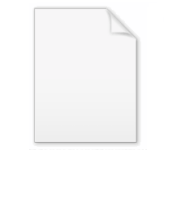
Fisher information
Encyclopedia
In mathematical statistics
and information theory
, the Fisher information (sometimes simply called information) is the variance
of the score
. In Bayesian statistics
, the asymptotic distribution of the posterior mode
depends on the Fisher information and not on the prior (according to the Bernstein–von Mises theorem
, which was anticipated by Laplace for exponential families). The role of the Fisher information in the asymptotic theory of maximum-likelihood estimation was emphasized by the statistician R.A. Fisher
(following some initial results by F. Y. Edgeworth
). The Fisher information is also used in the calculation of the Jeffreys prior
, which is used in Bayesian statistics.
The Fisher-information matrix is used to calculate the covariance matrices associated with maximum-likelihood estimates. It can also be used in the formulation of test statistics, such as the Wald test
.
. For example, Savage says: "In it [Fisher information], he [Fisher] was to some extent anticipated (Edgeworth 1908–9 esp. 502, 507–8, 662, 677–8, 82–5 and references he [Edgeworth] cites including Pearson and Filon 1898 [. . .])."
There are a number of early historical sources
and a number of reviews of this early work.
that an observable random variable
X carries about an unknown parameter
θ upon which the probability of X depends. The probability function for X, which is also the likelihood function
for θ, is a function ƒ(X; θ); it is the probability mass
(or probability density
) of the random variable X conditional on the value of θ. The partial derivative with respect to θ of the natural logarithm
of the likelihood function is called the score
. Under certain regularity conditions, it can be shown that the first moment
of the score is 0. The second moment is called the Fisher information:

where, for any given value of θ, the expression E[…|θ] denotes the conditional expectation
over values for X with respect to the probability function ƒ(x; θ) given θ. Note that
. A random variable carrying high Fisher information implies that the absolute value of the score is often high. The Fisher information is not a function of a particular observation, as the random variable X has been averaged out.
Since the expectation
of the score
is zero, the Fisher information is also the variance
of the score.
If is twice differentiable with respect to θ, and under certain regularity conditions, then the Fisher information may also be written as
Thus, the Fisher information is the negative of the expectation of the second derivative
with respect to θ of the natural logarithm
of f. Information may be seen to be a measure of the "curvature" of the support curve
near the maximum likelihood estimate
of θ. A "blunt" support curve (one with a shallow maximum) would have a low negative expected second derivative, and thus low information; while a sharp one would have a high negative expected second derivative and thus high information.
Information is additive, in that the information yielded by two independent
experiments is the sum of the information from each experiment separately:

This result follows from the elementary fact that if random variables are independent, the variance of their sum is the sum of their variances.
Hence the information in a random sample of size n is n times that in a sample of size 1 (if observations are independent).
The information provided by a sufficient statistic
is the same as that of the sample X. This may be seen by using Neyman's factorization criterion for a sufficient statistic. If T(X) is sufficient for θ, then

for some functions g and h. See sufficient statistic
for a more detailed explanation. The equality of information then follows from the following fact:

which follows from the definition of Fisher information, and the independence of h(X) from θ. More generally, if is a statistic
, then

with equality if and only if
T is a sufficient statistic.
Consider an unbiased estimator
. Mathematically, we write

The likelihood function
ƒ(X; θ) describes the probability that we observe a given sample x given a known value of θ. If ƒ is sharply peaked with respect to changes in θ, it is easy to intuit the "correct" value of θ given the data, and hence the data contains a lot of information about the parameter. If the likelihood ƒ is flat and spread-out, then it would take many, many samples of X to estimate the actual "true" value of θ. Therefore, we would intuit that the data contain much less information about the parameter.
Now, we differentiate the unbiased-ness condition above to get

We now make use of two facts. The first is that the likelihood ƒ is just the probability of the data given the parameter. Since it is a probability, it must be normalized, implying that

Second, we know from basic calculus that

Using these two facts in the above let us write

Factoring the integrand gives

If we square the equation, the Cauchy–Schwarz inequality
lets us write

The right-most factor is defined to be the Fisher Information
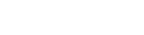
The left-most factor is the expected mean-squared error of the estimator θ, since

Notice that the inequality tells us that, fundamentally,
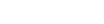
In other words, the precision to which we can estimate θ is fundamentally limited by the Fisher Information of the likelihood function.
is a random variable with two possible outcomes, "success" and "failure", with "success" having a probability of θ. The outcome can be thought of as determined by a coin toss, with the probability of obtaining a "head" being θ and the probability of obtaining a "tail" being .
The Fisher information contained in n independent Bernoulli trial
s may be calculated as follows. In the following, A represents the number of successes, B the number of failures, and is the total number of trials.

