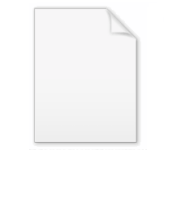
Part-of-speech tagging
Encyclopedia
In corpus linguistics
, part-of-speech tagging (POS tagging or POST), also called grammatical
tagging or word-category
disambiguation, is the process of marking up a word in a text (corpus) as corresponding to a particular part of speech, based on both its definition, as well as its context—i.e. relationship with adjacent and related words
in a phrase
, sentence
, or paragraph
.
A simplified form of this is commonly taught to school-age children, in the identification of words as noun
s, verb
s, adjective
s, adverb
s, etc.
Once performed by hand, POS tagging is now done in the context of computational linguistics
, using algorithms which associate discrete terms, as well as hidden parts of speech, in accordance with a set of descriptive tags. POS-tagging algorithms fall into two distinctive groups: rule-based and stochastic. E.Brill's tagger
, one of the first and widely used English POS-taggers, employs rule-based algorithms.
s (as opposed to many artificial language
s), a large percentage of word-forms are ambiguous. For example, even "dogs", which is usually thought of as just a plural noun, can also be a verb:
Performing grammatical tagging will indicate that "dogs" is a verb, and not the more common plural noun, since one of the words must be the main verb, and the noun reading is less likely following "sailor" (sailor !
→
dogs). Semantic analysis can then extrapolate that "sailor" and "barmaid" implicate "dogs" as 1) in the nautical context (sailor→←barmaid) and 2) an action applied to the object "barmaid" ([subject] dogs→barmaid). In this context, "dogs" is a nautical
term meaning "fastens (a watertight barmaid) securely; applies a dog
to".
"Dogged", on the other hand, can be either an adjective or a past-tense verb. Just which parts of speech a word can represent varies greatly.
Native speakers of a language perform grammatical and semantic analysis innately, and thus trained linguists can identify the grammatical parts of speech to various fine degrees depending on the tagging system. Schools commonly teach that there are 9 parts of speech in English: noun
, verb
, article
, adjective
, preposition, pronoun
, adverb
, conjunction
, and interjection
. However, there are clearly many more categories and sub-categories. For nouns, plural, possessive, and singular forms can be distinguished. In many languages words are also marked for their "case
" (role as subject, object, etc.), grammatical gender
, and so on; while verbs are marked for tense
, aspect
, and other things.
In part-of-speech tagging by computer, it is typical to distinguish from 50 to 150 separate parts of speech for English, for example, NN for singular common nouns, NNS for plural common nouns, NP for singular proper nouns (see the POS tags used in the Brown Corpus). Work on stochastic
methods for tagging Koine Greek
(DeRose 1990) has used over 1,000 parts of speech, and found that about as many words were ambiguous there as in English. A morphosyntactic descriptor in the case of morphologically rich languages can be expressed like Ncmsan, which means Category=Noun, Type = common, Gender = masculine, Number = singular, Case = accusative, Animate = no.
. The first major corpus of English for computer analysis was the Brown Corpus
developed at Brown University
by Henry Kucera
and Nelson Francis, in the mid-1960s. It consists of about 1,000,000 words of running English prose text, made up of 500 samples from randomly chosen publications. Each sample is 2,000 or more words (ending at the first sentence-end after 2,000 words, so that the corpus contains only complete sentences).
The Brown Corpus
was painstakingly "tagged" with part-of-speech markers over many years. A first approximation was done with a program by Greene and Rubin, which consisted of a huge handmade list of what categories could co-occur at all. For example, article then noun can occur, but article verb (arguably) cannot. The program got about 70% correct. Its results were repeatedly reviewed and corrected by hand, and later users sent in errata, so that by the late 70s the tagging was nearly perfect (allowing for some cases on which even human speakers might not agree).
This corpus has been used for innumerable studies of word-frequency and of part-of-speech, and inspired the development of similar "tagged" corpora in many other languages. Statistics derived by analyzing it formed the basis for most later part-of-speech tagging systems, such as CLAWS (linguistics) and VOLSUNGA. However, by this time (2005) it has been superseded by larger corpora such as the 100 million word British National Corpus
.
