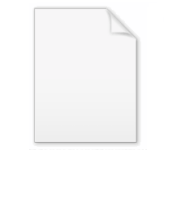
Efficiency (statistics)
Encyclopedia
In statistics
, an efficient estimator is an estimator
that estimates the quantity of interest in some “best possible” manner. The notion of “best possible” relies upon the choice of a particular loss function
— the function which quantifies the relative degree of undesirability of estimation errors of different magnitudes. The most common choice of the loss function is quadratic, resulting in the mean squared error
criterion of optimality.
and is the data sampled from this model. Let be the estimator
for the parameter θ. If this estimator is unbiased
(that is), then the celebrated Cramér–Rao inequality states the variance
of this estimator is bounded from below:
Statistics
Statistics is the study of the collection, organization, analysis, and interpretation of data. It deals with all aspects of this, including the planning of data collection in terms of the design of surveys and experiments....
, an efficient estimator is an estimator
Estimator
In statistics, an estimator is a rule for calculating an estimate of a given quantity based on observed data: thus the rule and its result are distinguished....
that estimates the quantity of interest in some “best possible” manner. The notion of “best possible” relies upon the choice of a particular loss function
Loss function
In statistics and decision theory a loss function is a function that maps an event onto a real number intuitively representing some "cost" associated with the event. Typically it is used for parameter estimation, and the event in question is some function of the difference between estimated and...
— the function which quantifies the relative degree of undesirability of estimation errors of different magnitudes. The most common choice of the loss function is quadratic, resulting in the mean squared error
Mean squared error
In statistics, the mean squared error of an estimator is one of many ways to quantify the difference between values implied by a kernel density estimator and the true values of the quantity being estimated. MSE is a risk function, corresponding to the expected value of the squared error loss or...
criterion of optimality.
Finite-sample efficiency
Suppose } is a parametric modelParametric model
In statistics, a parametric model or parametric family or finite-dimensional model is a family of distributions that can be described using a finite number of parameters...
and is the data sampled from this model. Let be the estimator
Estimator
In statistics, an estimator is a rule for calculating an estimate of a given quantity based on observed data: thus the rule and its result are distinguished....
for the parameter θ. If this estimator is unbiased
Bias of an estimator
In statistics, bias of an estimator is the difference between this estimator's expected value and the true value of the parameter being estimated. An estimator or decision rule with zero bias is called unbiased. Otherwise the estimator is said to be biased.In ordinary English, the term bias is...
(that is), then the celebrated Cramér–Rao inequality states the variance
Variance
In probability theory and statistics, the variance is a measure of how far a set of numbers is spread out. It is one of several descriptors of a probability distribution, describing how far the numbers lie from the mean . In particular, the variance is one of the moments of a distribution...
of this estimator is bounded from below:
-
whereis the Fisher information matrix of the model at point θ. Generally, the variance measures the degree of dispersion of a random variable around its mean. Thus estimators with small variances are more concentrated, they estimate the parameters more precisely. We say that the estimator is finite-sample efficient estimator (in the class of unbiased estimators) if it reaches the lower bound in the Cramér–Rao inequality above, for all . Efficient estimators are always minimum variance unbiased estimator, however the opposite is not true: a minimum variance unbiased estimator may be inefficient.
Historically, the finite-sample efficiency was the first optimality notion introduced, and it is still sometimes encountered in old textbooks or introductory statistics courses, mainly because the Cramér–Rao inequality is easy to understand and to derive. However there are several drawbacks with this definition, which does not allow the concept of finite-sample efficiency to maintain popularity:- Finite-sample efficient estimators are extremely rare. In fact, it was proved that efficient estimation is possible only in an exponential familyExponential familyIn probability and statistics, an exponential family is an important class of probability distributions sharing a certain form, specified below. This special form is chosen for mathematical convenience, on account of some useful algebraic properties, as well as for generality, as exponential...
