimensions must be able to match perfectly.
Conformed dimensions are either identical or strict mathematical subsets of the most granular, detailed dimension. Dimension tables are not conformed if the attributes are labeled differently or contain different values. Conformed dimensions come in several different flavors. At the most basic level, conformed dimensions mean exactly the same thing with every possible fact table to which they are joined. The date dimension table connected to the sales facts is identical to the date dimension connected to the inventory facts.
Junk dimension
A junk dimension is a convenient grouping of typically low-cardinality flags and indicators. By creating an abstract dimension, these flags and indicators are removed from the fact table while placing them into a useful dimensional framework.A Junk Dimension is a dimension table consisting of attributes that do not belong in the fact table or in any of the existing dimension tables. The nature of these attributes is usually text or various flags, e.g. non-generic comments or just simple yes/no or true/false indicators. These kinds of attributes are typically remaining when all the obvious dimensions in the business process have been identified and thus the designer is faced with the challenge of where to put these attributes that do not belong in the other dimensions.
One solution is to create a new dimension for each of the remaining attributes, but due to their nature, it could be necessary to create a vast number of new dimensions resulting in a fact table with a very large number of foreign keys. The designer could also decide to leave the remaining attributes in the fact table but this could make the row length of the table unnecessarily large if, for example, the attributes is a long text string.
The solution to this challenge is to identify all the attributes and then put them into one or several Junk Dimensions. One Junk Dimension can hold several true/false or yes/no indicators that have no correlation with each other, so it would be convenient to convert the indicators into a more describing attribute. An example would be an indicator about whether a package had arrived, instead of indicating this as “yes” or “no”, it would be converted into “arrived” or “pending” in the junk dimension. The designer can choose to build the dimension table so it ends up holding all the indicators occurring with every other indicator so that all combinations are covered. This sets up a fixed size for the table itself which would be 2^x rows, where x is the number of indicators. This solution is appropriate in situations where the designer would expect to encounter a lot of different combinations and where the possible combinations are limited to an acceptable level. In a situation where the number of indicators are large, thus creating a very big table or where the designer only expect to encounter a few of the possible combinations, it would be more appropriate to build each row in the junk dimension as new combinations are encountered. To limit the size of the tables, multiple junk dimensions might be appropriate in other situations depending on the correlation between various indicators.
Junk dimensions are also appropriate for placing attributes like non-generic comments from the fact table. Such attributes might consist of data from an optional comment field when a customer places an order and as a result will probably be blank in many cases. Therefore the junk dimension should contain a single row representing the blanks as a surrogate key that will be used in the fact table for every row returned with a blank comment field
A dimension key, such as a transaction number, invoice number, ticket number, or bill-of-lading number, that has no attributes and hence does not join to an actual dimension table. Degenerate dimensions are very common when the grain of a fact table represents a single transaction item or line item because the degenerate dimension represents the unique identifier of the parent. Degenerate dimensions often play an integral role in the fact table's primary key.
Role-playing dimensions
Dimensions are often recycled for multiple applications within the same database. For instance, a "Date" dimension can be used for "Date of Sale", as well as "Date of Delivery", or "Date of Hire". This is often referred to as a "role-playing dimension".Use of ISO representation terms
When referencing data from a metadata registry such as ISO/IEC 11179ISO/IEC 11179
ISO/IEC 11179 is an international standard for representing metadata for an organization in a metadata registry.- Intended purpose :...
, representation term
Representation term
A representation term is a word, or a combination of words, that semantically represent the data type of a data element. A representation term is commonly referred to as a class word by those familiar with data dictionaries...
s such as Indicator (a boolean true/false value), Code (a set of non-overlapping enumerated values) are typically used as dimensions. For example using the National Information Exchange Model
National Information Exchange Model
The National Information Exchange Model is an XML-based information exchange framework from the United States. NIEM represents a collaborative partnership of agencies and organizations across all levels of government and with private industry...
(NIEM) the data element name would be PersonGenderCode and the enumerated values would be male, female and unknown.
Relationship to other components of a data warehouse
A data warehouse cubeOLAP cube
An OLAP cube is a data structure that allows fast analysis of data. It can also be defined as the capability of manipulating and analyzing data from multiple perspectives...
is frequently composed of both dimensions and measures
Measure (data warehouse)
In a data warehouse, a measure is a property on which calculations can be made using precomputed aggregates.-Example:...