For some time, part-of-speech tagging was considered an inseparable part of natural language processing
, because there are certain cases where the correct part of speech cannot be decided without understanding the semantics
or even the pragmatics
of the context. This is extremely expensive, especially because analyzing the higher levels is much harder when multiple part-of-speech possibilities must be considered for each word.
s (HMMs) to disambiguate parts of speech, when working to tag the Lancaster-Oslo-Bergen Corpus
of British English. HMMs involve counting cases (such as from the Brown Corpus), and making a table of the probabilities of certain sequences. For example, once you've seen an article such as 'the', perhaps the next word is a noun 40% of the time, an adjective 40%, and a number 20%. Knowing this, a program can decide that "can" in "the can" is far more likely to be a noun than a verb or a modal. The same method can of course be used to benefit from knowledge about following words.
More advanced ("higher order") HMMs learn the probabilities not only of pairs, but triples or even larger sequences. So, for example, if you've just seen an article and a verb, the next item may be very likely a preposition, article, or noun, but much less likely another verb.
When several ambiguous words occur together, the possibilities multiply. However, it is easy to enumerate every combination and to assign a relative probability to each one, by multiplying together the probabilities of each choice in turn. The combination with highest probability is then chosen. The European group developed CLAWS, a tagging program that did exactly this, and achieved accuracy in the 93-95% range.
It is worth remembering, as Eugene Charniak
points out in Statistical techniques for natural language parsing http://www.cs.brown.edu/people/ec/home.html, that merely assigning the most common tag to each known word and the tag "proper noun
" to all unknowns, will approach 90% accuracy because many words are unambiguous.
CLAWS pioneered the field of HMM-based part of speech tagging, but was quite expensive since it enumerated all possibilities. It sometimes had to resort to backup methods when there were simply too many (the Brown Corpus
contains a case with 17 ambiguous words in a row, and there are words such as "still" that can represent as many as 7 distinct parts of speech).
HMMs underlie the functioning of stochastic taggers and are used in various algorithms one of the most widely used being the bi-directional inference algorithm .
and Ken Church independently developed dynamic programming
algorithms to solve the same problem in vastly less time. Their methods were similar to the Viterbi algorithm
known for some time in other fields. DeRose used a table of pairs, while Church used a table of triples and an ingenious method of estimating the values for triples that were rare or nonexistent in the Brown Corpus (actual measurement of triple probabilities would require a much larger corpus). Both methods achieved accuracy over 95%. DeRose's 1990 dissertation at Brown University
included analyses of the specific error types, probabilities, and other related data, and replicated his work for Greek, where it proved similarly effective.
These findings were surprisingly disruptive to the field of natural language processing. The accuracy reported was higher than the typical accuracy of very sophisticated algorithms that integrated part of speech choice with many higher levels of linguistic analysis: syntax, morphology, semantics, and so on. CLAWS, DeRose's and Church's methods did fail for some of the known cases where semantics is required, but those proved negligibly rare. This convinced many in the field that part-of-speech tagging could usefully be separated out from the other levels of processing; this in turn simplified the theory and practice of computerized language analysis, and encouraged researchers to find ways to separate out other pieces as well. Markov Models are now the standard method for part-of-speech assignment.
using "unsupervised" tagging. Unsupervised tagging techniques use an untagged corpus for their training data and produce the tagset by induction. That is, they observe patterns in word use, and derive part-of-speech categories themselves. For example, statistics readily reveal that "the", "a", and "an" occur in similar contexts, while "eat" occurs in very different ones. With sufficient iteration, similarity classes of words emerge that are remarkably similar to those human linguists would expect; and the differences themselves sometimes suggest valuable new insights.
These two categories can be further subdivided into rule-based, stochastic, and neural approaches.
, Brill Tagger
, Constraint Grammar
, and the Baum-Welch algorithm
(also known as the forward-backward algorithm). Hidden Markov model
and visible Markov model
taggers can both be implemented using the Viterbi algorithm
. The Brill tagger in unusual in that it learns a set of patterns, and then applies those patterns rather than optimizing a statistical quantity.
Many machine learning
methods have also been applied to the problem of POS tagging. Methods such as SVM
, Maximum entropy classifier, Perceptron
, and Nearest-neighbor have all been tried, and most can achieve accuracy above 95%.
