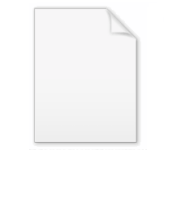
Feedforward neural networks
Encyclopedia
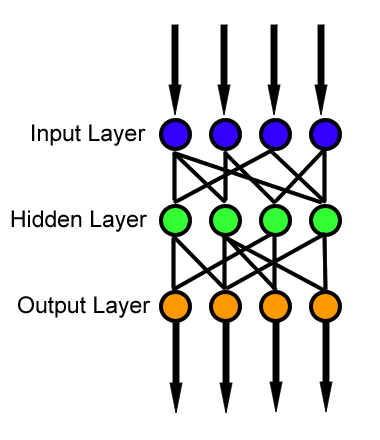
Artificial neural network
An artificial neural network , usually called neural network , is a mathematical model or computational model that is inspired by the structure and/or functional aspects of biological neural networks. A neural network consists of an interconnected group of artificial neurons, and it processes...
where connections between the units do not form a directed cycle. This is different from recurrent neural networks.
The feedforward neural network was the first and arguably simplest type of artificial neural network devised. In this network, the information moves in only one direction, forward, from the input nodes, through the hidden nodes (if any) and to the output nodes. There are no cycles or loops in the network.
Single-layer perceptron
The earliest kind of neural network is a single-layer perceptron network, which consists of a single layer of output nodes; the inputs are fed directly to the outputs via a series of weights. In this way it can be considered the simplest kind of feed-forward network. The sum of the products of the weights and the inputs is calculated in each node, and if the value is above some threshold (typically 0) the neuron fires and takes the activated value (typically 1); otherwise it takes the deactivated value (typically -1). Neurons with this kind of activation functionActivation function
In computational networks, the activation function of a node defines the output of that node given an input or set of inputs. A standard computer chip circuit can be seen as a digital network of activation functions that can be "ON" or "OFF" , depending on input. This is similar to the behavior of...
are also called Artificial neurons or linear threshold units. In the literature the term perceptron
Perceptron
The perceptron is a type of artificial neural network invented in 1957 at the Cornell Aeronautical Laboratory by Frank Rosenblatt. It can be seen as the simplest kind of feedforward neural network: a linear classifier.- Definition :...
often refers to networks consisting of just one of these units. A similar neuron was described by Warren McCulloch and Walter Pitts
Walter Pitts
Walter Harry Pitts, Jr. was a logician who worked in the field of cognitive psychology.He proposed landmark theoretical formulations of neural activity and emergent processes that influenced diverse fields such as cognitive sciences and psychology, philosophy, neurosciences, computer science,...
in the 1940s.
A perceptron can be created using any values for the activated and deactivated states as long as the threshold value lies between the two. Most perceptrons have outputs of 1 or -1 with a threshold of 0 and there is some evidence that such networks can be trained more quickly than networks created from nodes with different activation and deactivation values.
Perceptrons can be trained by a simple learning algorithm that is usually called the delta rule
Delta rule
The delta rule is a gradient descent learning rule for updating the weights of the artificial neurons in a single-layer perceptron. It is a special case of the more general backpropagation algorithm...
. It calculates the errors between calculated output and sample output data, and uses this to create an adjustment to the weights, thus implementing a form of gradient descent
Gradient descent
Gradient descent is a first-order optimization algorithm. To find a local minimum of a function using gradient descent, one takes steps proportional to the negative of the gradient of the function at the current point...
.
Single-unit perceptrons are only capable of learning linearly separable
Linearly separable
In geometry, two sets of points in a two-dimensional space are linearly separable if they can be completely separated by a single line. In general, two point sets are linearly separable in n-dimensional space if they can be separated by a hyperplane....
patterns; in 1969 in a famous monograph
Monograph
A monograph is a work of writing upon a single subject, usually by a single author.It is often a scholarly essay or learned treatise, and may be released in the manner of a book or journal article. It is by definition a single document that forms a complete text in itself...
entitled Perceptrons
Perceptrons (book)
Perceptrons: an introduction to computational geometry is a book authored by Marvin Minsky and Seymour Papert, published in 1969. An edition with handwritten corrections and additions was released in the early 1970s...
