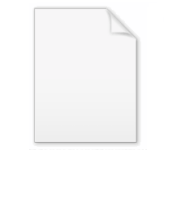
Mutually exclusive
Encyclopedia
In layman
's terms, two events are mutually exclusive if they cannot occur at the same time. An example is tossing a coin once, which can result in either heads or tails, but not both.
In the coin-tossing example, both outcomes are collectively exhaustive, which means that at least one of the outcomes must happen, so these two possibilities together exhaust all the possibilities. However, not all mutually exclusive events are collectively exhaustive. For example, the outcomes 1 and 4 of a single roll of a six-sided die are mutually exclusive (cannot both happen) but not collectively exhaustive (there are other possible outcomes).
, two mutually exclusive propositions are propositions that logically cannot
be true at the same time. Another term for mutually exclusive is "disjoint". To say that more than two propositions are mutually exclusive, depending on context, means that one cannot be true if the other one is true, or at least one of them cannot be true. The term pairwise mutually exclusive always means two of them cannot be true simultaneously.
, events E1, E2, ..., En are said to be mutually exclusive if the occurrence of any one of them automatically implies the non-occurrence of the remaining n − 1 events. Therefore, two mutually exclusive events cannot both occur. Mutually exclusive events have the property: P(A and B) = 0. For example, one cannot draw a card that is both red and a club because clubs are always black. If one draws just one card from the deck, either a red card or a club can be drawn. When A and B are mutually exclusive, P(A or B) = P(A) + P(B). One might ask, "What is the probability of drawing a red card or a club?" This problem would be solved by adding together the probability of drawing a red card and the probability of drawing a club. In a standard 52-card deck, there are twenty-six red cards and thirteen clubs: 26/52 + 13/52 = 39/52 or 3/4.
One would have to draw at least two cards in order to draw both a red card and a club. The probability of doing so in two draws would depend on whether the first card drawn were replaced before the second drawing, since without replacement there would be one fewer card after the first card was drawn. The probabilities of the individual events (red, and club) would be multiplied rather than added. The probability of drawing a red and a club in two drawings without replacement would be 26/52 * 13/51 = 338/2652, or 13/102. With replacement, the probability would be 26/52 * 13/52 = 338/2704, or 13/104.
In probability theory the word "or" allows for the possibility of both events happening. The probability of one or both events occurring is denoted P(A or B) and in general it equals P(A) + P(B) – P(A and B). Therefore, if one asks, "What is the probability of drawing a red card or a king?", drawing any of a red king, a red non-king, or a black king is considered a success. In a standard 52-card deck, there are twenty-six red cards and four kings, two of which are red, so the probability of drawing a red or a king is 26/52 + 4/52 – 2/52 = 28/52. However, with mutually exclusive events the last term in the formula, – P(A and B), is zero, so the formula simplifies to the one given in the previous paragraph.
Events are collectively exhaustive
if all the possibilities for outcomes are exhausted by those possible events, so at least one of those outcomes must occur. The probability that at least one of the events will occur is equal to 1. For example, there are theoretically only two possibilities for flipping a coin. Flipping a head and flipping a tail are collectively exhaustive events, and there is a probability of 1 of flipping either a head or a tail.
Events can be both mutually exclusive and collectively exhaustive. In the case of flipping a coin, flipping a head and flipping a tail are also mutually exclusive events. Both outcomes cannot occur for a single trial (i.e., when a coin is flipped only once). The probability of flipping a head and the probability of flipping a tail can be added to yield a probability of 1: 1/2 + 1/2 =1.
and regression analysis
, an independent variable
that can take on only two possible values is called a dummy variable
. For example, it may take on the value 0 if an observation is of a male subject or 1 if the observation is of a female subject. The two possible categories associated with the two possible values are mutually exclusive, so that no observation falls into more than one category, and the categories are exhaustive, so that every observation falls into some category. Sometimes there are three or more possible categories, which are pairwise mutually exclusive and are collectively exhaustive — for example, under 18 years of age, 18 to 64 years of age, and age 65 or above. In this case a set of dummy variables is constructed, each dummy variable having two mutually exclusive and jointly exhaustive categories — in this example, one dummy variable (called D1) would equal 1 if age is less than 18, and would equal 0 otherwise; a second dummy variable (called D2) would equal 1 if age is in the range 18-64, and 0 otherwise. In this set-up, the dummy variable pairs (D1, D2) can have the values (1,0) (under 18), (0,1) (between 18 and 64), or (0,0) (65 or older) (but not (1,1), which would nonsensically imply that an observed subject is both under 18 and between 18 and 64). Then the dummy variables can be included as independent (explanatory) variables in a regression. Note that the number of dummy variables is always one less than the number of categories: with the two categories male and female there is a single dummy variable to distinguish them, while with the three age categories two dummy variables are needed to distinguish them.
