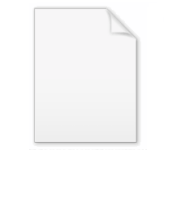
Structured data mining
Encyclopedia
Structure mining or structured data mining is the process of finding and extracting useful information from semi structured data sets. Graph mining is a special case of structured data mining.
has created new opportunities for data mining, which has traditionally been concerned with tabular data sets, reflecting the strong association between data mining
and relational databases. Much of the world's interesting and mineable data does not easily fold into relational databases, though a generation of software engineers have been trained to believe this was the only way to handle data, and data mining algorithms have generally been developed only to cope with tabular data.
XML
, being the most frequent way of representing semi-structured data, is able to represent both tabular data and arbitrary trees. Any particular representation of data to be exchanged between two applications in XML is normally described by a Schema often written in XSD. Practical examples of such Schemata, for instance NewsML, are normally very sophisticated, containing multiple optional subtrees, used for representing special case data. Frequently around 90% of a Schema is concerned with the definition of these optional data items and sub-trees.
Messages and data, therefore, that are transmitted or encoded using XML and that conform to the same Schema are liable to contain very different data depending on what is being transmitted.
Such data presents large problems for conventional data mining. Two messages that conform to the same Schema may have little data in common. Building a training set from such data means that if one were to try to format it as tabular data for conventional data mining, large sections of the tables would or could be empty.
There is a tacit assumption made in the design of most data mining algorithms that the data presented will be complete. Many algorithms perform badly with incomplete data sets, for instance those based on neural networks
.
XPath
is the standard mechanism used to refer to nodes and data items within XML. It has similarities to standard techniques for navigating directory hierarchies used in operating systems user interfaces. To data and structure mine XML data of any form, at least two extensions are required to conventional data mining. These are the ability to associate an XPath statement with any data pattern and sub statements with each data node in the data pattern, and the ability to mine the presence and count of any node or set of nodes within the document.
As an example, if one were to represent a family tree in XML, using these extensions one could create a data set containing all the individuals in the tree, data items such as name and age at death, and counts of related nodes, such as number of children. More sophisticated searches could extract data such as grandparents' lifespans etc.
The addition of these data types related to the structure of a document or message facilitates structure mining.
The other desideratum is that the actual mining algorithms employed, whether supervised or unsupervised, must be able to handle sparse data. In practice the set of data mining algorithms that are best at handling sparse data are those that process the training set data into trees of related patterns. These are frequently descendants of or take their inspiration from Ross Quinlan
's ID3 algorithm
.
Description
The growth of the use of semi-structured dataSemi-structured data
Semi-structured data is a form of structured data that does not conform with the formal structure of tables and data models associated with relational databases but nonetheless contains tags or other markers to separate semantic elements and enforce hierarchies of records and fields within the data...
has created new opportunities for data mining, which has traditionally been concerned with tabular data sets, reflecting the strong association between data mining
Data mining
Data mining , a relatively young and interdisciplinary field of computer science is the process of discovering new patterns from large data sets involving methods at the intersection of artificial intelligence, machine learning, statistics and database systems...
and relational databases. Much of the world's interesting and mineable data does not easily fold into relational databases, though a generation of software engineers have been trained to believe this was the only way to handle data, and data mining algorithms have generally been developed only to cope with tabular data.
XML
XML
Extensible Markup Language is a set of rules for encoding documents in machine-readable form. It is defined in the XML 1.0 Specification produced by the W3C, and several other related specifications, all gratis open standards....
, being the most frequent way of representing semi-structured data, is able to represent both tabular data and arbitrary trees. Any particular representation of data to be exchanged between two applications in XML is normally described by a Schema often written in XSD. Practical examples of such Schemata, for instance NewsML, are normally very sophisticated, containing multiple optional subtrees, used for representing special case data. Frequently around 90% of a Schema is concerned with the definition of these optional data items and sub-trees.
Messages and data, therefore, that are transmitted or encoded using XML and that conform to the same Schema are liable to contain very different data depending on what is being transmitted.
Such data presents large problems for conventional data mining. Two messages that conform to the same Schema may have little data in common. Building a training set from such data means that if one were to try to format it as tabular data for conventional data mining, large sections of the tables would or could be empty.
There is a tacit assumption made in the design of most data mining algorithms that the data presented will be complete. Many algorithms perform badly with incomplete data sets, for instance those based on neural networks
Artificial neural network
An artificial neural network , usually called neural network , is a mathematical model or computational model that is inspired by the structure and/or functional aspects of biological neural networks. A neural network consists of an interconnected group of artificial neurons, and it processes...
.
XPath
XPath
XPath is a language for selecting nodes from an XML document. In addition, XPath may be used to compute values from the content of an XML document...
is the standard mechanism used to refer to nodes and data items within XML. It has similarities to standard techniques for navigating directory hierarchies used in operating systems user interfaces. To data and structure mine XML data of any form, at least two extensions are required to conventional data mining. These are the ability to associate an XPath statement with any data pattern and sub statements with each data node in the data pattern, and the ability to mine the presence and count of any node or set of nodes within the document.
As an example, if one were to represent a family tree in XML, using these extensions one could create a data set containing all the individuals in the tree, data items such as name and age at death, and counts of related nodes, such as number of children. More sophisticated searches could extract data such as grandparents' lifespans etc.
The addition of these data types related to the structure of a document or message facilitates structure mining.
The other desideratum is that the actual mining algorithms employed, whether supervised or unsupervised, must be able to handle sparse data. In practice the set of data mining algorithms that are best at handling sparse data are those that process the training set data into trees of related patterns. These are frequently descendants of or take their inspiration from Ross Quinlan
Ross Quinlan
John Ross Quinlan is a computer science researcher in data mining and decision theory. He has contributed extensively to the development of decision tree algorithms, including inventing the canonical C4.5 and ID3 algorithms...
's ID3 algorithm
ID3 algorithm
In decision tree learning, ID3 is an algorithm used to generate a decision tree invented by Ross Quinlan. ID3 is the precursor to the C4.5 algorithm.-Algorithm:The ID3 algorithm can be summarized as follows:...
.
See also
- Molecule miningMolecule miningThis page describes mining for molecules. Since molecules may be represented by molecular graphs this is strongly related to graph mining and structured data mining. The main problem is how to represent molecules while discriminating the data instances...
- Sequence miningSequence miningSequence mining is concerned with finding statistically relevant patterns between data examples where the values are delivered in a sequence. It is usually presumed that the values are discrete, and thus Time series mining is closely related, but usually considered a different activity...
- Data miningData miningData mining , a relatively young and interdisciplinary field of computer science is the process of discovering new patterns from large data sets involving methods at the intersection of artificial intelligence, machine learning, statistics and database systems...
- Data warehousing
- Structured contentStructured contentStructured content refers to information or content that has been broken down and classified using metadata. Structured content often refers to information that has been classified using XML, but can also relate to information classified using other standard or proprietary forms of metadata....