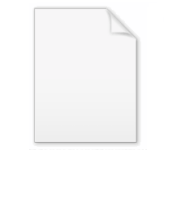
Cache language model
Encyclopedia
A cache language model is a type of statistical language model
. These occur in the natural language processing
subfield of computer science
and assign probabilities
to given sequences of words by means of a probability distribution
. Statistical language models are key components of speech recognition
systems and of many machine translation
systems: they tell such systems which possible output word sequences are probable and which are improbable. The particular characteristic of a cache language model is that it contains a cache component
and assigns relatively high probabilities to words or word sequences that occur elsewhere in a given text. The primary, but by no means sole, use of cache language models is in speech recognition systems.
To understand why it is a good idea for a statistical language model to contain a cache component one might consider someone who is dictating a letter about elephants to a speech recognition system. Standard (non-cache) N-gram
language models will assign a very low probability to the word “elephant” because it is a very rare word in English
. If the speech recognition system does not contain a cache component the person dictating the letter may be annoyed: each time the word “elephant” is spoken another sequence of words with a higher probability according to the N-gram language model may be recognized (e.g., “tell a plan”). These erroneous sequences will have to be deleted manually and replaced in the text by “elephant” each time “elephant” is spoken. If the system has a cache language model, “elephant” will still probably be misrecognized the first time it is spoken and will have to be entered into the text manually; however, from this point on the system is aware that “elephant” is likely to occur again – the estimated probability of occurrence of “elephant” has been increased, making it more likely that if it is spoken it will be recognized correctly. Once “elephant” has occurred several times the system is likely to recognize it correctly every time it is spoken until the letter has been completely dictated. This increase in the probability assigned to the occurrence of "elephant" is an example of a consequence of machine learning
and more specifically of pattern recognition
.
There exist variants of the cache language model in which not only single words but also multi-word sequences that have occurred previously are assigned higher probabilities (e.g., if “San Francisco” occurred near the beginning of the text subsequent instances of it would be assigned a higher probability).
The cache language model was first proposed in a paper published in 1990, after which the IBM
speech-recognition group experimented with the concept. The group found that implementation of a form of cache language model yielded a 24% drop in word-error rates
once the first few hundred words of a document had been dictated. A detailed survey of language modeling techniques concluded that the cache language model was one of the few new language modeling techniques that yielded improvements over the standard N-gram approach: “Our caching results show that caching is by far the most useful technique for perplexity reduction at small and medium training data
sizes".
The development of the cache language model has generated considerable interest among those concerned with computational linguistics
in general and statistical natural language processing in particular: recently there has been interest in applying the cache language model in the field of statistical machine translation.
The success of the cache language model in improving word prediction rests on the human tendency to use words in a “bursty” fashion: when one is discussing a certain topic in a certain context the frequency with which one uses certain words will be quite different from their frequencies when one is discussing other topics in other contexts. The traditional N-gram language models, which rely entirely on information from a very small number (four, three, or two) of words preceding the word to which a probability is to be assigned, do not adequately model this “burstiness”.
Language model
A statistical language model assigns a probability to a sequence of m words P by means of a probability distribution.Language modeling is used in many natural language processing applications such as speech recognition, machine translation, part-of-speech tagging, parsing and information...
. These occur in the natural language processing
Natural language processing
Natural language processing is a field of computer science and linguistics concerned with the interactions between computers and human languages; it began as a branch of artificial intelligence....
subfield of computer science
Computer science
Computer science or computing science is the study of the theoretical foundations of information and computation and of practical techniques for their implementation and application in computer systems...
and assign probabilities
Probability
Probability is ordinarily used to describe an attitude of mind towards some proposition of whose truth we arenot certain. The proposition of interest is usually of the form "Will a specific event occur?" The attitude of mind is of the form "How certain are we that the event will occur?" The...
to given sequences of words by means of a probability distribution
Probability distribution
In probability theory, a probability mass, probability density, or probability distribution is a function that describes the probability of a random variable taking certain values....
. Statistical language models are key components of speech recognition
Speech recognition
Speech recognition converts spoken words to text. The term "voice recognition" is sometimes used to refer to recognition systems that must be trained to a particular speaker—as is the case for most desktop recognition software...
systems and of many machine translation
Machine translation
Machine translation, sometimes referred to by the abbreviation MT is a sub-field of computational linguistics that investigates the use of computer software to translate text or speech from one natural language to another.On a basic...
systems: they tell such systems which possible output word sequences are probable and which are improbable. The particular characteristic of a cache language model is that it contains a cache component
Cache
In computer engineering, a cache is a component that transparently stores data so that future requests for that data can be served faster. The data that is stored within a cache might be values that have been computed earlier or duplicates of original values that are stored elsewhere...
and assigns relatively high probabilities to words or word sequences that occur elsewhere in a given text. The primary, but by no means sole, use of cache language models is in speech recognition systems.
