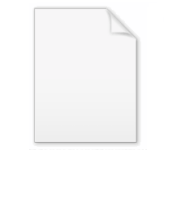
Bayesian brain
Encyclopedia
Bayesian brain is a term that is used to refer to the ability of the nervous system to operate in situations of uncertainty in a fashion that is close to the optimal prescribed by Bayesian statistics. This term is used in behavioural sciences
and neuroscience
and studies associated with this term often strive to explain the brain
's cognitive abilities based on statistical principles. It is frequently assumed that the nervous system maintains internal probabilistic models that are updated by neural processing
of sensory information using methods approximating those of Bayesian probability
.
This field of study has its historical roots in numerous disciplines including machine learning
, experimental psychology
and Bayesian statistics
. As early as the 1860s, with the work of Hermann Helmholtz in experimental psychology the brain's ability to extract perceptual information from sensory data was modeled in terms of probabilistic estimation. The basic idea is that the nervous system needs to organize sensory data into an accurate internal model
of the outside world.
This idea was taken up in research on Unsupervised learning
, in particular the Analysis by Synthesis approach, branches of Machine Learning
.
In 1983 Geoffrey Hinton
and colleagues proposed the brain could be seen as a machine making decisions based on the uncertainties of the outside world. During the 1990s researchers including Peter Dayan
, Geoffrey Hinton and Richard Zemel proposed that the brain represents knowledge of the world in terms of probabilities and made specific proposals for tractable neural processes that could manifest such a Helmholtz Machine
.
Bayesian probability, has been developed by a large field with a wide range of important contributors, e.g. Pierre-Simon Laplace
, Thomas Bayes
, Harold Jeffreys
, Richard Cox
and Edwin Jaynes
has developed mathematical techniques and procedures for treating probability as the degree of plausibility which should be assigned to a given supposition or hypothesis based on the available evidence. In 1988 E.T. Jaynes presented a framework for using Bayesian Probability to model mental processes. It was thus realized early on that the Bayesian statistical framework holds the potential to lead to insights into the function of the nervous system.
A wide range of approaches exist that link Bayesian ideas to the function of the brain.
and Karl Friston began examining the concept of 'free energy
' as a calculably tractable measure of the discrepancy between actual features of the world and representations of those features captured by neural network models..
A synthesis has been attempted recently by Karl Friston, in which the Bayesian brain emerges from a general principle of free energy minimisation. In this framework, both action and perception are seen as a consequence of suppressing free-energy, leading to perceptual and active inference and a more embodied (enactive) view of the Bayesian brain. Using Variational Bayes
ian methods, it can be shown how internal model
s of the world are updated by sensory information to minimize free energy or the discrepancy between sensory input and predictions of that input. This can be cast (in neurobiologically plausible terms) as predictive coding or, more generally, Bayesian filtering.
According to Friston:
This area of research was summarized in terms understandable by the layperson in a 2008 article in New Scientist
that offered a unifying theory of brain function. Friston makes the following claims about the explanatory power of the theory:
Psychology
Psychology is the study of the mind and behavior. Its immediate goal is to understand individuals and groups by both establishing general principles and researching specific cases. For many, the ultimate goal of psychology is to benefit society...
and neuroscience
Neuroscience
Neuroscience is the scientific study of the nervous system. Traditionally, neuroscience has been seen as a branch of biology. However, it is currently an interdisciplinary science that collaborates with other fields such as chemistry, computer science, engineering, linguistics, mathematics,...
and studies associated with this term often strive to explain the brain
Human brain
The human brain has the same general structure as the brains of other mammals, but is over three times larger than the brain of a typical mammal with an equivalent body size. Estimates for the number of neurons in the human brain range from 80 to 120 billion...
's cognitive abilities based on statistical principles. It is frequently assumed that the nervous system maintains internal probabilistic models that are updated by neural processing
Neural Computation
Neural Computation is a peer-reviewed academic journal covering aspects of neural computation. Articles highlight problems and techniques in modeling the brain, and in the design and construction of neurally-inspired information processing systems. Neural Computation was founded in 1989 and is...
of sensory information using methods approximating those of Bayesian probability
Bayesian probability
Bayesian probability is one of the different interpretations of the concept of probability and belongs to the category of evidential probabilities. The Bayesian interpretation of probability can be seen as an extension of logic that enables reasoning with propositions, whose truth or falsity is...
.
This field of study has its historical roots in numerous disciplines including machine learning
Machine learning
Machine learning, a branch of artificial intelligence, is a scientific discipline concerned with the design and development of algorithms that allow computers to evolve behaviors based on empirical data, such as from sensor data or databases...