Such qualitative data can also be used for dependent variables. For example, a researcher might want to predict whether someone goes to college or not, using family income, a gender dummy variable, and so forth as explanatory variables. Here the variable to be explained is a dummy variable that equals 0 if the observed subject does not go to college and equals 1 if the subject does go to college. In such a situation, ordinary least squares
(the basic regression technique) is widely seen as inadequate; instead probit regression or logistic regression
is used. Further, sometimes there are three or more categories for the dependent variable — for example, no college, community college, and four-year college. In this case, the multinomial probit
or multinomial logit
technique is used.
Layman
A layperson or layman is a person who is not an expert in a given field of knowledge. The term originally meant a member of the laity, i.e. a non-clergymen, but over the centuries shifted in definition....
's terms, two events are mutually exclusive if they cannot occur at the same time. An example is tossing a coin once, which can result in either heads or tails, but not both.
In the coin-tossing example, both outcomes are collectively exhaustive, which means that at least one of the outcomes must happen, so these two possibilities together exhaust all the possibilities. However, not all mutually exclusive events are collectively exhaustive. For example, the outcomes 1 and 4 of a single roll of a six-sided die are mutually exclusive (cannot both happen) but not collectively exhaustive (there are other possible outcomes).
Logic
In logicLogic
In philosophy, Logic is the formal systematic study of the principles of valid inference and correct reasoning. Logic is used in most intellectual activities, but is studied primarily in the disciplines of philosophy, mathematics, semantics, and computer science...
, two mutually exclusive propositions are propositions that logically cannot
Logical possibility
A logically possible proposition is one that can be asserted without implying a logical contradiction. This is to say that a proposition is logically possible if there is some coherent way for the world to be, under which the proposition would be true...
be true at the same time. Another term for mutually exclusive is "disjoint". To say that more than two propositions are mutually exclusive, depending on context, means that one cannot be true if the other one is true, or at least one of them cannot be true. The term pairwise mutually exclusive always means two of them cannot be true simultaneously.
Probability
In probability theoryProbability theory
Probability theory is the branch of mathematics concerned with analysis of random phenomena. The central objects of probability theory are random variables, stochastic processes, and events: mathematical abstractions of non-deterministic events or measured quantities that may either be single...
, events E1, E2, ..., En are said to be mutually exclusive if the occurrence of any one of them automatically implies the non-occurrence of the remaining n − 1 events. Therefore, two mutually exclusive events cannot both occur. Mutually exclusive events have the property: P(A and B) = 0. For example, one cannot draw a card that is both red and a club because clubs are always black. If one draws just one card from the deck, either a red card or a club can be drawn. When A and B are mutually exclusive, P(A or B) = P(A) + P(B). One might ask, "What is the probability of drawing a red card or a club?" This problem would be solved by adding together the probability of drawing a red card and the probability of drawing a club. In a standard 52-card deck, there are twenty-six red cards and thirteen clubs: 26/52 + 13/52 = 39/52 or 3/4.
One would have to draw at least two cards in order to draw both a red card and a club. The probability of doing so in two draws would depend on whether the first card drawn were replaced before the second drawing, since without replacement there would be one fewer card after the first card was drawn. The probabilities of the individual events (red, and club) would be multiplied rather than added. The probability of drawing a red and a club in two drawings without replacement would be 26/52 * 13/51 = 338/2652, or 13/102. With replacement, the probability would be 26/52 * 13/52 = 338/2704, or 13/104.
In probability theory the word "or" allows for the possibility of both events happening. The probability of one or both events occurring is denoted P(A or B) and in general it equals P(A) + P(B) – P(A and B). Therefore, if one asks, "What is the probability of drawing a red card or a king?", drawing any of a red king, a red non-king, or a black king is considered a success. In a standard 52-card deck, there are twenty-six red cards and four kings, two of which are red, so the probability of drawing a red or a king is 26/52 + 4/52 – 2/52 = 28/52. However, with mutually exclusive events the last term in the formula, – P(A and B), is zero, so the formula simplifies to the one given in the previous paragraph.
Events are collectively exhaustive
Collectively exhaustive
In probability theory, a set of events is jointly or collectively exhaustive if at least one of the events must occur. For example, when rolling a six-sided die, the outcomes 1, 2, 3, 4, 5, and 6 are collectively exhaustive, because they encompass the entire range of possible outcomes.Another way...
if all the possibilities for outcomes are exhausted by those possible events, so at least one of those outcomes must occur. The probability that at least one of the events will occur is equal to 1. For example, there are theoretically only two possibilities for flipping a coin. Flipping a head and flipping a tail are collectively exhaustive events, and there is a probability of 1 of flipping either a head or a tail.
Events can be both mutually exclusive and collectively exhaustive. In the case of flipping a coin, flipping a head and flipping a tail are also mutually exclusive events. Both outcomes cannot occur for a single trial (i.e., when a coin is flipped only once). The probability of flipping a head and the probability of flipping a tail can be added to yield a probability of 1: 1/2 + 1/2 =1.
Statistics
In statisticsStatistics
Statistics is the study of the collection, organization, analysis, and interpretation of data. It deals with all aspects of this, including the planning of data collection in terms of the design of surveys and experiments....
and regression analysis
Regression analysis
In statistics, regression analysis includes many techniques for modeling and analyzing several variables, when the focus is on the relationship between a dependent variable and one or more independent variables...
, an independent variable
Dependent and independent variables
The terms "dependent variable" and "independent variable" are used in similar but subtly different ways in mathematics and statistics as part of the standard terminology in those subjects...
that can take on only two possible values is called a dummy variable
Dummy variable
In statistics and econometrics, particularly in regression analysis, a dummy variable is one that takes the values 0 or 1 to indicate the absence or presence of some categorical effect that may be expected to shift the outcome...
. For example, it may take on the value 0 if an observation is of a male subject or 1 if the observation is of a female subject. The two possible categories associated with the two possible values are mutually exclusive, so that no observation falls into more than one category, and the categories are exhaustive, so that every observation falls into some category. Sometimes there are three or more possible categories, which are pairwise mutually exclusive and are collectively exhaustive — for example, under 18 years of age, 18 to 64 years of age, and age 65 or above. In this case a set of dummy variables is constructed, each dummy variable having two mutually exclusive and jointly exhaustive categories — in this example, one dummy variable (called D1) would equal 1 if age is less than 18, and would equal 0 otherwise; a second dummy variable (called D2) would equal 1 if age is in the range 18-64, and 0 otherwise. In this set-up, the dummy variable pairs (D1, D2) can have the values (1,0) (under 18), (0,1) (between 18 and 64), or (0,0) (65 or older) (but not (1,1), which would nonsensically imply that an observed subject is both under 18 and between 18 and 64). Then the dummy variables can be included as independent (explanatory) variables in a regression. Note that the number of dummy variables is always one less than the number of categories: with the two categories male and female there is a single dummy variable to distinguish them, while with the three age categories two dummy variables are needed to distinguish them.
Such qualitative data can also be used for dependent variables. For example, a researcher might want to predict whether someone goes to college or not, using family income, a gender dummy variable, and so forth as explanatory variables. Here the variable to be explained is a dummy variable that equals 0 if the observed subject does not go to college and equals 1 if the subject does go to college. In such a situation, ordinary least squares
Ordinary least squares
In statistics, ordinary least squares or linear least squares is a method for estimating the unknown parameters in a linear regression model. This method minimizes the sum of squared vertical distances between the observed responses in the dataset and the responses predicted by the linear...
(the basic regression technique) is widely seen as inadequate; instead probit regression or logistic regression
Logistic regression
In statistics, logistic regression is used for prediction of the probability of occurrence of an event by fitting data to a logit function logistic curve. It is a generalized linear model used for binomial regression...
is used. Further, sometimes there are three or more categories for the dependent variable — for example, no college, community college, and four-year college. In this case, the multinomial probit
Multinomial probit
In econometrics and statistics, the multinomial probit model, a popular alternative to the multinomial logit model, is a generalization of the probit model that allows more than two discrete, unordered outcomes. It is not to be confused with the multivariate probit model, which is used to model...
or multinomial logit
Multinomial logit
In statistics, economics, and genetics, a multinomial logit model, also known as multinomial logistic regression, is a regression model which generalizes logistic regression by allowing more than two discrete outcomes...
technique is used.