, and only for the natural parameters of that family. - This notion of efficiency is restricted to the class of unbiasedBias of an estimatorIn statistics, bias of an estimator is the difference between this estimator's expected value and the true value of the parameter being estimated. An estimator or decision rule with zero bias is called unbiased. Otherwise the estimator is said to be biased.In ordinary English, the term bias is...
estimators. Since there are no good theoretical reasons to require that estimators are unbiased, this restriction is inconvenient. In fact, if we use mean squared errorMean squared errorIn statistics, the mean squared error of an estimator is one of many ways to quantify the difference between values implied by a kernel density estimator and the true values of the quantity being estimated. MSE is a risk function, corresponding to the expected value of the squared error loss or...
as a selection criterion, many biased estimators will slightly outperform the “best” unbiased ones. For example, the James–Stein estimator is known to outperform some unbiased estimators. - Finite-sample efficiency is based on the variance, as a criterion according to which the estimators are judged. A more general approach is to use loss functionLoss functionIn statistics and decision theory a loss function is a function that maps an event onto a real number intuitively representing some "cost" associated with the event. Typically it is used for parameter estimation, and the event in question is some function of the difference between estimated and...
s other than quadratic ones, in which case the finite-sample efficiency can no longer be formulated.
Example
Among the models encountered in practice, efficient estimators exist for: the mean μ of the normal distribution (but not the variance σ2), parameter λ of the Poisson distributionPoisson distributionIn probability theory and statistics, the Poisson distribution is a discrete probability distribution that expresses the probability of a given number of events occurring in a fixed interval of time and/or space if these events occur with a known average rate and independently of the time since...
, the probability p in the binomial or multinomial distribution.
Consider the model of a normal distribution with unknown mean but known variance: The data consists of n iid observations from this model: . We estimate the parameter θ using the sample mean of all observations:-
This estimator has mean θ and variance of , which is equal to the reciprocal of the Fisher informationFisher informationIn mathematical statistics and information theory, the Fisher information is the variance of the score. In Bayesian statistics, the asymptotic distribution of the posterior mode depends on the Fisher information and not on the prior...
from the sample. Thus, the sample mean is a finite-sample efficient estimator for the mean of the normal distribution.
Relative efficiency
Ifand
are estimators for the parameter
, then
is said to dominate
Dominating decision ruleIn decision theory, a decision rule is said to dominate another if the performance of the former is sometimes better, and never worse, than that of the latter....
if:
- its mean squared errorMean squared errorIn statistics, the mean squared error of an estimator is one of many ways to quantify the difference between values implied by a kernel density estimator and the true values of the quantity being estimated. MSE is a risk function, corresponding to the expected value of the squared error loss or...
(MSE) is smaller for at least some value of - the MSE does not exceed that of
for any value of θ.
Formally,dominates
if
holds for all, with strict inequality holding somewhere.
The relative efficiency is defined as
Althoughis in general a function of
, in many cases the dependence drops out; if this is so,
being greater than one would indicate that
is preferable, whatever the true value of
.
Asymptotic efficiency
For some estimatorEstimatorIn statistics, an estimator is a rule for calculating an estimate of a given quantity based on observed data: thus the rule and its result are distinguished....
s, they can attain efficiency asymptotically and are thus called asymptotically efficient estimators.
This can be the case for some maximum likelihoodMaximum likelihoodIn statistics, maximum-likelihood estimation is a method of estimating the parameters of a statistical model. When applied to a data set and given a statistical model, maximum-likelihood estimation provides estimates for the model's parameters....
estimators or for any estimators that attain equality of the Cramér-Rao bound asymptotically.
See also
- Hodges–Lehmann efficiency
- Pitman efficiency
- Bayes estimatorBayes estimatorIn estimation theory and decision theory, a Bayes estimator or a Bayes action is an estimator or decision rule that minimizes the posterior expected value of a loss function . Equivalently, it maximizes the posterior expectation of a utility function...
- Hodges’ estimatorHodges’ estimatorIn statistics, Hodges’ estimator is a famous counter example of an estimator which is "superefficient", i.e. it attains smaller asymptotic variance than regular efficient estimators...
- its mean squared error
- Finite-sample efficient estimators are extremely rare. In fact, it was proved that efficient estimation is possible only in an exponential family