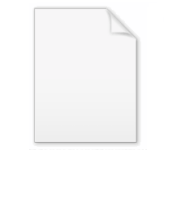
Law of total expectation
Encyclopedia
The proposition in probability theory
known as the law of total expectation, the law of iterated expectations, the tower rule, the smoothing theorem, among other names, states that if X is an integrable random variable
(i.e., a random variable satisfying E( | X | ) < ∞) and Y is any random variable, not necessarily integrable, on the same probability space
, then

i.e., the expected value
of the conditional expected value of X given Y is the same as the expected value of X.
The nomenclature used here parallels the phrase law of total probability
. See also law of total variance
.
(The conditional expected value E( X | Y ) is a random variable in its own right, whose value depends on the value of Y. Notice that the conditional expected value of X given the event Y = y is a function of y (this is where adherence to the conventional rigidly case-sensitive notation of probability theory
becomes important!). If we write E( X | Y = y) = g(y) then the random variable E( X | Y ) is just g(Y).
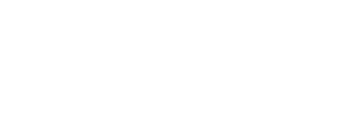

where the value of I1 is determined by that of I2. To build intuition, imagine an investor who forecasts a random stock price X based on the limited information set I1. The law of iterated expectations says that the investor can never gain a more precise forecast of X by conditioning on more specific information (I2), if the more specific forecast must itself be forecast with the original information (I1).
This formulation is often applied in a time series
context, where Et denotes expectations conditional on only the information observed up to and including time period t. In typical models the information set t + 1 contains all information available through time t, plus additional information revealed at time t + 1. One can then write:
Probability theory
Probability theory is the branch of mathematics concerned with analysis of random phenomena. The central objects of probability theory are random variables, stochastic processes, and events: mathematical abstractions of non-deterministic events or measured quantities that may either be single...
known as the law of total expectation, the law of iterated expectations, the tower rule, the smoothing theorem, among other names, states that if X is an integrable random variable
Random variable
In probability and statistics, a random variable or stochastic variable is, roughly speaking, a variable whose value results from a measurement on some type of random process. Formally, it is a function from a probability space, typically to the real numbers, which is measurable functionmeasurable...
(i.e., a random variable satisfying E( | X | ) < ∞) and Y is any random variable, not necessarily integrable, on the same probability space
Probability space
In probability theory, a probability space or a probability triple is a mathematical construct that models a real-world process consisting of states that occur randomly. A probability space is constructed with a specific kind of situation or experiment in mind...
, then

i.e., the expected value
Expected value
In probability theory, the expected value of a random variable is the weighted average of all possible values that this random variable can take on...
of the conditional expected value of X given Y is the same as the expected value of X.
The nomenclature used here parallels the phrase law of total probability
Law of total probability
In probability theory, the law of total probability is a fundamental rule relating marginal probabilities to conditional probabilities.-Statement:The law of total probability is the proposition that if \left\...
. See also law of total variance
Law of total variance
In probability theory, the law of total variance or variance decomposition formula states that if X and Y are random variables on the same probability space, and the variance of Y is finite, then...
.
(The conditional expected value E( X | Y ) is a random variable in its own right, whose value depends on the value of Y. Notice that the conditional expected value of X given the event Y = y is a function of y (this is where adherence to the conventional rigidly case-sensitive notation of probability theory
Notation in probability
Probability theory and statistics has some commonly used conventions of its own, in addition to standard mathematical notation and mathematical symbols.-Probability theory:* Random variables are usually written in upper case roman letters: X, Y, etc....
becomes important!). If we write E( X | Y = y) = g(y) then the random variable E( X | Y ) is just g(Y).
Proof in the discrete case
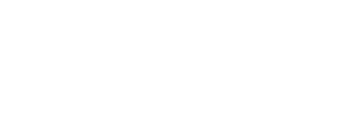
Iterated expectations with nested conditioning sets
The following formulation of the law of iterated expectations plays an important role in many economic and finance models:
where the value of I1 is determined by that of I2. To build intuition, imagine an investor who forecasts a random stock price X based on the limited information set I1. The law of iterated expectations says that the investor can never gain a more precise forecast of X by conditioning on more specific information (I2), if the more specific forecast must itself be forecast with the original information (I1).
This formulation is often applied in a time series
Time series
In statistics, signal processing, econometrics and mathematical finance, a time series is a sequence of data points, measured typically at successive times spaced at uniform time intervals. Examples of time series are the daily closing value of the Dow Jones index or the annual flow volume of the...
context, where Et denotes expectations conditional on only the information observed up to and including time period t. In typical models the information set t + 1 contains all information available through time t, plus additional information revealed at time t + 1. One can then write:
