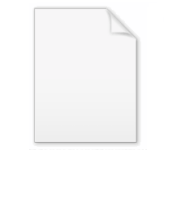
Kolmogorov extension theorem
Encyclopedia
In mathematics
, the Kolmogorov extension theorem (also known as Kolmogorov existence theorem or Kolmogorov consistency theorem) is a theorem
that guarantees that a suitably "consistent" collection of finite-dimensional distribution
s will define a stochastic process
. It is credited to the Soviet mathematician
Andrey Nikolaevich Kolmogorov
.
denote some interval
(thought of as "time
"), and let
. For each
and finite sequence
of times
, let
be a probability measure
on
. Suppose that these measures satisfy two consistency conditions:
1. for all permutation
s
of
and measurable sets
,
2. for all measurable sets
,

Then there exists a probability space
and a stochastic process
such that
for all
,
and measurable sets
, i.e.
has
as its finite-dimensional distributions relative to times
.
In fact, it is always possible to take as the underlying probability space
and to take for
the canonical process
. Therefore, an alternative way of stating Kolomogorov's extension theorem is that, provided that the above consistency conditions hold, there exists a (unique) measure
on
with marginals
for any finite collection of times
. The remarkable feature of Kolmogorov's extension theorem is that it does not require
to be countable, but the price to pay
for this level of generality is that the measure
is only defined on the product σ-algebra of
, which is not very rich.
. Then the probability
can be computed either as
or as
. Hence, for the finite-dimensional distributions to be consistent, it must hold that
.
The first condition generalises this obvious statement to hold for any number of time points
, and any control sets
.
Continuing the example, the second condition implies that
. Also this is a trivial statement that must be satisfied for any consistent family of finite-dimensional distributions.
The measure-theoretic approach to stochastic processes starts with a probability space and defines a stochastic process as a family of functions on this probability space. However, in many applications the starting point is really the finite-dimensional distributions ("statistics") of the stochastic process. The theorem says that provided the finite-dimensional distributions satisfy the obvious consistency requirements, one can always identify a probability space to match the purpose. In many situations, this means that one does not have to be explicit about what the probability space is. Many texts on stochastic processes do, indeed, assume a probability space but never state explicitly what it is.
Mathematics
Mathematics is the study of quantity, space, structure, and change. Mathematicians seek out patterns and formulate new conjectures. Mathematicians resolve the truth or falsity of conjectures by mathematical proofs, which are arguments sufficient to convince other mathematicians of their validity...
, the Kolmogorov extension theorem (also known as Kolmogorov existence theorem or Kolmogorov consistency theorem) is a theorem
Theorem
In mathematics, a theorem is a statement that has been proven on the basis of previously established statements, such as other theorems, and previously accepted statements, such as axioms...
that guarantees that a suitably "consistent" collection of finite-dimensional distribution
Finite-dimensional distribution
In mathematics, finite-dimensional distributions are a tool in the study of measures and stochastic processes. A lot of information can be gained by studying the "projection" of a measure onto a finite-dimensional vector space .-Finite-dimensional distributions of a measure:Let be a measure space...
s will define a stochastic process
Stochastic process
In probability theory, a stochastic process , or sometimes random process, is the counterpart to a deterministic process...
. It is credited to the Soviet mathematician
Mathematician
A mathematician is a person whose primary area of study is the field of mathematics. Mathematicians are concerned with quantity, structure, space, and change....
Andrey Nikolaevich Kolmogorov
Andrey Kolmogorov
Andrey Nikolaevich Kolmogorov was a Soviet mathematician, preeminent in the 20th century, who advanced various scientific fields, among them probability theory, topology, intuitionistic logic, turbulence, classical mechanics and computational complexity.-Early life:Kolmogorov was born at Tambov...
.
Statement of the theorem
Let
Interval (mathematics)
In mathematics, a interval is a set of real numbers with the property that any number that lies between two numbers in the set is also included in the set. For example, the set of all numbers satisfying is an interval which contains and , as well as all numbers between them...
(thought of as "time
Time
Time is a part of the measuring system used to sequence events, to compare the durations of events and the intervals between them, and to quantify rates of change such as the motions of objects....
"), and let


Sequence
In mathematics, a sequence is an ordered list of objects . Like a set, it contains members , and the number of terms is called the length of the sequence. Unlike a set, order matters, and exactly the same elements can appear multiple times at different positions in the sequence...
of times


Probability measure
In mathematics, a probability measure is a real-valued function defined on a set of events in a probability space that satisfies measure properties such as countable additivity...
on

1. for all permutation
Permutation
In mathematics, the notion of permutation is used with several slightly different meanings, all related to the act of permuting objects or values. Informally, a permutation of a set of objects is an arrangement of those objects into a particular order...
s




2. for all measurable sets



Then there exists a probability space
Probability space
In probability theory, a probability space or a probability triple is a mathematical construct that models a real-world process consisting of states that occur randomly. A probability space is constructed with a specific kind of situation or experiment in mind...



for all






In fact, it is always possible to take as the underlying probability space








for this level of generality is that the measure


Explanation of the conditions
The two conditions required by the theorem are trivially satisfied by any stochastic process. For example, consider a real-valued discrete-time stochastic process




The first condition generalises this obvious statement to hold for any number of time points


Continuing the example, the second condition implies that

Implications of the theorem
Since the two conditions are trivially satisfied for any stochastic process, the powerful statement of the theorem is that no other conditions are required: For any reasonable (i.e., consistent) family of finite-dimensional distributions, there exists a stochastic process with these distributions.The measure-theoretic approach to stochastic processes starts with a probability space and defines a stochastic process as a family of functions on this probability space. However, in many applications the starting point is really the finite-dimensional distributions ("statistics") of the stochastic process. The theorem says that provided the finite-dimensional distributions satisfy the obvious consistency requirements, one can always identify a probability space to match the purpose. In many situations, this means that one does not have to be explicit about what the probability space is. Many texts on stochastic processes do, indeed, assume a probability space but never state explicitly what it is.