A direct comparison of several methods is reported (with references) at http://aclweb.org/aclwiki/index.php?title=POS_Tagging_%28State_of_the_art%29. This comparison uses the Penn tag set on some of the Penn Treebank data, so the results are directly comparable.
However, many significant taggers are not included (perhaps because of the labor involved in reconfiguring them for this particular dataset). Thus, it should not be assumed that the results reported there are the best that can be achieved with a given approach; nor even the best that have been achieved with a given approach.
the big green fire truck
A second important example is the use/mention distinction, as in the following example, where "blue" is clearly not functioning as an adjective (the Brown Corpus tag set appends the suffix "-NC" in such cases):
the word "blue" has 4 letters.
Words in a language other than that of the "main" text, are commonly tagged as "foreign", usually in addition to a tag for the role the foreign word is actually playing in context.
There are also many cases where POS categories and "words" do not map one to one, for example:
David's
gonna
don't
vice versa
first-cut
cannot
pre- and post-secondary
look (a word) up
In the last example, "look" and "up" arguably function as a single verbal unit, despite the possibility of other words coming between them. Some tag sets (such as Penn) break hyphenated words, contractions, and possessives into separate tokens, thus avoiding some but far from all such problems.
It is unclear whether it is best to treat words such as "be", "have", and "do" as categories in their own right (as in the Brown Corpus), or as simply verbs (as in the LOB Corpus and the Penn Treebank
). "be" has more forms than other English verbs, and occurs in quite different grammatical contexts, complicating the issue.
The most popular "tag set" for POS tagging for American English is probably the Penn tag set, developed in the Penn Treebank project. It is largely similar to the earlier Brown Corpus and LOB Corpus tag sets, though much smaller. In Europe, tag sets from the Eagles Guidelines
see wide use, and include versions for multiple languages.
POS tagging work has been done in a variety of languages, and the set of POS tags used varies greatly with language. Tags usually are designed to include overt morphological distinctions (this makes the tag sets for heavily inflected languages such as Greek
and Latin
very large; and makes tagging words in agglutinative languages
such an Inuit
virtually impossible. However, Petrov, D. Das, and R. McDonald ("A Universal Part-of-Speech Tagset" http://arxiv.org/abs/1104.2086) have proposed a "universal" tag set, with 12 categories (for example, no subtypes of nouns, verbs, punctuation, etc; no distinction of "to" as an infinitive marker vs. preposition, etc). Whether a very small set of very broad tags, or a much larger set of more precise ones, is preferable, depends on the purpose at hand. Automatic tagging is easier on smaller tag-sets.
A different issue is that some cases are in fact ambiguous. Beatrice Santorini gives examples in "Part-of-speech Tagging Guidelines for the Penn Treebank Project," (3rd rev, June 1990 [ftp://ftp.cis.upenn.edu/pub/treebank/doc/tagguide.ps.gz]), including the following (p. 32) case in which entertaining can function either as an adjective or a verb, and there is no evident way to decide:
The Duchess was entertaining last night.
Corpus linguistics
Corpus linguistics is the study of language as expressed in samples or "real world" text. This method represents a digestive approach to deriving a set of abstract rules by which a natural language is governed or else relates to another language. Originally done by hand, corpora are now largely...
, part-of-speech tagging (POS tagging or POST), also called grammatical
Grammar
In linguistics, grammar is the set of structural rules that govern the composition of clauses, phrases, and words in any given natural language. The term refers also to the study of such rules, and this field includes morphology, syntax, and phonology, often complemented by phonetics, semantics,...
tagging or word-category
Lexical category
In grammar, a part of speech is a linguistic category of words , which is generally defined by the syntactic or morphological behaviour of the lexical item in question. Common linguistic categories include noun and verb, among others...
disambiguation, is the process of marking up a word in a text (corpus) as corresponding to a particular part of speech, based on both its definition, as well as its context—i.e. relationship with adjacent and related words
Lexicography
Lexicography is divided into two related disciplines:*Practical lexicography is the art or craft of compiling, writing and editing dictionaries....
in a phrase
Phrase
In everyday speech, a phrase may refer to any group of words. In linguistics, a phrase is a group of words which form a constituent and so function as a single unit in the syntax of a sentence. A phrase is lower on the grammatical hierarchy than a clause....
