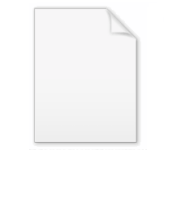
Structured prediction
Encyclopedia
Structured prediction is an umbrella term for machine learning
and regression
techniques that involve predicting structured objects. For example, the problem of translating a natural language sentence into a semantic representation such as a parse tree can be seen as a structured prediction problem in which the structured output domain is the set of all possible parse trees. Structured prediction generalizes supervised learning where the output domain is usually a small or simple set.
Probabilistic graphical models form a large class of structured prediction models. In particular, Bayesian network
s and random fields are popularly used to solve structured prediction problems in a wide variety of application domains including bioinformatics, natural language processing, speech recognition, and computer vision.
Similar to commonly used supervised learning techniques, structured prediction models are typically trained by means of observed data in which the true prediction value is used to adjust model parameters. Due to the complexity of the model and the interrelations of predicted variables the process of prediction using a trained model and of training itself is often computationally infeasible and approximate inference and learning methods are used.
Another commonly used term for structured prediction is structured output learning.
Machine learning
Machine learning, a branch of artificial intelligence, is a scientific discipline concerned with the design and development of algorithms that allow computers to evolve behaviors based on empirical data, such as from sensor data or databases...
and regression
Regression analysis
In statistics, regression analysis includes many techniques for modeling and analyzing several variables, when the focus is on the relationship between a dependent variable and one or more independent variables...
techniques that involve predicting structured objects. For example, the problem of translating a natural language sentence into a semantic representation such as a parse tree can be seen as a structured prediction problem in which the structured output domain is the set of all possible parse trees. Structured prediction generalizes supervised learning where the output domain is usually a small or simple set.
Probabilistic graphical models form a large class of structured prediction models. In particular, Bayesian network
Bayesian network
A Bayesian network, Bayes network, belief network or directed acyclic graphical model is a probabilistic graphical model that represents a set of random variables and their conditional dependencies via a directed acyclic graph . For example, a Bayesian network could represent the probabilistic...
s and random fields are popularly used to solve structured prediction problems in a wide variety of application domains including bioinformatics, natural language processing, speech recognition, and computer vision.
Similar to commonly used supervised learning techniques, structured prediction models are typically trained by means of observed data in which the true prediction value is used to adjust model parameters. Due to the complexity of the model and the interrelations of predicted variables the process of prediction using a trained model and of training itself is often computationally infeasible and approximate inference and learning methods are used.
Another commonly used term for structured prediction is structured output learning.