. These can then be placed into dimension
Dimension table
In data warehousing, a dimension table is one of the set of companion tables to a fact table.The fact table contains business facts or measures and foreign keys which refer to candidate keys in the dimension tables....
and fact table
Fact table
In data warehousing, a fact table consists of the measurements, metrics or facts of a business process. It is often located at the centre of a star schema or a snowflake schema, surrounded by dimension tables....
s in a relational database
Relational database
A relational database is a database that conforms to relational model theory. The software used in a relational database is called a relational database management system . Colloquial use of the term "relational database" may refer to the RDBMS software, or the relational database itself...
.
Common patterns
Date and timeSince many fact tables
Fact table
In data warehousing, a fact table consists of the measurements, metrics or facts of a business process. It is often located at the centre of a star schema or a snowflake schema, surrounded by dimension tables....
in a data warehouse are time series of observations, one or more date dimensions are often needed. One of the reasons to have date dimensions is to place calendar knowledge in the data warehouse instead of hard coded in an application. While a simple SQL date/timestamp is useful for providing accurate information about the time a fact was recorded, it can not give information about holidays, fiscal periods, etc. An SQL date/timestamp can still be useful to store in the fact table, as it allows for precise calculations.
Having both the date and time of day in the same dimension, may easily result in a huge dimension with millions of rows. If a high amount of detail is needed it is usually a good idea to split date and time into two or more separate dimensions. A time dimension with a grain of seconds in a day will only have 86400 rows. A more or less detailed grain for date/time dimensions can be chosen depending on needs. As examples, date dimensions can be accurate to year, quarter, month or day and time dimensions can be accurate to hours, minutes or seconds.
As a rule of thumb, time of day dimension should only be created if hierarchical groupings are needed or if there are meaningful textual descriptions for periods of time within the day (ex. “evening rush” or “first shift”).
If the rows in a fact table are coming from several timezones, it might be useful to store date and time in both local time and a standard time. This can be done by having two dimensions for each date/time dimension needed – one for local time, and one for standard time. Storing date/time in both local and standard time, will allow for analysis on when facts are created in a local setting and in a global setting as well. The standard time chosen can be a global standard time (ex. UTC
Coordinated Universal Time
Coordinated Universal Time is the primary time standard by which the world regulates clocks and time. It is one of several closely related successors to Greenwich Mean Time. Computer servers, online services and other entities that rely on having a universally accepted time use UTC for that purpose...
), it can be the local time of the business’ headquarter, or any other time zone that would make sense to use.
See also
- Categorical variable
- Data warehouseData warehouseIn computing, a data warehouse is a database used for reporting and analysis. The data stored in the warehouse is uploaded from the operational systems. The data may pass through an operational data store for additional operations before it is used in the DW for reporting.A data warehouse...
- Dimension tableDimension tableIn data warehousing, a dimension table is one of the set of companion tables to a fact table.The fact table contains business facts or measures and foreign keys which refer to candidate keys in the dimension tables....
- Degenerate dimensionDegenerate dimension-The Kimball definition:According to Ralph Kimball , in a data warehouse, a degenerate dimension is a dimension key in the fact table that does not have its own dimension table, because all the interesting attributes have been placed in analytic dimensions...
- Slowly changing dimensionSlowly Changing DimensionDimension is a term in data management and data warehousing that refers to logical groupings of data such as geographical location, customer information, or product information...
- Fact tableFact tableIn data warehousing, a fact table consists of the measurements, metrics or facts of a business process. It is often located at the centre of a star schema or a snowflake schema, surrounded by dimension tables....
- ISO/IEC 11179ISO/IEC 11179ISO/IEC 11179 is an international standard for representing metadata for an organization in a metadata registry.- Intended purpose :...
- Measure (data warehouse)Measure (data warehouse)In a data warehouse, a measure is a property on which calculations can be made using precomputed aggregates.-Example:...
- MetadataMetadataThe term metadata is an ambiguous term which is used for two fundamentally different concepts . Although the expression "data about data" is often used, it does not apply to both in the same way. Structural metadata, the design and specification of data structures, cannot be about data, because at...