Mathematical statistics
Mathematical statistics is the study of statistics from a mathematical standpoint, using probability theory as well as other branches of mathematics such as linear algebra and analysis...
and information theory
Information theory
Information theory is a branch of applied mathematics and electrical engineering involving the quantification of information. Information theory was developed by Claude E. Shannon to find fundamental limits on signal processing operations such as compressing data and on reliably storing and...
, the Fisher information (sometimes simply called information) is the variance
Variance
In probability theory and statistics, the variance is a measure of how far a set of numbers is spread out. It is one of several descriptors of a probability distribution, describing how far the numbers lie from the mean . In particular, the variance is one of the moments of a distribution...
of the score
Score (statistics)
In statistics, the score, score function, efficient score or informant plays an important role in several aspects of inference...
. In Bayesian statistics
Bayesian statistics
Bayesian statistics is that subset of the entire field of statistics in which the evidence about the true state of the world is expressed in terms of degrees of belief or, more specifically, Bayesian probabilities...
, the asymptotic distribution of the posterior mode
Mode (statistics)
In statistics, the mode is the value that occurs most frequently in a data set or a probability distribution. In some fields, notably education, sample data are often called scores, and the sample mode is known as the modal score....
depends on the Fisher information and not on the prior (according to the Bernstein–von Mises theorem
Bernstein–von Mises theorem
In Bayesian inference, the Bernstein–von Mises theorem provides the basis for the important result that the posterior distribution for unknown quantities in any problem is effectively independent of the prior distribution once the amount of information supplied by a sample of data is large...
, which was anticipated by Laplace for exponential families). The role of the Fisher information in the asymptotic theory of maximum-likelihood estimation was emphasized by the statistician R.A. Fisher
Ronald Fisher
Sir Ronald Aylmer Fisher FRS was an English statistician, evolutionary biologist, eugenicist and geneticist. Among other things, Fisher is well known for his contributions to statistics by creating Fisher's exact test and Fisher's equation...
(following some initial results by F. Y. Edgeworth
Francis Ysidro Edgeworth
Francis Ysidro Edgeworth FBA was an Irish philosopher and political economist who made significant contributions to the methods of statistics during the 1880s...
). The Fisher information is also used in the calculation of the Jeffreys prior
Jeffreys prior
In Bayesian probability, the Jeffreys prior, named after Harold Jeffreys, is a non-informative prior distribution on parameter space that is proportional to the square root of the determinant of the Fisher information:...
, which is used in Bayesian statistics.
The Fisher-information matrix is used to calculate the covariance matrices associated with maximum-likelihood estimates. It can also be used in the formulation of test statistics, such as the Wald test
Wald test
The Wald test is a parametric statistical test named after Abraham Wald with a great variety of uses. Whenever a relationship within or between data items can be expressed as a statistical model with parameters to be estimated from a sample, the Wald test can be used to test the true value of the...
.
History
The Fisher information was discussed by several early statisticians, notably F. Y. EdgeworthFrancis Ysidro Edgeworth
Francis Ysidro Edgeworth FBA was an Irish philosopher and political economist who made significant contributions to the methods of statistics during the 1880s...
. For example, Savage says: "In it [Fisher information], he [Fisher] was to some extent anticipated (Edgeworth 1908–9 esp. 502, 507–8, 662, 677–8, 82–5 and references he [Edgeworth] cites including Pearson and Filon 1898 [. . .])."
There are a number of early historical sources
and a number of reviews of this early work.
Definition
The Fisher information is a way of measuring the amount of informationInformation
Information in its most restricted technical sense is a message or collection of messages that consists of an ordered sequence of symbols, or it is the meaning that can be interpreted from such a message or collection of messages. Information can be recorded or transmitted. It can be recorded as...
that an observable random variable
Random variable
In probability and statistics, a random variable or stochastic variable is, roughly speaking, a variable whose value results from a measurement on some type of random process. Formally, it is a function from a probability space, typically to the real numbers, which is measurable functionmeasurable...
X carries about an unknown parameter
Parameter
Parameter from Ancient Greek παρά also “para” meaning “beside, subsidiary” and μέτρον also “metron” meaning “measure”, can be interpreted in mathematics, logic, linguistics, environmental science and other disciplines....
θ upon which the probability of X depends. The probability function for X, which is also the likelihood function
Likelihood function
In statistics, a likelihood function is a function of the parameters of a statistical model, defined as follows: the likelihood of a set of parameter values given some observed outcomes is equal to the probability of those observed outcomes given those parameter values...
for θ, is a function ƒ(X; θ); it is the probability mass
Probability mass function
In probability theory and statistics, a probability mass function is a function that gives the probability that a discrete random variable is exactly equal to some value...
(or probability density
Probability density function
In probability theory, a probability density function , or density of a continuous random variable is a function that describes the relative likelihood for this random variable to occur at a given point. The probability for the random variable to fall within a particular region is given by the...
) of the random variable X conditional on the value of θ. The partial derivative with respect to θ of the natural logarithm
Natural logarithm
The natural logarithm is the logarithm to the base e, where e is an irrational and transcendental constant approximately equal to 2.718281828...
of the likelihood function is called the score
Score (statistics)
In statistics, the score, score function, efficient score or informant plays an important role in several aspects of inference...
. Under certain regularity conditions, it can be shown that the first moment
Moment (mathematics)
In mathematics, a moment is, loosely speaking, a quantitative measure of the shape of a set of points. The "second moment", for example, is widely used and measures the "width" of a set of points in one dimension or in higher dimensions measures the shape of a cloud of points as it could be fit by...
of the score is 0. The second moment is called the Fisher information:

where, for any given value of θ, the expression E[…|θ] denotes the conditional expectation
Expected value
In probability theory, the expected value of a random variable is the weighted average of all possible values that this random variable can take on...
over values for X with respect to the probability function ƒ(x; θ) given θ. Note that

Since the expectation
Expected value
In probability theory, the expected value of a random variable is the weighted average of all possible values that this random variable can take on...
of the score
Score (statistics)
In statistics, the score, score function, efficient score or informant plays an important role in several aspects of inference...
is zero, the Fisher information is also the variance
Variance
In probability theory and statistics, the variance is a measure of how far a set of numbers is spread out. It is one of several descriptors of a probability distribution, describing how far the numbers lie from the mean . In particular, the variance is one of the moments of a distribution...
of the score.
If is twice differentiable with respect to θ, and under certain regularity conditions, then the Fisher information may also be written as

Thus, the Fisher information is the negative of the expectation of the second derivative
Derivative
In calculus, a branch of mathematics, the derivative is a measure of how a function changes as its input changes. Loosely speaking, a derivative can be thought of as how much one quantity is changing in response to changes in some other quantity; for example, the derivative of the position of a...
with respect to θ of the natural logarithm
Natural logarithm
The natural logarithm is the logarithm to the base e, where e is an irrational and transcendental constant approximately equal to 2.718281828...
of f. Information may be seen to be a measure of the "curvature" of the support curve
Support curve
Support curve is a statistical term, coined by A. W. F. Edwards, to describe the graph of the natural logarithm of the likelihood function. The function being plotted is used in the computation of the score and Fisher information, and the graph has a direct interpretation in the context of maximum...
near the maximum likelihood estimate
Maximum likelihood
In statistics, maximum-likelihood estimation is a method of estimating the parameters of a statistical model. When applied to a data set and given a statistical model, maximum-likelihood estimation provides estimates for the model's parameters....
of θ. A "blunt" support curve (one with a shallow maximum) would have a low negative expected second derivative, and thus low information; while a sharp one would have a high negative expected second derivative and thus high information.
Information is additive, in that the information yielded by two independent
Statistical independence
In probability theory, to say that two events are independent intuitively means that the occurrence of one event makes it neither more nor less probable that the other occurs...
experiments is the sum of the information from each experiment separately:

This result follows from the elementary fact that if random variables are independent, the variance of their sum is the sum of their variances.
Hence the information in a random sample of size n is n times that in a sample of size 1 (if observations are independent).
The information provided by a sufficient statistic
Sufficiency (statistics)
In statistics, a sufficient statistic is a statistic which has the property of sufficiency with respect to a statistical model and its associated unknown parameter, meaning that "no other statistic which can be calculated from the same sample provides any additional information as to the value of...
is the same as that of the sample X. This may be seen by using Neyman's factorization criterion for a sufficient statistic. If T(X) is sufficient for θ, then

for some functions g and h. See sufficient statistic
Sufficiency (statistics)
In statistics, a sufficient statistic is a statistic which has the property of sufficiency with respect to a statistical model and its associated unknown parameter, meaning that "no other statistic which can be calculated from the same sample provides any additional information as to the value of...
for a more detailed explanation. The equality of information then follows from the following fact:

which follows from the definition of Fisher information, and the independence of h(X) from θ. More generally, if is a statistic
Statistic
A statistic is a single measure of some attribute of a sample . It is calculated by applying a function to the values of the items comprising the sample which are known together as a set of data.More formally, statistical theory defines a statistic as a function of a sample where the function...
, then

with equality if and only if
If and only if
In logic and related fields such as mathematics and philosophy, if and only if is a biconditional logical connective between statements....
T is a sufficient statistic.
Informal derivation of the Cramér–Rao bound
The Cramér–Rao bound states that the inverse of the Fisher information is a lower bound on the variance of any unbiased estimator of θ. Van Trees (1968) and Frieden (2004) provide the following method of deriving the Cramér–Rao bound, a result which describes use of the Fisher information, informally:Consider an unbiased estimator


The likelihood function
Likelihood function
In statistics, a likelihood function is a function of the parameters of a statistical model, defined as follows: the likelihood of a set of parameter values given some observed outcomes is equal to the probability of those observed outcomes given those parameter values...
ƒ(X; θ) describes the probability that we observe a given sample x given a known value of θ. If ƒ is sharply peaked with respect to changes in θ, it is easy to intuit the "correct" value of θ given the data, and hence the data contains a lot of information about the parameter. If the likelihood ƒ is flat and spread-out, then it would take many, many samples of X to estimate the actual "true" value of θ. Therefore, we would intuit that the data contain much less information about the parameter.
Now, we differentiate the unbiased-ness condition above to get

We now make use of two facts. The first is that the likelihood ƒ is just the probability of the data given the parameter. Since it is a probability, it must be normalized, implying that

Second, we know from basic calculus that

Using these two facts in the above let us write

Factoring the integrand gives

If we square the equation, the Cauchy–Schwarz inequality
Cauchy–Schwarz inequality
In mathematics, the Cauchy–Schwarz inequality , is a useful inequality encountered in many different settings, such as linear algebra, analysis, probability theory, and other areas...
lets us write

The right-most factor is defined to be the Fisher Information
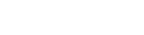
The left-most factor is the expected mean-squared error of the estimator θ, since

Notice that the inequality tells us that, fundamentally,
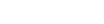
In other words, the precision to which we can estimate θ is fundamentally limited by the Fisher Information of the likelihood function.
Single-parameter Bernoulli experiment
A Bernoulli trialBernoulli trial
In the theory of probability and statistics, a Bernoulli trial is an experiment whose outcome is random and can be either of two possible outcomes, "success" and "failure"....
is a random variable with two possible outcomes, "success" and "failure", with "success" having a probability of θ. The outcome can be thought of as determined by a coin toss, with the probability of obtaining a "head" being θ and the probability of obtaining a "tail" being .
The Fisher information contained in n independent Bernoulli trial
Bernoulli trial
In the theory of probability and statistics, a Bernoulli trial is an experiment whose outcome is random and can be either of two possible outcomes, "success" and "failure"....
s may be calculated as follows. In the following, A represents the number of successes, B the number of failures, and is the total number of trials.

