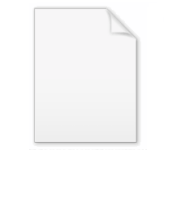
Collaborative filtering
Encyclopedia
Collaborative filtering (CF) is the process of filtering for information or patterns using techniques involving collaboration among multiple agents, viewpoints, data sources, etc. Applications of collaborative filtering typically involve very large data sets. Collaborative filtering methods have been applied to many different kinds of data including sensing and monitoring data - such as in mineral exploration, environmental sensing over large areas or multiple sensors; financial data - such as financial service institutions that integrate many financial sources; or in electronic commerce and web 2.0 applications where the focus is on user data, etc. The remainder of this discussion focuses on collaborative filtering for user data, although some of the methods and approaches may apply to the other major applications as well.
Collaborative filtering is a method of making automatic predictions (filtering) about the interests of a user by collecting preferences or taste
information from many users (collaborating). The underlying assumption of the CF approach is that those who agreed in the past tend to agree again in the future. For example, a collaborative filtering or recommendation system
for television
tastes could make predictions about which television show a user should like given a partial list of that user's tastes (likes or dislikes). Note that these predictions are specific to the user, but use information gleaned from many users. This differs from the simpler approach of giving an average
(non-specific) score for each item of interest, for example based on its number of votes.
This falls under the category of user-based collaborative filtering. A specific application of this is the user-based Nearest Neighbor algorithm
.
Alternatively, item-based collaborative filtering invented by Amazon.com
(users who bought x also bought y), proceeds in an item-centric manner:
See, for example, the Slope One
item-based collaborative filtering family.
Another form of collaborative filtering can be based on implicit observations of normal user behavior (as opposed to the artificial behavior imposed by a rating task). In these systems you observe what a user has done together with what all users have done (what music they have listened to, what items they have bought) and use that data to predict the user's behavior in the future or to predict how a user might like to behave if only they were given a chance. These predictions then have to be filtered through business logic
to determine how these predictions might affect what a business system ought to do. It is, for instance, not useful to offer to sell somebody some music if they already have demonstrated that they own that music or, considering another example, it is not useful to suggest more travel guides for Paris to someone who already bought a travel guide for this city.
Relying on a scoring or rating system which is averaged across all users ignores specific demands of a user, and is particularly poor in tasks where there is large variation in interest, for example in the recommendation of music. However, there are other methods to combat information explosion, for example web search, data clustering
, and more.
The neighborhood-based algorithm calculates the similarity between two users or items, produces a prediction for the user taking the weighted average of all the ratings. Similarity computation between items or users is an important part of this approach. Multiple mechanisms such as Pearson correlation
and vector cosine
based similarity are used for this.
The user based top-N recommendation algorithm identifies the k most similar users to an active user using similarity based vector model. After the k most similar users are found, their corresponding user-item matrices are aggregated to identify the set of items to be recommended. A popular method to find the similar users is the Locality sensitive hashing
, which implements the nearest neighbor mechanism
in linear time.
The advantages with this approach include: the explainability of the results, which is an important aspect of recommendation systems; it is easy to create and use; new data can be added easily and incrementally; it need not consider the content of the items being recommended; and the mechanism scales well with co-rated items.
There are several disadvantages with this approach. First, it depends on human ratings. Second, its performance decreases when data gets sparse, which is frequent with web related items. This prevents the scalability of this approach and has problems with large datasets. Third, it cannot handle new users or new items.
, machine learning
algorithms to find patterns based on training data. These are used to make predictions for real data. There are many model based CF algorithms. These include Bayesian Networks, clustering models, latent semantic models
such as singular value decomposition
, probabilistic latent semantic analysis
, Multiple Multiplicative Factor, Latent Dirichlet allocation
and markov decision process
based models.
This approach has a more holistic goal to uncover latent factors that explain observed ratings. Most of the models are based on creating a classification or clustering technique to identify the user based on the test set. The number of the parameters can be reduced based on types of principal component analysis.
There are several advantages with this paradigm. It handles the sparsity better than memory based ones. This helps with scalability with large data sets. It improves the prediction performance. It gives an intuitive rationale for the recommendations.
The disadvantages with this approach are in the expensive model building. One needs to have a tradeoff between prediction performance and scalability. One can lose useful information due to reduction models. A number of models have difficulty explaining the predictions.