Marvin Minsky
Marvin Minsky
Marvin Lee Minsky is an American cognitive scientist in the field of artificial intelligence , co-founder of Massachusetts Institute of Technology's AI laboratory, and author of several texts on AI and philosophy.-Biography:...
and Seymour Papert
Seymour Papert
Seymour Papert is an MIT mathematician, computer scientist, and educator. He is one of the pioneers of artificial intelligence, as well as an inventor of the Logo programming language....
showed that it was impossible for a single-layer perceptron network to learn an XOR function. It is often believed that they also conjectured (incorrectly) that a similar result would hold for a multi-layer perceptron network. However, this is not true, as both Minsky and Papert already knew that multi-layer perceptrons were capable of producing an XOR Function. (See the page on Perceptrons
Perceptrons (book)
Perceptrons: an introduction to computational geometry is a book authored by Marvin Minsky and Seymour Papert, published in 1969. An edition with handwritten corrections and additions was released in the early 1970s...
for more information.)
Although a single threshold unit is quite limited in its computational power, it has been shown that networks of parallel threshold units can approximate any continuous function from a compact interval of the real numbers into the interval [-1,1]. This very recent result can be found in [ Peter Auer, Harald Burgsteiner and Wolfgang Maass: A learning rule for very simple universal approximators consisting of a single layer of perceptrons, 2008].
A multi-layer neural network can compute a continuous output instead of a step function
Step function
In mathematics, a function on the real numbers is called a step function if it can be written as a finite linear combination of indicator functions of intervals...
. A common choice is the so-called logistic function
Logistic function
A logistic function or logistic curve is a common sigmoid curve, given its name in 1844 or 1845 by Pierre François Verhulst who studied it in relation to population growth. It can model the "S-shaped" curve of growth of some population P...
:
(In general form, f(X) is in place of x, where f(X) is an analytic function
Analytic function
In mathematics, an analytic function is a function that is locally given by a convergent power series. There exist both real analytic functions and complex analytic functions, categories that are similar in some ways, but different in others...
in set of x's.) With this choice, the single-layer network is identical to the logistic regression
Logistic regression
In statistics, logistic regression is used for prediction of the probability of occurrence of an event by fitting data to a logit function logistic curve. It is a generalized linear model used for binomial regression...
model, widely used in statistical model
Statistical model
A statistical model is a formalization of relationships between variables in the form of mathematical equations. A statistical model describes how one or more random variables are related to one or more random variables. The model is statistical as the variables are not deterministically but...
ing. The logistic function
Logistic function
A logistic function or logistic curve is a common sigmoid curve, given its name in 1844 or 1845 by Pierre François Verhulst who studied it in relation to population growth. It can model the "S-shaped" curve of growth of some population P...
is also known as the sigmoid function
Sigmoid function
Many natural processes, including those of complex system learning curves, exhibit a progression from small beginnings that accelerates and approaches a climax over time. When a detailed description is lacking, a sigmoid function is often used. A sigmoid curve is produced by a mathematical...
. It has a continuous derivative, which allows it to be used in backpropagation. This function is also preferred because its derivative is easily calculated:
-
(times
, in general form, according to the Chain Rule
Chain ruleIn calculus, the chain rule is a formula for computing the derivative of the composition of two or more functions. That is, if f is a function and g is a function, then the chain rule expresses the derivative of the composite function in terms of the derivatives of f and g.In integration, the...
)
Multi-layer perceptron
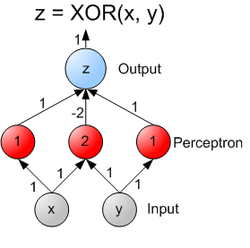
The universal approximation theorem
Universal approximation theorem
In the mathematical theory of neural networks, the universal approximation theorem states that the standard multilayer feed-forward network with a single hidden layer that contains finite number of hidden neurons, and with arbitrary activation function are universal approximators on a compact...
for neural networks states that every continuous function that maps intervals of real numbers to some output interval of real numbers can be approximated arbitrarily closely by a multi-layer perceptron with just one hidden layer. This result holds only for restricted classes of activation functions, e.g. for the sigmoidal functions.