To understand why it is a good idea for a statistical language model to contain a cache component one might consider someone who is dictating a letter about elephants to a speech recognition system. Standard (non-cache) N-gram
N-gram
In the fields of computational linguistics and probability, an n-gram is a contiguous sequence of n items from a given sequence of text or speech. The items in question can be phonemes, syllables, letters, words or base pairs according to the application...
language models will assign a very low probability to the word “elephant” because it is a very rare word in English
English language
English is a West Germanic language that arose in the Anglo-Saxon kingdoms of England and spread into what was to become south-east Scotland under the influence of the Anglian medieval kingdom of Northumbria...
. If the speech recognition system does not contain a cache component the person dictating the letter may be annoyed: each time the word “elephant” is spoken another sequence of words with a higher probability according to the N-gram language model may be recognized (e.g., “tell a plan”). These erroneous sequences will have to be deleted manually and replaced in the text by “elephant” each time “elephant” is spoken. If the system has a cache language model, “elephant” will still probably be misrecognized the first time it is spoken and will have to be entered into the text manually; however, from this point on the system is aware that “elephant” is likely to occur again – the estimated probability of occurrence of “elephant” has been increased, making it more likely that if it is spoken it will be recognized correctly. Once “elephant” has occurred several times the system is likely to recognize it correctly every time it is spoken until the letter has been completely dictated. This increase in the probability assigned to the occurrence of "elephant" is an example of a consequence of machine learning
Machine learning
Machine learning, a branch of artificial intelligence, is a scientific discipline concerned with the design and development of algorithms that allow computers to evolve behaviors based on empirical data, such as from sensor data or databases...
and more specifically of pattern recognition
Pattern recognition
In machine learning, pattern recognition is the assignment of some sort of output value to a given input value , according to some specific algorithm. An example of pattern recognition is classification, which attempts to assign each input value to one of a given set of classes...
.
There exist variants of the cache language model in which not only single words but also multi-word sequences that have occurred previously are assigned higher probabilities (e.g., if “San Francisco” occurred near the beginning of the text subsequent instances of it would be assigned a higher probability).
The cache language model was first proposed in a paper published in 1990, after which the IBM
IBM
International Business Machines Corporation or IBM is an American multinational technology and consulting corporation headquartered in Armonk, New York, United States. IBM manufactures and sells computer hardware and software, and it offers infrastructure, hosting and consulting services in areas...
speech-recognition group experimented with the concept. The group found that implementation of a form of cache language model yielded a 24% drop in word-error rates
Word error rate
Word error rate is a common metric of the performance of a speech recognition or machine translation system.The general difficulty of measuring performance lies in the fact that the recognized word sequence can have a different length from the reference word sequence...
once the first few hundred words of a document had been dictated. A detailed survey of language modeling techniques concluded that the cache language model was one of the few new language modeling techniques that yielded improvements over the standard N-gram approach: “Our caching results show that caching is by far the most useful technique for perplexity reduction at small and medium training data
Training set
A training set is a set of data used in various areas of information science to discover potentially predictive relationships. Training sets are used in artificial intelligence, machine learning, genetic programming, intelligent systems, and statistics...
sizes".
The development of the cache language model has generated considerable interest among those concerned with computational linguistics
Computational linguistics
Computational linguistics is an interdisciplinary field dealing with the statistical or rule-based modeling of natural language from a computational perspective....
in general and statistical natural language processing in particular: recently there has been interest in applying the cache language model in the field of statistical machine translation.
The success of the cache language model in improving word prediction rests on the human tendency to use words in a “bursty” fashion: when one is discussing a certain topic in a certain context the frequency with which one uses certain words will be quite different from their frequencies when one is discussing other topics in other contexts. The traditional N-gram language models, which rely entirely on information from a very small number (four, three, or two) of words preceding the word to which a probability is to be assigned, do not adequately model this “burstiness”.
See also
- Artificial intelligenceArtificial intelligenceArtificial intelligence is the intelligence of machines and the branch of computer science that aims to create it. AI textbooks define the field as "the study and design of intelligent agents" where an intelligent agent is a system that perceives its environment and takes actions that maximize its...
- History of natural language processingHistory of natural language processingThe history of natural language processing describes the advances of natural language processing. There is some overlap with the history of machine translation and the history of artificial intelligence.-Theoretical history:...
- History of machine translationHistory of machine translationThe history of machine translation generally starts in the 1950s, although work can be found from earlier periods. The Georgetown experiment in 1954 involved fully automatic translation of more than sixty Russian sentences into English. The experiment was a great success and ushered in an era of...
- Speech recognitionSpeech recognitionSpeech recognition converts spoken words to text. The term "voice recognition" is sometimes used to refer to recognition systems that must be trained to a particular speaker—as is the case for most desktop recognition software...
- Statistical machine translationStatistical machine translationStatistical machine translation is a machine translation paradigm where translations are generated on the basis of statistical models whose parameters are derived from the analysis of bilingual text corpora...