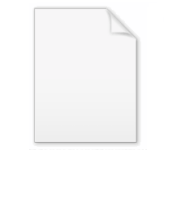
Inverse probability weighting
Encyclopedia
Inverse probability weighting is a statistical technique for calculating statistics standardized to a population different from that in which the data was collected. Study designs with a disparate sampling population and population of target inference (target population) are common in application. There may be prohibitive factors barring researchers from directly sampling from the target population such as cost, time, or ethical concerns. A solution to this problem is to use an alternate design strategy, e.g. stratified sampling. Weighting, when correctly applied, can potentially improve the efficiency and reduce the bias of unweighted estimators.
One very early weighted estimator is the Horvitz–Thompson estimator
of the mean. When the sampling probability is known, from which the sampling population is drawn from the target population, then the inverse of this probability is used to weight the mean. This approach has been generalized to many aspects of statistics under various frameworks. In particular, there are weighted likelihoods
, weighted estimating equations
, and weighted probability densities
from which a majority of statistics are derived. These applications codified the theory of other statistics and estimators such as marginal structural models, the standardized mortality ratio
, and the EM algorithm for coarsened or aggregate data.
Inverse probability weighting is also used to account for missing data when subjects with missing data cannot be included in the primary analysis.
With an estimate of the inclusion probability, or the probability that the factor would be measured in another measurement, inverse probability weighting can be used to inflate the weight for subjects who are under-represented due to a large degree of missing data.
One very early weighted estimator is the Horvitz–Thompson estimator
Horvitz–Thompson estimator
In statistics, the Horvitz–Thompson estimator, named after Daniel G. Horvitz and Donovan J. Thompson, is a method for estimating the mean of a superpopulation in a stratified sample. Inverse probability weighting is applied to account for different proportions of observations within strata...
of the mean. When the sampling probability is known, from which the sampling population is drawn from the target population, then the inverse of this probability is used to weight the mean. This approach has been generalized to many aspects of statistics under various frameworks. In particular, there are weighted likelihoods
Likelihood function
In statistics, a likelihood function is a function of the parameters of a statistical model, defined as follows: the likelihood of a set of parameter values given some observed outcomes is equal to the probability of those observed outcomes given those parameter values...
, weighted estimating equations
Generalized estimating equations
In statistics, a generalized estimating equation is used to estimate the parameters of a generalized linear model with a possible unknown correlation between outcomes....
, and weighted probability densities
Probability density function
In probability theory, a probability density function , or density of a continuous random variable is a function that describes the relative likelihood for this random variable to occur at a given point. The probability for the random variable to fall within a particular region is given by the...
from which a majority of statistics are derived. These applications codified the theory of other statistics and estimators such as marginal structural models, the standardized mortality ratio
Standardized mortality ratio
The standardized mortality ratio or SMR in epidemiology is the ratio of observed deaths to expected deaths, where expected deaths are calculated for a typical area with the same age and gender mix by looking at the death rates for different ages and genders in the larger population.The SMR may be...
, and the EM algorithm for coarsened or aggregate data.
Inverse probability weighting is also used to account for missing data when subjects with missing data cannot be included in the primary analysis.
With an estimate of the inclusion probability, or the probability that the factor would be measured in another measurement, inverse probability weighting can be used to inflate the weight for subjects who are under-represented due to a large degree of missing data.