-
-
-
(on differentiating log x, see natural logarithm
Natural logarithmThe natural logarithm is the logarithm to the base e, where e is an irrational and transcendental constant approximately equal to 2.718281828...
)
-
-
(as the expected value of A given θ is nθ, etc.)
(1) defines Fisher information.
(2) invokes the fact that the information in a sufficient statistic is the same as that of the sample itself.
(3) expands the natural logarithmNatural logarithmThe natural logarithm is the logarithm to the base e, where e is an irrational and transcendental constant approximately equal to 2.718281828...
term and drops a constant.
(4) and (5) differentiate with respect to θ.
(6) replaces A and B with their expectations. (7) is algebra.
The end result, namely,
is the reciprocal of the varianceVarianceIn probability theory and statistics, the variance is a measure of how far a set of numbers is spread out. It is one of several descriptors of a probability distribution, describing how far the numbers lie from the mean . In particular, the variance is one of the moments of a distribution...
of the mean number of successes in n Bernoulli trialBernoulli trialIn the theory of probability and statistics, a Bernoulli trial is an experiment whose outcome is random and can be either of two possible outcomes, "success" and "failure"....
s, as expected (see last sentence of the preceding section).
Matrix form
When there are N parameters, so that θ is a Nx1 vectorthen the Fisher information takes the form of an NxN matrix
Matrix (mathematics)In mathematics, a matrix is a rectangular array of numbers, symbols, or expressions. The individual items in a matrix are called its elements or entries. An example of a matrix with six elements isMatrices of the same size can be added or subtracted element by element...
, the Fisher Information Matrix (FIM), with typical element:
The FIM is a NxN positive semidefinite symmetric matrix, defining a Riemannian metric on the N-dimensionDimensionIn physics and mathematics, the dimension of a space or object is informally defined as the minimum number of coordinates needed to specify any point within it. Thus a line has a dimension of one because only one coordinate is needed to specify a point on it...
al parameter spaceParameter spaceIn science, a parameter space is the set of values of parameters encountered in a particular mathematical model. Often the parameters are inputs of a function, in which case the technical term for the parameter space is domain of a function....
, thus connecting Fisher information to differential geometry. In that context, this metric is known as the Fisher information metricFisher information metricIn information geometry, the Fisher information metric is a particular Riemannian metric which can be defined on a smooth statistical manifold, i.e., a smooth manifold whose points are probability measures defined on a common probability space....
, and the topic is called information geometryInformation geometryInformation geometry is a branch of mathematics that applies the techniques of differential geometry to the field of probability theory. It derives its name from the fact that the Fisher information is used as the Riemannian metric when considering the geometry of probability distribution families...
.
Under certain regularity conditions, the Fisher Information Matrix may also be written as:
Orthogonal parameters
We say that two parameters θi and θj are orthogonal if the element of the ith row and jth column of the Fisher information matrix is zero. Orthogonal parameters are easy to deal with in the sense that their maximum likelihood estimatesMaximum likelihoodIn statistics, maximum-likelihood estimation is a method of estimating the parameters of a statistical model. When applied to a data set and given a statistical model, maximum-likelihood estimation provides estimates for the model's parameters....
are independent and can be calculated separately. When dealing with research problems, it is very common for the researcher to invest some time searching for an orthogonal parametrization of the densities involved in the problem.
Multivariate normal distribution
The FIM for a N-variate multivariate normal distribution has a special form. Letand let Σ(θ) be the covariance matrix
Covariance matrixIn probability theory and statistics, a covariance matrix is a matrix whose element in the i, j position is the covariance between the i th and j th elements of a random vector...
. Then the typical element, 0 ≤ m, n < M, of the FIM for is:
wheredenotes the transpose
TransposeIn linear algebra, the transpose of a matrix A is another matrix AT created by any one of the following equivalent actions:...
of a vector, tr(..) denotes the trace of a square matrix, and:
Note that a special, but very common, case is the one where
, a constant. Then
In this case the Fisher information matrix may be identified with the coefficient matrix of the normal equations of least squaresLeast squaresThe method of least squares is a standard approach to the approximate solution of overdetermined systems, i.e., sets of equations in which there are more equations than unknowns. "Least squares" means that the overall solution minimizes the sum of the squares of the errors made in solving every...
estimation theory.
Reparametrization
The Fisher information depends on the parametrization of the problem. If θ and η are two scalar parametrizations of an estimation problem, and θ is a continuously differentiable function of η, then
whereand
are the Fisher information measures of η and θ, respectively.
In the vector case, supposeand
are k-vectors which parametrize an estimation problem, and suppose that
is a continuously differentiable function of