Multi-layer networks use a variety of learning techniques, the most popular being back-propagation. Here, the output values are compared with the correct answer to compute the value of some predefined error-function. By various techniques, the error is then fed back through the network. Using this information, the algorithm adjusts the weights of each connection in order to reduce the value of the error function by some small amount. After repeating this process for a sufficiently large number of training cycles, the network will usually converge to some state where the error of the calculations is small. In this case, one would say that the network has learned a certain target function. To adjust weights properly, one applies a general method for non-linear optimization
Optimization (mathematics)
In mathematics, computational science, or management science, mathematical optimization refers to the selection of a best element from some set of available alternatives....
that is called gradient descent
Gradient descent
Gradient descent is a first-order optimization algorithm. To find a local minimum of a function using gradient descent, one takes steps proportional to the negative of the gradient of the function at the current point...
. For this, the derivative of the error function with respect to the network weights is calculated, and the weights are then changed such that the error decreases (thus going downhill on the surface of the error function). For this reason, back-propagation can only be applied on networks with differentiable activation functions.
In general, the problem of teaching a network to perform well, even on samples that were not used as training samples, is a quite subtle issue that requires additional techniques. This is especially important for cases where only very limited numbers of training samples are available. The danger is that the network overfits
Overfitting
In statistics, overfitting occurs when a statistical model describes random error or noise instead of the underlying relationship. Overfitting generally occurs when a model is excessively complex, such as having too many parameters relative to the number of observations...
the training data and fails to capture the true statistical process generating the data. Computational learning theory
Computational learning theory
In theoretical computer science, computational learning theory is a mathematical field related to the analysis of machine learning algorithms.-Overview:Theoretical results in machine learning mainly deal with a type of...
is concerned with training classifiers on a limited amount of data. In the context of neural networks a simple heuristic
Heuristic
Heuristic refers to experience-based techniques for problem solving, learning, and discovery. Heuristic methods are used to speed up the process of finding a satisfactory solution, where an exhaustive search is impractical...
, called early stopping
Early stopping
In machine learning, early stopping is a form of regularization used when a machine learning model is trained by on-line gradient descent. In early stopping, the training set is split into a new training set and a validation set. Gradient descent is applied to the new training set...
, often ensures that the network will generalize well to examples not in the training set.
Other typical problems of the back-propagation algorithm are the speed of convergence and the possibility of ending up in a local minimum of the error function. Today there are practical solutions that make back-propagation in multi-layer perceptrons the solution of choice for many machine learning
Machine learning
Machine learning, a branch of artificial intelligence, is a scientific discipline concerned with the design and development of algorithms that allow computers to evolve behaviors based on empirical data, such as from sensor data or databases...
tasks.
ADALINE
ADALINE stands for Adaptive Linear Element. It was developed by Professor Bernard WidrowBernard Widrow
Bernard Widrow is a U.S. professor of electrical engineering at Stanford University. He is the co-inventor of the Widrow–Hoff least mean squares filter adaptive algorithm with his then doctoral student Ted Hoff...
and his graduate student Ted Hoff at Stanford University
Stanford University
The Leland Stanford Junior University, commonly referred to as Stanford University or Stanford, is a private research university on an campus located near Palo Alto, California. It is situated in the northwestern Santa Clara Valley on the San Francisco Peninsula, approximately northwest of San...
in 1960. It is based on the McCulloch-Pitts model and consists of a weight, a bias and a summation function.
Operation:

Its adaptation is defined through a cost function (error metric) of the residual


Mean squared error
In statistics, the mean squared error of an estimator is one of many ways to quantify the difference between values implied by a kernel density estimator and the true values of the quantity being estimated. MSE is a risk function, corresponding to the expected value of the squared error loss or...
error metric

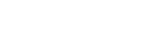
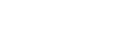
The Adaline has practical applications in the controls area. A single neuron with tap delayed inputs (the number of inputs is bounded by the lowest frequency present and the Nyquist rate) can be used to determine the higher order transfer function of a physical system via the bi-linear z-transform. This is done as the Adaline is, functionally, an adaptive FIR filter. Like the single-layer perceptron, ADALINE has a counterpart in statistical modelling, in this case least squares
Least squares
The method of least squares is a standard approach to the approximate solution of overdetermined systems, i.e., sets of equations in which there are more equations than unknowns. "Least squares" means that the overall solution minimizes the sum of the squares of the errors made in solving every...
regression
Regression analysis
In statistics, regression analysis includes many techniques for modeling and analyzing several variables, when the focus is on the relationship between a dependent variable and one or more independent variables...
.
There is an extension of the Adaline, called the Multiple Adaline (MADALINE) that consists of two or more adalines serially connected.