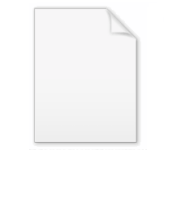
Multiple discriminant analysis
Encyclopedia
Multiple Discriminant Analysis (MDA) is a method for compressing a multivariate signal to yield a lower dimensional signal amenable to classification.
MDA is not directly used to perform classification. It merely supports classification by yielding a compressed signal amenable to classification. The method described in Duda et al. (2001) §3.8.3 projects the multivariate signal down to an M−1 dimensional space where M is the number of categories.
MDA is useful because most classifiers are strongly affected by the curse of dimensionality
. In other words, when signals are represented in very high dimensional spaces, the classifier's performance is catastrophically impaired by the over-fitting problem. This problem is reduced by compressing the signal down to a lower-dimensional space as MDA does.
MDA has been used to reveal neural codes.
MDA is not directly used to perform classification. It merely supports classification by yielding a compressed signal amenable to classification. The method described in Duda et al. (2001) §3.8.3 projects the multivariate signal down to an M−1 dimensional space where M is the number of categories.
MDA is useful because most classifiers are strongly affected by the curse of dimensionality
Curse of dimensionality
The curse of dimensionality refers to various phenomena that arise when analyzing and organizing high-dimensional spaces that do not occur in low-dimensional settings such as the physical space commonly modeled with just three dimensions.There are multiple phenomena referred to by this name in...
. In other words, when signals are represented in very high dimensional spaces, the classifier's performance is catastrophically impaired by the over-fitting problem. This problem is reduced by compressing the signal down to a lower-dimensional space as MDA does.
MDA has been used to reveal neural codes.