Collaborative filtering is a method of making automatic predictions (filtering) about the interests of a user by collecting preferences or taste
Taste (sociology)
Taste as an aesthetic, sociological, economic and anthropological concept refers to a cultural patterns of choice and preference. While taste is often understood as a biological concept, it can also be reasonably studied as a social or cultural phenomenon. Taste is about drawing distinctions...
information from many users (collaborating). The underlying assumption of the CF approach is that those who agreed in the past tend to agree again in the future. For example, a collaborative filtering or recommendation system
Recommendation system
Recommender systems, recommendation systems, recommendation engines, recommendation frameworks, recommendation platforms or simply recommender form or work from a specific type of information filtering system technique that attempts to recommend information items Recommender systems, recommendation...
for television
Television
Television is a telecommunication medium for transmitting and receiving moving images that can be monochrome or colored, with accompanying sound...
tastes could make predictions about which television show a user should like given a partial list of that user's tastes (likes or dislikes). Note that these predictions are specific to the user, but use information gleaned from many users. This differs from the simpler approach of giving an average
Average
In mathematics, an average, or central tendency of a data set is a measure of the "middle" value of the data set. Average is one form of central tendency. Not all central tendencies should be considered definitions of average....
(non-specific) score for each item of interest, for example based on its number of votes.
Methodology
Collaborative filtering systems have many forms, but many common systems can be reduced to two steps:- Look for users who share the same rating patterns with the active user (the user whom the prediction is for).
- Use the ratings from those like-minded users found in step 1 to calculate a prediction for the active user
This falls under the category of user-based collaborative filtering. A specific application of this is the user-based Nearest Neighbor algorithm
K-nearest neighbor algorithm
In pattern recognition, the k-nearest neighbor algorithm is a method for classifying objects based on closest training examples in the feature space. k-NN is a type of instance-based learning, or lazy learning where the function is only approximated locally and all computation is deferred until...
.
Alternatively, item-based collaborative filtering invented by Amazon.com
Amazon.com
Amazon.com, Inc. is a multinational electronic commerce company headquartered in Seattle, Washington, United States. It is the world's largest online retailer. Amazon has separate websites for the following countries: United States, Canada, United Kingdom, Germany, France, Italy, Spain, Japan, and...
(users who bought x also bought y), proceeds in an item-centric manner:
- Build an item-item matrix determining relationships between pairs of items
- Using the matrix, and the data on the current user, infer his taste
See, for example, the Slope One
Slope One
Slope One is a family of algorithms used for collaborative filtering, introduced in a 2005 paper by Daniel Lemire and Anna Maclachlan. Arguably, it is the simplest form of non-trivial item-based collaborative filtering based on ratings...
item-based collaborative filtering family.
Another form of collaborative filtering can be based on implicit observations of normal user behavior (as opposed to the artificial behavior imposed by a rating task). In these systems you observe what a user has done together with what all users have done (what music they have listened to, what items they have bought) and use that data to predict the user's behavior in the future or to predict how a user might like to behave if only they were given a chance. These predictions then have to be filtered through business logic
Business logic
Business logic, or domain logic, is a non-technical term generally used to describe the functional algorithms that handle information exchange between a database and a user interface.- Scope of business logic :Business logic:...
to determine how these predictions might affect what a business system ought to do. It is, for instance, not useful to offer to sell somebody some music if they already have demonstrated that they own that music or, considering another example, it is not useful to suggest more travel guides for Paris to someone who already bought a travel guide for this city.
Relying on a scoring or rating system which is averaged across all users ignores specific demands of a user, and is particularly poor in tasks where there is large variation in interest, for example in the recommendation of music. However, there are other methods to combat information explosion, for example web search, data clustering
Data clustering
Cluster analysis or clustering is the task of assigning a set of objects into groups so that the objects in the same cluster are more similar to each other than to those in other clusters....
, and more.
Memory-Based
This mechanism uses user rating data to compute similarity between users or items. This is used for making recommendations. This was the earlier mechanism and is used in many commercial systems. It is easy to implement and is effective. Typical examples of this mechanism are neighborhood based CF and item-based/user-based top-N recommendations.The neighborhood-based algorithm calculates the similarity between two users or items, produces a prediction for the user taking the weighted average of all the ratings. Similarity computation between items or users is an important part of this approach. Multiple mechanisms such as Pearson correlation
Pearson product-moment correlation coefficient
In statistics, the Pearson product-moment correlation coefficient is a measure of the correlation between two variables X and Y, giving a value between +1 and −1 inclusive...
and vector cosine
Cosine similarity
Cosine similarity is a measure of similarity between two vectors by measuring the cosine of the angle between them. The cosine of 0 is 1, and less than 1 for any other angle. The cosine of the angle between two vectors thus determines whether two vectors are pointing in roughly the same...
based similarity are used for this.