, then,
where the (i, j)th element of the k × k Jacobian matrixis defined by
and whereis the matrix transpose of
.
In information geometryInformation geometryInformation geometry is a branch of mathematics that applies the techniques of differential geometry to the field of probability theory. It derives its name from the fact that the Fisher information is used as the Riemannian metric when considering the geometry of probability distribution families...
, this is seen as a change of coordinates on a Riemannian manifoldRiemannian manifoldIn Riemannian geometry and the differential geometry of surfaces, a Riemannian manifold or Riemannian space is a real differentiable manifold M in which each tangent space is equipped with an inner product g, a Riemannian metric, which varies smoothly from point to point...
, and the intrinsic properties of curvature are unchanged under different parametrization.
Optimal design of experiments
Fisher information is widely used in optimal experimental designOptimal designOptimal designs are a class of experimental designs that are optimal with respect to some statistical criterion.In the design of experiments for estimating statistical models, optimal designs allow parameters to be estimated without bias and with minimum-variance...
. Because of the reciprocity of estimator-variance and Fisher information, minimizing the variance corresponds to maximizing the information.
When the linearLinear modelIn statistics, the term linear model is used in different ways according to the context. The most common occurrence is in connection with regression models and the term is often taken as synonymous with linear regression model. However the term is also used in time series analysis with a different...
(or linearizedNonlinear regressionIn statistics, nonlinear regression is a form of regression analysis in which observational data are modeled by a function which is a nonlinear combination of the model parameters and depends on one or more independent variables...
) statistical modelStatistical modelA statistical model is a formalization of relationships between variables in the form of mathematical equations. A statistical model describes how one or more random variables are related to one or more random variables. The model is statistical as the variables are not deterministically but...
has several parameterParameterParameter from Ancient Greek παρά also “para” meaning “beside, subsidiary” and μέτρον also “metron” meaning “measure”, can be interpreted in mathematics, logic, linguistics, environmental science and other disciplines....
s, the meanExpected valueIn probability theory, the expected value of a random variable is the weighted average of all possible values that this random variable can take on...
of the parameter-estimator is a vector and its varianceCovariance matrixIn probability theory and statistics, a covariance matrix is a matrix whose element in the i, j position is the covariance between the i th and j th elements of a random vector...
is a matrixMatrix (mathematics)In mathematics, a matrix is a rectangular array of numbers, symbols, or expressions. The individual items in a matrix are called its elements or entries. An example of a matrix with six elements isMatrices of the same size can be added or subtracted element by element...
. The inverse matrix of the variance-matrix is called the "information matrix". Because the variance of the estimator of a parameter vector is a matrix, the problem of "minimizing the variance" is complicated. Using statistical theoryStatistical theoryThe theory of statistics provides a basis for the whole range of techniques, in both study design and data analysis, that are used within applications of statistics. The theory covers approaches to statistical-decision problems and to statistical inference, and the actions and deductions that...
, statisticians compress the information-matrix using real-valued summary statisticsSummary statisticsIn descriptive statistics, summary statistics are used to summarize a set of observations, in order to communicate the largest amount as simply as possible...
; being real-valued functions, these "information criteria" can be maximized.
Traditionally, statisticians have evaluated estimators and designs by considering some summary statisticSummary statisticsIn descriptive statistics, summary statistics are used to summarize a set of observations, in order to communicate the largest amount as simply as possible...
of the covariance matrix (of a meanExpected valueIn probability theory, the expected value of a random variable is the weighted average of all possible values that this random variable can take on...
-unbiased estimatorEstimatorIn statistics, an estimator is a rule for calculating an estimate of a given quantity based on observed data: thus the rule and its result are distinguished....
), usually with positive real values (like the determinantDeterminantIn linear algebra, the determinant is a value associated with a square matrix. It can be computed from the entries of the matrix by a specific arithmetic expression, while other ways to determine its value exist as well...
or matrix trace). Working with positive real-numbers brings several advantages: If the estimator of a single parameter has a positive variance, then the variance and the Fisher information are both positive real numbers; hence they are members of the convex cone of nonnegative real numbers (whose nonzero members have reciprocals in this same cone).
For several parameters, the covariance-matrices and information-matrices are elements of the convex cone of nonnegative-definite symmetric matrices in a partially ordered vector spaceOrdered vector spaceIn mathematics an ordered vector space or partially ordered vector space is a vector space equipped with a partial order which is compatible with the vector space operations.- Definition:...