, sentence
Sentence (linguistics)
In the field of linguistics, a sentence is an expression in natural language, and often defined to indicate a grammatical unit consisting of one or more words that generally bear minimal syntactic relation to the words that precede or follow it...
, or paragraph
Paragraph
A paragraph is a self-contained unit of a discourse in writing dealing with a particular point or idea. A paragraph consists of one or more sentences. The start of a paragraph is indicated by beginning on a new line. Sometimes the first line is indented...
.
A simplified form of this is commonly taught to school-age children, in the identification of words as noun
Noun
In linguistics, a noun is a member of a large, open lexical category whose members can occur as the main word in the subject of a clause, the object of a verb, or the object of a preposition .Lexical categories are defined in terms of how their members combine with other kinds of...
s, verb
Verb
A verb, from the Latin verbum meaning word, is a word that in syntax conveys an action , or a state of being . In the usual description of English, the basic form, with or without the particle to, is the infinitive...
s, adjective
Adjective
In grammar, an adjective is a 'describing' word; the main syntactic role of which is to qualify a noun or noun phrase, giving more information about the object signified....
s, adverb
Adverb
An adverb is a part of speech that modifies verbs or any part of speech other than a noun . Adverbs can modify verbs, adjectives , clauses, sentences, and other adverbs....
s, etc.
Once performed by hand, POS tagging is now done in the context of computational linguistics
Computational linguistics
Computational linguistics is an interdisciplinary field dealing with the statistical or rule-based modeling of natural language from a computational perspective....
, using algorithms which associate discrete terms, as well as hidden parts of speech, in accordance with a set of descriptive tags. POS-tagging algorithms fall into two distinctive groups: rule-based and stochastic. E.Brill's tagger
Brill Tagger
The Brill tagger is a method for doing part-of-speech tagging. It was described by Eric Brill in his 1993 PhD thesis . It can be summarized as an "error-driven transformation-based tagger". It is...
, one of the first and widely used English POS-taggers, employs rule-based algorithms.
Principle
Part-of-speech tagging is harder than just having a list of words and their parts of speech, because some words can represent more than one part of speech at different times, and because some parts of speech are complex or unspoken. This is not rare—in natural languageNatural language
In the philosophy of language, a natural language is any language which arises in an unpremeditated fashion as the result of the innate facility for language possessed by the human intellect. A natural language is typically used for communication, and may be spoken, signed, or written...
s (as opposed to many artificial language
Constructed language
A planned or constructed language—known colloquially as a conlang—is a language whose phonology, grammar, and/or vocabulary has been consciously devised by an individual or group, instead of having evolved naturally...
s), a large percentage of word-forms are ambiguous. For example, even "dogs", which is usually thought of as just a plural noun, can also be a verb:
- The sailor dogs the barmaid.
Performing grammatical tagging will indicate that "dogs" is a verb, and not the more common plural noun, since one of the words must be the main verb, and the noun reading is less likely following "sailor" (sailor !
Negation
In logic and mathematics, negation, also called logical complement, is an operation on propositions, truth values, or semantic values more generally. Intuitively, the negation of a proposition is true when that proposition is false, and vice versa. In classical logic negation is normally identified...
→
Logical conjunction
In logic and mathematics, a two-place logical operator and, also known as logical conjunction, results in true if both of its operands are true, otherwise the value of false....
dogs). Semantic analysis can then extrapolate that "sailor" and "barmaid" implicate "dogs" as 1) in the nautical context (sailor→
Seamanship
Seamanship is the art of operating a ship or boat.It involves a knowledge of a variety of topics and development of specialised skills including: navigation and international maritime law; weather, meteorology and forecasting; watchstanding; ship-handling and small boat handling; operation of deck...
term meaning "fastens (a watertight barmaid) securely; applies a dog
Dog (engineering)
In engineering a dog is a tool that prevents movement or imparts movement by offering physical obstruction or engagement of some kind. It may hold another object in place by blocking it, clamping it, or otherwise obstructing its movement...
to".