The user based top-N recommendation algorithm identifies the k most similar users to an active user using similarity based vector model. After the k most similar users are found, their corresponding user-item matrices are aggregated to identify the set of items to be recommended. A popular method to find the similar users is the Locality sensitive hashing
Locality sensitive hashing
Locality-sensitive hashing is a method of performing probabilistic dimension reduction of high-dimensional data. The basic idea is to hash the input items so that similar items are mapped to the same buckets with high probability .-Definition:An LSH family \mathcal F is defined fora...
, which implements the nearest neighbor mechanism
Nearest neighbor search
Nearest neighbor search , also known as proximity search, similarity search or closest point search, is an optimization problem for finding closest points in metric spaces. The problem is: given a set S of points in a metric space M and a query point q ∈ M, find the closest point in S to q...
in linear time.
The advantages with this approach include: the explainability of the results, which is an important aspect of recommendation systems; it is easy to create and use; new data can be added easily and incrementally; it need not consider the content of the items being recommended; and the mechanism scales well with co-rated items.
There are several disadvantages with this approach. First, it depends on human ratings. Second, its performance decreases when data gets sparse, which is frequent with web related items. This prevents the scalability of this approach and has problems with large datasets. Third, it cannot handle new users or new items.
Model-Based
Models are developed using data miningData mining
Data mining , a relatively young and interdisciplinary field of computer science is the process of discovering new patterns from large data sets involving methods at the intersection of artificial intelligence, machine learning, statistics and database systems...
, machine learning
Machine learning
Machine learning, a branch of artificial intelligence, is a scientific discipline concerned with the design and development of algorithms that allow computers to evolve behaviors based on empirical data, such as from sensor data or databases...
algorithms to find patterns based on training data. These are used to make predictions for real data. There are many model based CF algorithms. These include Bayesian Networks, clustering models, latent semantic models
Latent semantic indexing
Latent Semantic Indexing is an indexing and retrieval method that uses a mathematical technique called Singular value decomposition to identify patterns in the relationships between the terms and concepts contained in an unstructured collection of text. LSI is based on the principle that words...
such as singular value decomposition
Singular value decomposition
In linear algebra, the singular value decomposition is a factorization of a real or complex matrix, with many useful applications in signal processing and statistics....
, probabilistic latent semantic analysis
Probabilistic latent semantic analysis
Probabilistic latent semantic analysis , also known as probabilistic latent semantic indexing is a statistical technique for the analysis of two-mode and co-occurrence data. PLSA evolved from latent semantic analysis, adding a sounder probabilistic model...
, Multiple Multiplicative Factor, Latent Dirichlet allocation
Latent Dirichlet allocation
In statistics, latent Dirichlet allocation is a generative model that allows sets of observations to be explained by unobserved groups that explain why some parts of the data are similar...
and markov decision process
Markov decision process
Markov decision processes , named after Andrey Markov, provide a mathematical framework for modeling decision-making in situations where outcomes are partly random and partly under the control of a decision maker. MDPs are useful for studying a wide range of optimization problems solved via...
based models.
This approach has a more holistic goal to uncover latent factors that explain observed ratings. Most of the models are based on creating a classification or clustering technique to identify the user based on the test set. The number of the parameters can be reduced based on types of principal component analysis.
There are several advantages with this paradigm. It handles the sparsity better than memory based ones. This helps with scalability with large data sets. It improves the prediction performance. It gives an intuitive rationale for the recommendations.
The disadvantages with this approach are in the expensive model building. One needs to have a tradeoff between prediction performance and scalability. One can lose useful information due to reduction models. A number of models have difficulty explaining the predictions.
Hybrid
A number of applications combines the memory-based and the model-based CF algorithms. These overcome the limitations of native CF approaches. It improves the prediction performance. Importantly, it overcomes the CF problems such as sparsity and loss of information. However, they have increased complexity and are expensive to implement.Innovations
- New algorithms have been developed for CF as a result of the Netflix prizeNetflix PrizeThe Netflix Prize was an open competition for the best collaborative filtering algorithm to predict user ratings for films, based on previous ratings....
. - Cross-System Collaborative Filtering where user profiles across multiple recommender systems are combined in a privacy preserving manner.
- Robust Collaborative Filtering, where recommendation is stable towards efforts of manipulation. This research area is still active and not completely solved.
See also
- Attention Profiling Mark-up Language (APML)
- Cold startCold startCold start is a potential problem in computer-based information systems which involve a degree of automated data modelling. Specifically, it concerns the issue that the system cannot draw any inferences for users or items about which it has not yet gathered sufficient information.-Systems...
- Collaborative search engineCollaborative search engineCollaborative Search Engines are an emerging trend for Web search and Enterprise search within company intranets. CSEs let users concert their efforts in information retrieval activities, share information resources collaboratively using knowledge tags, and allow experts to guide less experienced...
- Collective intelligenceCollective intelligenceCollective intelligence is a shared or group intelligence that emerges from the collaboration and competition of many individuals and appears in consensus decision making in bacteria, animals, humans and computer networks....
- Customer engagementCustomer engagementCustomer engagement refers to the engagement of customers with one another, with a company or a brand. The initiative for engagement can be either consumer- or company-led and the medium of engagement can be on or offline....
- Enterprise bookmarkingEnterprise bookmarkingEnterprise bookmarking is a method for Enterprise 2.0 users to tag, organize, store, and search bookmarks of both web pages on the Internet and data resources stored in a distributed database or fileserver...
- Preference elicitationPreference elicitationPreference elicitation refers to the problem of developing a decision support system capable of generating recommendations to a user, thus assisting him in decision making. It is important for such a system to model user's preferences accurately, find hidden preferences and avoid redundancy. This...
- Recommendation systemRecommendation systemRecommender systems, recommendation systems, recommendation engines, recommendation frameworks, recommendation platforms or simply recommender form or work from a specific type of information filtering system technique that attempts to recommend information items Recommender systems, recommendation...
- Relevance (information retrieval)Relevance (information retrieval)In information science and information retrieval, relevance denotes how well a retrieved document or set of documents meets the information need of the user.-Types:...
- Reputation systemReputation systemA reputation system computes and publishes reputation scores for a set of objects within a community or domain, based on a collection of opinions that other entities hold about the objects...
- Similarity search
- Social translucenceSocial translucenceSocial translucence is a term that was proposed by Thomas Erickson and Wendy A. Kellogg to refer to "design digital systems that support coherent behavior by making participants and their activities visible to one another"....
- The Long TailThe Long TailThe Long Tail or long tail refers to the statistical property that a larger share of population rests within the tail of a probability distribution than observed under a 'normal' or Gaussian distribution...
External links
- Recommender Systems. Prem Melville and Vikas Sindhwani. In Encyclopedia of Machine Learning, Claude Sammut and Geoffrey Webb (Eds), Springer, 2010.
- Toward the next generation of recommender systems: a survey of the state-of-the-art and possible extensions. Adomavicius, G. and Tuzhilin, A. IEEE Transactions on Knowledge and Data Engineering 06.2005
- Evaluating collaborative filtering recommender systems (DOI: 10.1145/963770.963772)
- GroupLens research papers. GroupLens is one of the research labs that did a lot of pioneering research in collaborative filtering.
- Content-Boosted Collaborative Filtering for Improved Recommendations. Prem Melville, Raymond J. Mooney, and Ramadass Nagarajan. Proceedings of the Eighteenth National Conference on Artificial Intelligence (AAAI-2002), pp. 187–192, Edmonton, Canada, July 2002.
- A collection of past and present "information filtering" projects (including collaborative filtering) at MIT Media Lab
- Eigentaste: A Constant Time Collaborative Filtering Algorithm. Ken Goldberg, Theresa Roeder, Dhruv Gupta, and Chris Perkins. Information Retrieval, 4(2), 133-151. July 2001.
- Methods and Metrics for Cold-Start Recommendations
- A Survey of Collaborative Filtering Techniques Su, Xiaoyuan and Khoshgortaar, Taghi. M
- Google News Personalization: Scalable Online Collaborative Filtering Abhinandan Das, Mayur Datar, Ashutosh Garg, and Shyam Rajaram. International World Wide Web Conference, Proceedings of the 16th international conference on World Wide Web
- Factor in the Neighbors: Scalable and Accurate Collaborative Filtering Yehuda Koren, Transactions on Knowledge Discovery from Data (TKDD) (2009)
- Rating Prediction Using Collaborative Filtering