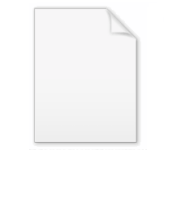
Lilliefors test
Encyclopedia
In statistics
, the Lilliefors test, named after Hubert Lilliefors
, professor of statistics at George Washington University
, is an adaptation of the Kolmogorov–Smirnov test. It is used to test the null hypothesis
that data come from a normally distributed population, when the null hypothesis does not specify which normal distribution; i.e., it does not specify the expected value
and variance
of the distribution
1. First estimate the population mean and population variance based on the data.
2. Then find the maximum discrepancy between the empirical distribution function
and the cumulative distribution function
(CDF) of the normal distribution with the estimated mean and estimated variance. Just as in the Kolmogorov–Smirnov test, this will be the test statistic.
3. Finally, assess whether the maximum discrepancy is large enough to be statistically significant
, thus requiring rejection of the null hypothesis. This is where this test becomes more complicated than the Kolmogorov–Smirnov test. Since the hypothesized CDF has been moved closer to the data by estimation based on those data, the maximum discrepancy has been made smaller than it would have been if the null hypothesis had singled out just one normal distribution. Thus the "null distribution" of the test statistic, i.e. its probability distribution
assuming the null hypothesis is true, is stochastically smaller than the Kolmogorov–Smirnov distribution. This is the Lilliefors distribution. To date, tables for this distribution have been computed only by Monte Carlo method
s.
There is an extensive literature on normality test
ing, but as a practical matter many experienced data analysts sidestep formal testing and assess the feasibility of a normal model by using a graphical tool such as a Q-Q plot
.
Statistics
Statistics is the study of the collection, organization, analysis, and interpretation of data. It deals with all aspects of this, including the planning of data collection in terms of the design of surveys and experiments....
, the Lilliefors test, named after Hubert Lilliefors
Hubert Lilliefors
Hubert Whitman Lilliefors was an American statistician, noted for his introduction of the Lilliefors test...
, professor of statistics at George Washington University
George Washington University
The George Washington University is a private, coeducational comprehensive university located in Washington, D.C. in the United States...
, is an adaptation of the Kolmogorov–Smirnov test. It is used to test the null hypothesis
Null hypothesis
The practice of science involves formulating and testing hypotheses, assertions that are capable of being proven false using a test of observed data. The null hypothesis typically corresponds to a general or default position...
that data come from a normally distributed population, when the null hypothesis does not specify which normal distribution; i.e., it does not specify the expected value
Expected value
In probability theory, the expected value of a random variable is the weighted average of all possible values that this random variable can take on...
and variance
Variance
In probability theory and statistics, the variance is a measure of how far a set of numbers is spread out. It is one of several descriptors of a probability distribution, describing how far the numbers lie from the mean . In particular, the variance is one of the moments of a distribution...
of the distribution
The test
The test proceeds as follows:1. First estimate the population mean and population variance based on the data.
2. Then find the maximum discrepancy between the empirical distribution function
Empirical distribution function
In statistics, the empirical distribution function, or empirical cdf, is the cumulative distribution function associated with the empirical measure of the sample. This cdf is a step function that jumps up by 1/n at each of the n data points. The empirical distribution function estimates the true...
and the cumulative distribution function
Cumulative distribution function
In probability theory and statistics, the cumulative distribution function , or just distribution function, describes the probability that a real-valued random variable X with a given probability distribution will be found at a value less than or equal to x. Intuitively, it is the "area so far"...
(CDF) of the normal distribution with the estimated mean and estimated variance. Just as in the Kolmogorov–Smirnov test, this will be the test statistic.
3. Finally, assess whether the maximum discrepancy is large enough to be statistically significant
Statistical significance
In statistics, a result is called statistically significant if it is unlikely to have occurred by chance. The phrase test of significance was coined by Ronald Fisher....
, thus requiring rejection of the null hypothesis. This is where this test becomes more complicated than the Kolmogorov–Smirnov test. Since the hypothesized CDF has been moved closer to the data by estimation based on those data, the maximum discrepancy has been made smaller than it would have been if the null hypothesis had singled out just one normal distribution. Thus the "null distribution" of the test statistic, i.e. its probability distribution
Probability distribution
In probability theory, a probability mass, probability density, or probability distribution is a function that describes the probability of a random variable taking certain values....
assuming the null hypothesis is true, is stochastically smaller than the Kolmogorov–Smirnov distribution. This is the Lilliefors distribution. To date, tables for this distribution have been computed only by Monte Carlo method
Monte Carlo method
Monte Carlo methods are a class of computational algorithms that rely on repeated random sampling to compute their results. Monte Carlo methods are often used in computer simulations of physical and mathematical systems...
s.
There is an extensive literature on normality test
Normality test
In statistics, normality tests are used to determine whether a data set is well-modeled by a normal distribution or not, or to compute how likely an underlying random variable is to be normally distributed....
ing, but as a practical matter many experienced data analysts sidestep formal testing and assess the feasibility of a normal model by using a graphical tool such as a Q-Q plot
Q-Q plot
In statistics, a Q-Q plot is a probability plot, which is a graphical method for comparing two probability distributions by plotting their quantiles against each other. First, the set of intervals for the quantiles are chosen...
.