"Dogged", on the other hand, can be either an adjective or a past-tense verb. Just which parts of speech a word can represent varies greatly.
Native speakers of a language perform grammatical and semantic analysis innately, and thus trained linguists can identify the grammatical parts of speech to various fine degrees depending on the tagging system. Schools commonly teach that there are 9 parts of speech in English: noun
Noun
In linguistics, a noun is a member of a large, open lexical category whose members can occur as the main word in the subject of a clause, the object of a verb, or the object of a preposition .Lexical categories are defined in terms of how their members combine with other kinds of...
, verb
Verb
A verb, from the Latin verbum meaning word, is a word that in syntax conveys an action , or a state of being . In the usual description of English, the basic form, with or without the particle to, is the infinitive...
, article
Article (grammar)
An article is a word that combines with a noun to indicate the type of reference being made by the noun. Articles specify the grammatical definiteness of the noun, in some languages extending to volume or numerical scope. The articles in the English language are the and a/an, and some...
, adjective
Adjective
In grammar, an adjective is a 'describing' word; the main syntactic role of which is to qualify a noun or noun phrase, giving more information about the object signified....
, preposition, pronoun
Pronoun
In linguistics and grammar, a pronoun is a pro-form that substitutes for a noun , such as, in English, the words it and he...
, adverb
Adverb
An adverb is a part of speech that modifies verbs or any part of speech other than a noun . Adverbs can modify verbs, adjectives , clauses, sentences, and other adverbs....
, conjunction
Grammatical conjunction
In grammar, a conjunction is a part of speech that connects two words, sentences, phrases or clauses together. A discourse connective is a conjunction joining sentences. This definition may overlap with that of other parts of speech, so what constitutes a "conjunction" must be defined for each...
, and interjection
Interjection
In grammar, an interjection or exclamation is a word used to express an emotion or sentiment on the part of the speaker . Filled pauses such as uh, er, um are also considered interjections...
. However, there are clearly many more categories and sub-categories. For nouns, plural, possessive, and singular forms can be distinguished. In many languages words are also marked for their "case
Grammatical case
In grammar, the case of a noun or pronoun is an inflectional form that indicates its grammatical function in a phrase, clause, or sentence. For example, a pronoun may play the role of subject , of direct object , or of possessor...
" (role as subject, object, etc.), grammatical gender
Grammatical gender
Grammatical gender is defined linguistically as a system of classes of nouns which trigger specific types of inflections in associated words, such as adjectives, verbs and others. For a system of noun classes to be a gender system, every noun must belong to one of the classes and there should be...
, and so on; while verbs are marked for tense
Grammatical tense
A tense is a grammatical category that locates a situation in time, to indicate when the situation takes place.Bernard Comrie, Aspect, 1976:6:...
, aspect
Grammatical aspect
In linguistics, the grammatical aspect of a verb is a grammatical category that defines the temporal flow in a given action, event, or state, from the point of view of the speaker...
, and other things.
In part-of-speech tagging by computer, it is typical to distinguish from 50 to 150 separate parts of speech for English, for example, NN for singular common nouns, NNS for plural common nouns, NP for singular proper nouns (see the POS tags used in the Brown Corpus). Work on stochastic
Stochastic
Stochastic refers to systems whose behaviour is intrinsically non-deterministic. A stochastic process is one whose behavior is non-deterministic, in that a system's subsequent state is determined both by the process's predictable actions and by a random element. However, according to M. Kac and E...
methods for tagging Koine Greek
Koine Greek
Koine Greek is the universal dialect of the Greek language spoken throughout post-Classical antiquity , developing from the Attic dialect, with admixture of elements especially from Ionic....
(DeRose 1990) has used over 1,000 parts of speech, and found that about as many words were ambiguous there as in English. A morphosyntactic descriptor in the case of morphologically rich languages can be expressed like Ncmsan, which means Category=Noun, Type = common, Gender = masculine, Number = singular, Case = accusative, Animate = no.
The Brown Corpus
Research on part-of-speech tagging has been closely tied to corpus linguisticsCorpus linguistics
Corpus linguistics is the study of language as expressed in samples or "real world" text. This method represents a digestive approach to deriving a set of abstract rules by which a natural language is governed or else relates to another language. Originally done by hand, corpora are now largely...