, under the LoewnerCharles LoewnerCharles Loewner was an American mathematician. His name was Karel Löwner in Czech and Karl Löwner in German.Loewner received his Ph.D...
(Löwner) order. This cone is closed under matrix-matrix addition, under matrix-inversion, and under the multiplication of positive real-numbers and matrices. An exposition of matrix theory and the Loewner-order appears in Pukelsheim.
The traditional optimality-criteria are the informationInformationInformation in its most restricted technical sense is a message or collection of messages that consists of an ordered sequence of symbols, or it is the meaning that can be interpreted from such a message or collection of messages. Information can be recorded or transmitted. It can be recorded as...
-matrix's invariantsInvariant theoryInvariant theory is a branch of abstract algebra dealing with actions of groups on algebraic varieties from the point of view of their effect on functions...
; algebraically, the traditional optimality-criteria are functionalsFunctional (mathematics)In mathematics, and particularly in functional analysis, a functional is a map from a vector space into its underlying scalar field. In other words, it is a function that takes a vector as its input argument, and returns a scalar...
of the eigenvalues of the (Fisher) information matrix: see optimal designOptimal designOptimal designs are a class of experimental designs that are optimal with respect to some statistical criterion.In the design of experiments for estimating statistical models, optimal designs allow parameters to be estimated without bias and with minimum-variance...
.
Jeffreys prior in Bayesian statistics
In Bayesian statisticsBayesian statisticsBayesian statistics is that subset of the entire field of statistics in which the evidence about the true state of the world is expressed in terms of degrees of belief or, more specifically, Bayesian probabilities...
, the Fisher information is used to calculate the Jeffreys priorJeffreys priorIn Bayesian probability, the Jeffreys prior, named after Harold Jeffreys, is a non-informative prior distribution on parameter space that is proportional to the square root of the determinant of the Fisher information:...
, which is a standard, non-informative prior for continuous distribution parameters.
Relation to KL-divergence
Fisher information is the curvature (second derivative) of the Kullback–Leibler divergenceKullback–Leibler divergenceIn probability theory and information theory, the Kullback–Leibler divergence is a non-symmetric measure of the difference between two probability distributions P and Q...
of the distributionfrom the true distribution
. Here,
is the true value of the parameter
and derivatives are taken with respect to
.
Distinction from the Hessian of the entropy
In general, the Fisher Information
is not the same as the negative of the second derivative of the entropy
For instance, with, the entropy H is independent of the distribution mean θ. Thus, in this case, the second derivative of the entropy is zero. However, for the Fisher information, we have
See also
- Observed informationObserved informationIn statistics, the observed information, or observed Fisher information, is the negative of the second derivative of the "log-likelihood"...
- Fisher information metricFisher information metricIn information geometry, the Fisher information metric is a particular Riemannian metric which can be defined on a smooth statistical manifold, i.e., a smooth manifold whose points are probability measures defined on a common probability space....
- Formation matrixFormation matrixIn statistics and information theory, the expected formation matrix of a likelihood function L is the matrix inverse of the Fisher information matrix of L, while the observed formation matrix of L is the inverse of the observed information matrix of L.Currently, no notation for dealing with...
- Information geometryInformation geometryInformation geometry is a branch of mathematics that applies the techniques of differential geometry to the field of probability theory. It derives its name from the fact that the Fisher information is used as the Riemannian metric when considering the geometry of probability distribution families...
- Jeffreys priorJeffreys priorIn Bayesian probability, the Jeffreys prior, named after Harold Jeffreys, is a non-informative prior distribution on parameter space that is proportional to the square root of the determinant of the Fisher information:...
- Cramér–Rao bound
Other measures employed in information theoryInformation theoryInformation theory is a branch of applied mathematics and electrical engineering involving the quantification of information. Information theory was developed by Claude E. Shannon to find fundamental limits on signal processing operations such as compressing data and on reliably storing and...
:- Entropy (information theory)
- Kullback–Leibler divergenceKullback–Leibler divergenceIn probability theory and information theory, the Kullback–Leibler divergence is a non-symmetric measure of the difference between two probability distributions P and Q...
- Self-informationSelf-informationIn information theory, self-information is a measure of the information content associated with the outcome of a random variable. It is expressed in a unit of information, for example bits,nats,or...
External links
- Fisher4Cast: a Matlab, GUI-based Fisher information tool for research and teaching, primarily aimed at cosmological forecasting applications.
- Observed information
-
-
-
-
-