, experimental psychology
Experimental psychology
Experimental psychology is a methodological approach, rather than a subject, and encompasses varied fields within psychology. Experimental psychologists have traditionally conducted research, published articles, and taught classes on neuroscience, developmental psychology, sensation, perception,...
and Bayesian statistics
Bayesian statistics
Bayesian statistics is that subset of the entire field of statistics in which the evidence about the true state of the world is expressed in terms of degrees of belief or, more specifically, Bayesian probabilities...
. As early as the 1860s, with the work of Hermann Helmholtz in experimental psychology the brain's ability to extract perceptual information from sensory data was modeled in terms of probabilistic estimation. The basic idea is that the nervous system needs to organize sensory data into an accurate internal model
Internal model
An internal model is a postulated neural process that simulates the response of the motor system in order to estimate the outcome of a motor command....
of the outside world.
This idea was taken up in research on Unsupervised learning
Unsupervised learning
In machine learning, unsupervised learning refers to the problem of trying to find hidden structure in unlabeled data. Since the examples given to the learner are unlabeled, there is no error or reward signal to evaluate a potential solution...
, in particular the Analysis by Synthesis approach, branches of Machine Learning
Machine learning
Machine learning, a branch of artificial intelligence, is a scientific discipline concerned with the design and development of algorithms that allow computers to evolve behaviors based on empirical data, such as from sensor data or databases...
.
In 1983 Geoffrey Hinton
Geoffrey Hinton
Geoffrey Hinton is a British born informatician most noted for his work on the mathematics and applications of neural networks, and their relationship to information theory.-Career:...
and colleagues proposed the brain could be seen as a machine making decisions based on the uncertainties of the outside world. During the 1990s researchers including Peter Dayan
Peter Dayan
Peter Dayan is the director of the Gatsby Computational Neuroscience Unit at the University College London. He is the a co-author of 'Theoretical Neuroscience', a leading textbook of computational and mathematical modeling of neural systems...
, Geoffrey Hinton and Richard Zemel proposed that the brain represents knowledge of the world in terms of probabilities and made specific proposals for tractable neural processes that could manifest such a Helmholtz Machine
Helmholtz machine
The Helmholtz machine is a name used by Geoff Hinton to describe a class of neural networks which learn the hidden structure of a set of data by being trained to create a generative model which can produce the original set of data...
.
Bayesian probability, has been developed by a large field with a wide range of important contributors, e.g. Pierre-Simon Laplace
Pierre-Simon Laplace
Pierre-Simon, marquis de Laplace was a French mathematician and astronomer whose work was pivotal to the development of mathematical astronomy and statistics. He summarized and extended the work of his predecessors in his five volume Mécanique Céleste...
, Thomas Bayes
Thomas Bayes
Thomas Bayes was an English mathematician and Presbyterian minister, known for having formulated a specific case of the theorem that bears his name: Bayes' theorem...
, Harold Jeffreys
Harold Jeffreys
Sir Harold Jeffreys, FRS was a mathematician, statistician, geophysicist, and astronomer. His seminal book Theory of Probability, which first appeared in 1939, played an important role in the revival of the Bayesian view of probability.-Biography:Jeffreys was born in Fatfield, Washington, County...
, Richard Cox
Richard Threlkeld Cox
Richard Threlkeld Cox was a professor of physics at Johns Hopkins University, known for Cox's theorem relating to the foundations of probability....
and Edwin Jaynes
Edwin Thompson Jaynes
Edwin Thompson Jaynes was Wayman Crow Distinguished Professor of Physics at Washington University in St. Louis...
has developed mathematical techniques and procedures for treating probability as the degree of plausibility which should be assigned to a given supposition or hypothesis based on the available evidence. In 1988 E.T. Jaynes presented a framework for using Bayesian Probability to model mental processes. It was thus realized early on that the Bayesian statistical framework holds the potential to lead to insights into the function of the nervous system.
A wide range of approaches exist that link Bayesian ideas to the function of the brain.
- Psychophysics: Many aspects of human perceptual or motor behavior are modeled with Bayesian statistics. This approach, with its emphasis on behavioral outcomes as the ultimate expressions of neural information processing, is also known for modeling sensory and motor decisions using Bayesian decision theory. Examples are the work of Landy, Jacobs, Jordan, Knill, Kording and Wolpert.