. The first major corpus of English for computer analysis was the Brown Corpus
Brown Corpus
The Brown University Standard Corpus of Present-Day American English was compiled in the 1960s by Henry Kucera and W. Nelson Francis at Brown University, Providence, Rhode Island as a general corpus in the field of corpus linguistics...
developed at Brown University
Brown University
Brown University is a private, Ivy League university located in Providence, Rhode Island, United States. Founded in 1764 prior to American independence from the British Empire as the College in the English Colony of Rhode Island and Providence Plantations early in the reign of King George III ,...
by Henry Kucera
Henry Kucera
Henry Kučera, born Jindřich Kučera was a Czech linguist who was a pioneer in corpus linguistics and linguistic software....
and Nelson Francis, in the mid-1960s. It consists of about 1,000,000 words of running English prose text, made up of 500 samples from randomly chosen publications. Each sample is 2,000 or more words (ending at the first sentence-end after 2,000 words, so that the corpus contains only complete sentences).
The Brown Corpus
Brown Corpus
The Brown University Standard Corpus of Present-Day American English was compiled in the 1960s by Henry Kucera and W. Nelson Francis at Brown University, Providence, Rhode Island as a general corpus in the field of corpus linguistics...
was painstakingly "tagged" with part-of-speech markers over many years. A first approximation was done with a program by Greene and Rubin, which consisted of a huge handmade list of what categories could co-occur at all. For example, article then noun can occur, but article verb (arguably) cannot. The program got about 70% correct. Its results were repeatedly reviewed and corrected by hand, and later users sent in errata, so that by the late 70s the tagging was nearly perfect (allowing for some cases on which even human speakers might not agree).
This corpus has been used for innumerable studies of word-frequency and of part-of-speech, and inspired the development of similar "tagged" corpora in many other languages. Statistics derived by analyzing it formed the basis for most later part-of-speech tagging systems, such as CLAWS (linguistics) and VOLSUNGA. However, by this time (2005) it has been superseded by larger corpora such as the 100 million word British National Corpus
British National Corpus
The British National Corpus is a 100-million-word text corpus of samples of written and spoken English from a wide range of sources. It was compiled as a general corpus in the field of corpus linguistics...
.
For some time, part-of-speech tagging was considered an inseparable part of natural language processing
Natural language processing
Natural language processing is a field of computer science and linguistics concerned with the interactions between computers and human languages; it began as a branch of artificial intelligence....
, because there are certain cases where the correct part of speech cannot be decided without understanding the semantics
Semantics
Semantics is the study of meaning. It focuses on the relation between signifiers, such as words, phrases, signs and symbols, and what they stand for, their denotata....
or even the pragmatics
Pragmatics
Pragmatics is a subfield of linguistics which studies the ways in which context contributes to meaning. Pragmatics encompasses speech act theory, conversational implicature, talk in interaction and other approaches to language behavior in philosophy, sociology, and linguistics. It studies how the...
of the context. This is extremely expensive, especially because analyzing the higher levels is much harder when multiple part-of-speech possibilities must be considered for each word.
Use of Hidden Markov Models
In the mid 1980s, researchers in Europe began to use hidden Markov modelHidden Markov model
A hidden Markov model is a statistical Markov model in which the system being modeled is assumed to be a Markov process with unobserved states. An HMM can be considered as the simplest dynamic Bayesian network. The mathematics behind the HMM was developed by L. E...
s (HMMs) to disambiguate parts of speech, when working to tag the Lancaster-Oslo-Bergen Corpus
Lancaster-Oslo-Bergen Corpus
The Lancaster-Oslo-Bergen Corpus was compiled in 1980s in collaboration between the University of Lancaster, the University of Oslo, and the Norwegian Computing Centre for the Humanities, Bergen, to provide a British counterpart to the Brown Corpus compiled by Kucera and Francis for American...
of British English. HMMs involve counting cases (such as from the Brown Corpus), and making a table of the probabilities of certain sequences. For example, once you've seen an article such as 'the', perhaps the next word is a noun 40% of the time, an adjective 40%, and a number 20%. Knowing this, a program can decide that "can" in "the can" is far more likely to be a noun than a verb or a modal. The same method can of course be used to benefit from knowledge about following words.
More advanced ("higher order") HMMs learn the probabilities not only of pairs, but triples or even larger sequences. So, for example, if you've just seen an article and a verb, the next item may be very likely a preposition, article, or noun, but much less likely another verb.
When several ambiguous words occur together, the possibilities multiply. However, it is easy to enumerate every combination and to assign a relative probability to each one, by multiplying together the probabilities of each choice in turn. The combination with highest probability is then chosen. The European group developed CLAWS, a tagging program that did exactly this, and achieved accuracy in the 93-95% range.
It is worth remembering, as Eugene Charniak
Eugene Charniak
Eugene Charniak is a Computer Science and Cognitive Science professor at Brown University. He has an A.B. in Physics from The University of Chicago and a Ph.D. from M.I.T. in Computer Science. His research has always been in the area of language understanding or technologies which relate to it,...
points out in Statistical techniques for natural language parsing http://www.cs.brown.edu/people/ec/home.html, that merely assigning the most common tag to each known word and the tag "proper noun
Proper noun
A proper noun or proper name is a noun representing a unique entity , as distinguished from a common noun, which represents a class of entities —for example, city, planet, person or corporation)...
" to all unknowns, will approach 90% accuracy because many words are unambiguous.
CLAWS pioneered the field of HMM-based part of speech tagging, but was quite expensive since it enumerated all possibilities. It sometimes had to resort to backup methods when there were simply too many (the Brown Corpus
Brown Corpus
The Brown University Standard Corpus of Present-Day American English was compiled in the 1960s by Henry Kucera and W. Nelson Francis at Brown University, Providence, Rhode Island as a general corpus in the field of corpus linguistics...
contains a case with 17 ambiguous words in a row, and there are words such as "still" that can represent as many as 7 distinct parts of speech).
HMMs underlie the functioning of stochastic taggers and are used in various algorithms one of the most widely used being the bi-directional inference algorithm .
Dynamic Programming methods
In 1987, Steven DeRoseSteven DeRose
Steven J DeRose is a computer scientist with a significant history of contributions to Computational Linguistics and to key standards related to document processing, mostly around ISO's Standard Generalized Markup Language and W3C's Extensible Markup Language .His contributions include the...
and Ken Church independently developed dynamic programming
Dynamic programming
In mathematics and computer science, dynamic programming is a method for solving complex problems by breaking them down into simpler subproblems. It is applicable to problems exhibiting the properties of overlapping subproblems which are only slightly smaller and optimal substructure...
algorithms to solve the same problem in vastly less time. Their methods were similar to the Viterbi algorithm
Viterbi algorithm
The Viterbi algorithm is a dynamic programming algorithm for finding the most likely sequence of hidden states – called the Viterbi path – that results in a sequence of observed events, especially in the context of Markov information sources, and more generally, hidden Markov models...
known for some time in other fields. DeRose used a table of pairs, while Church used a table of triples and an ingenious method of estimating the values for triples that were rare or nonexistent in the Brown Corpus (actual measurement of triple probabilities would require a much larger corpus). Both methods achieved accuracy over 95%. DeRose's 1990 dissertation at Brown University
Brown University
Brown University is a private, Ivy League university located in Providence, Rhode Island, United States. Founded in 1764 prior to American independence from the British Empire as the College in the English Colony of Rhode Island and Providence Plantations early in the reign of King George III ,...
included analyses of the specific error types, probabilities, and other related data, and replicated his work for Greek, where it proved similarly effective.
These findings were surprisingly disruptive to the field of natural language processing. The accuracy reported was higher than the typical accuracy of very sophisticated algorithms that integrated part of speech choice with many higher levels of linguistic analysis: syntax, morphology, semantics, and so on. CLAWS, DeRose's and Church's methods did fail for some of the known cases where semantics is required, but those proved negligibly rare. This convinced many in the field that part-of-speech tagging could usefully be separated out from the other levels of processing; this in turn simplified the theory and practice of computerized language analysis, and encouraged researchers to find ways to separate out other pieces as well. Markov Models are now the standard method for part-of-speech assignment.
