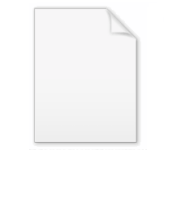
Firefly algorithm
Encyclopedia
The firefly algorithm is a metaheuristic algorithm
, inspired by the flashing behaviour of fireflies
. The primary purpose for a firefly's flash is to act as a signal system to attract other fireflies. Xin-She Yang
formulated this firefly algorithm by assuming:
The brightness should be associated with the objective function.
Firefly algorithm is a nature-inspired metaheuristic
optimization
algorithm
.
Begin
1) Objective function:
;
2) Generate an initial population of fireflies
;.
3) Formulate light intensity
so that it is associated with 
(for example, for maximization problems,
or simply
;
4) Define absorption coefficient
While (t
for i=1:n (all n fireflies)
for j=1:i (n fireflies)
if (
),
move firefly i towards j;
end if
Vary attractiveness with distance r via
;
Evaluate new solutions and update light intensity;
end for j
end for i
Rank fireflies and find the current best;
end while
Post-processing the results and visualization;
end
The main update formula for any pair of two fireflies
and
is
where
is a parameter controlling the step size, while
is a vector drawn from a Gaussian or other
distribution.
It can be shown that the limiting case
corresponds to the standard Particle Swarm Optimization (PSO). In fact, if the inner loop (for j) is removed and the brightness
is replaced by the current global best
, then FA essentially becomes the standard PSO.
should be related to the scales of design variables. Ideally, the
term should be order one, which
requires that
should be linked with scales. For example, one possible choice is to use
where
is the average scale of the problem. In case of scales vary significantly,
can be considered as a vector to suit different scales in different dimensions. Similarly,
should also be linked with scales. For example,
.
It is worth pointing out the above description does not include the randomness reduction. In fact, in actual implementation by most researchers, the motion of the fireflies is gradually reduced by an annealing-like randomness reduction via
where
. In some difficult problem, it may be helpful if you increase
at some stages, then reduce it when necessary. This non-monotonic variation of
will enable the algorithm to escape any local optima when in the unlikely case it might get stuck if randomness is reduced too quickly.
Parametric studies show that n (number of fireflies) should be about 15 to 40 for most problems.A python implementation is also available, though with limited functionalities.
Recent studies shows that the firefly algorithm is very efficient, and could outperform other metaheuristic algorithms including particle swarm optimization
.
Most metaheuristic algorithms may have difficulty in dealing with stochastic test functions, and it seems that firefly algorithm can deal with stochastic test functions very efficiently. In addition, FA is also better for dealing with noisy optimization problems with ease of implementation.
For image segmentation, the FA-based method is far more efficient to Otsu's method and recursive Otsu. Meanwhile, a good implementation of a discrete firefly algorithm for QAP problems has been carried out by Durkota.
For minimum cross entropy thresholding, firefly-based algorithm uses the least computation time
Recently, an evolutionary discrete FA has been developed for solving travelling salesman problem
s Further improvement in performance can be obtained by using preferential directions in firefly movements.
Algorithm
In mathematics and computer science, an algorithm is an effective method expressed as a finite list of well-defined instructions for calculating a function. Algorithms are used for calculation, data processing, and automated reasoning...
, inspired by the flashing behaviour of fireflies
Firefly
Lampyridae is a family of insects in the beetle order Coleoptera. They are winged beetles, and commonly called fireflies or lightning bugs for their conspicuous crepuscular use of bioluminescence to attract mates or prey. Fireflies produce a "cold light", with no infrared or ultraviolet frequencies...
. The primary purpose for a firefly's flash is to act as a signal system to attract other fireflies. Xin-She Yang
Xin-she Yang
Xin-She Yang has a DPhil in applied mathematics from Oxford UniversityHe is currently a Senior Research Scientist at National Physical Laboratory, andis the Editor-in-Chief of Int...
formulated this firefly algorithm by assuming:
- All fireflies are unisexual, so that one firefly will be attracted to all other fireflies;
- Attractiveness is proportional to their brightness, and for any two fireflies, the less brighter one will attract (and thus move) to the brighter one; however, the brightness can decrease as their distance increases;
- If there are no fireflies brighter than a given firefly, it will move randomly.
The brightness should be associated with the objective function.
Firefly algorithm is a nature-inspired metaheuristic
Metaheuristic
In computer science, metaheuristic designates a computational method that optimizes a problem by iteratively trying to improve a candidate solution with regard to a given measure of quality. Metaheuristics make few or no assumptions about the problem being optimized and can search very large spaces...
optimization
Optimization (mathematics)
In mathematics, computational science, or management science, mathematical optimization refers to the selection of a best element from some set of available alternatives....
algorithm
Algorithm
In mathematics and computer science, an algorithm is an effective method expressed as a finite list of well-defined instructions for calculating a function. Algorithms are used for calculation, data processing, and automated reasoning...
.
Algorithm description
The pseudo code can be summarized as:Begin
1) Objective function:

2) Generate an initial population of fireflies

3) Formulate light intensity


(for example, for maximization problems,


4) Define absorption coefficient

While (t
for j=1:i (n fireflies)
if (

move firefly i towards j;
end if
Vary attractiveness with distance r via

Evaluate new solutions and update light intensity;
end for j
end for i
Rank fireflies and find the current best;
end while
Post-processing the results and visualization;
end
The main update formula for any pair of two fireflies


where


distribution.
It can be shown that the limiting case



Implementation Guides
The

requires that






It is worth pointing out the above description does not include the randomness reduction. In fact, in actual implementation by most researchers, the motion of the fireflies is gradually reduced by an annealing-like randomness reduction via




Parametric studies show that n (number of fireflies) should be about 15 to 40 for most problems.A python implementation is also available, though with limited functionalities.
Recent studies shows that the firefly algorithm is very efficient, and could outperform other metaheuristic algorithms including particle swarm optimization
Particle swarm optimization
In computer science, particle swarm optimization is a computational method that optimizes a problem by iteratively trying to improve a candidate solution with regard to a given measure of quality...
.
Most metaheuristic algorithms may have difficulty in dealing with stochastic test functions, and it seems that firefly algorithm can deal with stochastic test functions very efficiently. In addition, FA is also better for dealing with noisy optimization problems with ease of implementation.
Discrete Firefly Algorithm (DFA)
A discrete version of Firefly Algorithm, namely, Discrete Firefly Algorithm (DFA) proposed recently by M. K. Sayadi, R. Ramezanian and N. Ghaffari-Nasab can efficiently solve NP-hard scheduling problems. DFA outperforms existing algorithms such as the ant colony algorithm.For image segmentation, the FA-based method is far more efficient to Otsu's method and recursive Otsu. Meanwhile, a good implementation of a discrete firefly algorithm for QAP problems has been carried out by Durkota.
Multiobjective FA
An important study of FA was carried out by Apostolopoulos and Vlachos, which provides a detailed background and analysis over a wide range of test problems including multobjective load dispatch problem.Lagrangian FA
An interesting, Lagrangian firefly algorithm is proposed to solve power system optimization unit commitment problems.Chaotic FA
A chaotic firefly algorithm (CFA) was developed and found to outperform the previously best-known solutions available.Hybrid Algorithms
A hybrid intelligent scheme has been developed by combining the firefly algorithm with the ant colony optimization.Digital Image Compression
Very recently, an FF-LGB algorithm for vector quantization of digital image compression was based on the firefly algorithm, which proves to be faster than other algorithms such as PSO-LBG and HBMO-LBG.For minimum cross entropy thresholding, firefly-based algorithm uses the least computation time
Eigenvalue optimization
Eigenvalue optimization of isospectral systems has solved by FA and multiple optimum points have been found efficiently.Feature selection and fault detection
Feature selection can be also carried out successfully using firefly algorithm. Real-time fault identification in large systems becomes viable, based on the recent work on fault identification with binary adaptive firefly optimization.Antenna Design
Firefly algorithms outperforms ABC for optimal design of linear array of isotropic sources.Structural Design
For mixed-variable problems, many optimization algorithms may struggle. However, firefly algorithm can efficiently sove optimization problems with mixed variables.Scheduling and TSP
Firefly-based algorithms for scheduling task graphs and job shop scheduling requires less computing than all other metaheuristics.Recently, an evolutionary discrete FA has been developed for solving travelling salesman problem
Travelling salesman problem
The travelling salesman problem is an NP-hard problem in combinatorial optimization studied in operations research and theoretical computer science. Given a list of cities and their pairwise distances, the task is to find the shortest possible tour that visits each city exactly once...
s Further improvement in performance can be obtained by using preferential directions in firefly movements.