- Neural coding: Many theoretical studies ask how the nervous system could implement Bayesian algorithms. Examples are the work of Pouget, Zemel, Deneve, Latham, Hinton and Dayan. George and HawkinsJeff HawkinsJeffrey Hawkins is the founder of Palm Computing and Handspring...
published a paper that establishes a model of cortical information processing called Hierarchical Temporal MemoryHierarchical Temporal MemoryHierarchical temporal memory is a machine learning model developed by Jeff Hawkins and Dileep George of Numenta, Inc. that models some of the structural and algorithmic properties of the neocortex. HTM is a biomimetic model based on the memory-prediction theory of brain function described by Jeff...
that is based on Bayesian network of Markov chains. They further map this mathematical model to the existing knowledge about the architecture of cortex and show how neurons could recognize patterns by hierarchical Bayesian inference. - Electrophysiology: A number of recent electrophysiological studies focus on the representation of probabilities in the nervous system. Examples are the work of Shadlen and Schultz.
- Predictive Coding: A neurobiologically plausible scheme for inferring the causes of sensory input based on minimizing prediction error. These schemes are related formally to Kalman filterKalman filterIn statistics, the Kalman filter is a mathematical method named after Rudolf E. Kálmán. Its purpose is to use measurements observed over time, containing noise and other inaccuracies, and produce values that tend to be closer to the true values of the measurements and their associated calculated...
ing and other Bayesian update schemes.
Free energy and the brain
During the 1990s some researchers such as Geoffrey HintonGeoffrey Hinton
Geoffrey Hinton is a British born informatician most noted for his work on the mathematics and applications of neural networks, and their relationship to information theory.-Career:...
and Karl Friston began examining the concept of 'free energy
Thermodynamic free energy
The thermodynamic free energy is the amount of work that a thermodynamic system can perform. The concept is useful in the thermodynamics of chemical or thermal processes in engineering and science. The free energy is the internal energy of a system less the amount of energy that cannot be used to...
' as a calculably tractable measure of the discrepancy between actual features of the world and representations of those features captured by neural network models..
A synthesis has been attempted recently by Karl Friston, in which the Bayesian brain emerges from a general principle of free energy minimisation. In this framework, both action and perception are seen as a consequence of suppressing free-energy, leading to perceptual and active inference and a more embodied (enactive) view of the Bayesian brain. Using Variational Bayes
Variational Bayes
Variational Bayesian methods, also called ensemble learning, are a family of techniques for approximating intractable integrals arising in Bayesian inference and machine learning...
ian methods, it can be shown how internal model
Internal model
An internal model is a postulated neural process that simulates the response of the motor system in order to estimate the outcome of a motor command....
s of the world are updated by sensory information to minimize free energy or the discrepancy between sensory input and predictions of that input. This can be cast (in neurobiologically plausible terms) as predictive coding or, more generally, Bayesian filtering.
According to Friston:
"The free-energy considered here represents a bound on the surprise inherent in any exchange with the environment, under expectations encoded by its state or configuration. A system can minimise free energy by changing its configuration to change the way it samples the environment, or to change its expectations. These changes correspond to action and perception, respectively, and lead to an adaptive exchange with the environment that is characteristic of biological systems. This treatment implies that the system’s state and structure encode an implicit and probabilistic model of the environment."
This area of research was summarized in terms understandable by the layperson in a 2008 article in New Scientist
New Scientist
New Scientist is a weekly non-peer-reviewed English-language international science magazine, which since 1996 has also run a website, covering recent developments in science and technology for a general audience. Founded in 1956, it is published by Reed Business Information Ltd, a subsidiary of...
that offered a unifying theory of brain function. Friston makes the following claims about the explanatory power of the theory:
"This model of brain function can explain a wide range of anatomical and physiological aspects of brain systems; for example, the hierarchical deployment of cortical areas, recurrent architectures using forward and backward connections and functional asymmetries in these connections. In terms of synaptic physiology, it predicts associative plasticity and, for dynamic models, spike-timing-dependent plasticity. In terms of electrophysiology it accounts for classical and extra-classical receptive field effects and long-latency or endogenous components of evoked cortical responses. It predicts the attenuation of responses encoding prediction error with perceptual learning and explains many phenomena like repetition suppression, mismatch negativity and the P300 in electroencephalography. In psychophysical terms, it accounts for the behavioural correlates of these physiological phenomena, e.g., primingPriming (psychology)Priming is an implicit memory effect in which exposure to a stimulus influences a response to a later stimulus. It can occur following perceptual, semantic, or conceptual stimulus repetition...
, and global precedence."
"It is fairly easy to show that both perceptual inference and learning rest on a minimisation of free energy or suppression of prediction error."