Unsupervised taggers
The methods already discussed involve working from a pre-existing corpus to learn tag probabilities. It is, however, also possible to bootstrapBootstrapping (linguistics)
In psycholinguistics, bootstrapping refers to the question of how language acquisition "gets started." A child gradually acquires a great deal of interlocking knowledge about the structure and vocabulary of his or her language...
using "unsupervised" tagging. Unsupervised tagging techniques use an untagged corpus for their training data and produce the tagset by induction. That is, they observe patterns in word use, and derive part-of-speech categories themselves. For example, statistics readily reveal that "the", "a", and "an" occur in similar contexts, while "eat" occurs in very different ones. With sufficient iteration, similarity classes of words emerge that are remarkably similar to those human linguists would expect; and the differences themselves sometimes suggest valuable new insights.
These two categories can be further subdivided into rule-based, stochastic, and neural approaches.
Other taggers and methods
Some current major algorithms for part-of-speech tagging include the Viterbi algorithmViterbi algorithm
The Viterbi algorithm is a dynamic programming algorithm for finding the most likely sequence of hidden states – called the Viterbi path – that results in a sequence of observed events, especially in the context of Markov information sources, and more generally, hidden Markov models...
, Brill Tagger
Brill Tagger
The Brill tagger is a method for doing part-of-speech tagging. It was described by Eric Brill in his 1993 PhD thesis . It can be summarized as an "error-driven transformation-based tagger". It is...
, Constraint Grammar
Constraint Grammar
Constraint Grammar is a methodological paradigm for Natural language processing . Linguist-written, context dependent rules are compiled into a grammar that assigns grammatical tags to words or other tokens in running text...
, and the Baum-Welch algorithm
Baum-Welch algorithm
In electrical engineering, computer science, statistical computing and bioinformatics, the Baum–Welch algorithm is used to find the unknown parameters of a hidden Markov model . It makes use of the forward-backward algorithm and is named for Leonard E. Baum and Lloyd R...
(also known as the forward-backward algorithm). Hidden Markov model
Hidden Markov model
A hidden Markov model is a statistical Markov model in which the system being modeled is assumed to be a Markov process with unobserved states. An HMM can be considered as the simplest dynamic Bayesian network. The mathematics behind the HMM was developed by L. E...
and visible Markov model
Markov model
In probability theory, a Markov model is a stochastic model that assumes the Markov property. Generally, this assumption enables reasoning and computation with the model that would otherwise be intractable.-Introduction:...
taggers can both be implemented using the Viterbi algorithm
Viterbi algorithm
The Viterbi algorithm is a dynamic programming algorithm for finding the most likely sequence of hidden states – called the Viterbi path – that results in a sequence of observed events, especially in the context of Markov information sources, and more generally, hidden Markov models...
. The Brill tagger in unusual in that it learns a set of patterns, and then applies those patterns rather than optimizing a statistical quantity.
Many machine learning
Machine learning
Machine learning, a branch of artificial intelligence, is a scientific discipline concerned with the design and development of algorithms that allow computers to evolve behaviors based on empirical data, such as from sensor data or databases...
methods have also been applied to the problem of POS tagging. Methods such as SVM
SVM
SVM can refer to:* SVM * Saskatchewan Volunteer Medal* Scanning voltage microscopy* Schuylkill Valley Metro* Secure Virtual Machine, or AMD Virtualization , a virtualization technology by AMD* Solaris Volume Manager...
, Maximum entropy classifier, Perceptron
Perceptron
The perceptron is a type of artificial neural network invented in 1957 at the Cornell Aeronautical Laboratory by Frank Rosenblatt. It can be seen as the simplest kind of feedforward neural network: a linear classifier.- Definition :...
, and Nearest-neighbor have all been tried, and most can achieve accuracy above 95%.
A direct comparison of several methods is reported (with references) at http://aclweb.org/aclwiki/index.php?title=POS_Tagging_%28State_of_the_art%29. This comparison uses the Penn tag set on some of the Penn Treebank data, so the results are directly comparable.
However, many significant taggers are not included (perhaps because of the labor involved in reconfiguring them for this particular dataset). Thus, it should not be assumed that the results reported there are the best that can be achieved with a given approach; nor even the best that have been achieved with a given approach.
Issues
While there is broad agreement about basic categories, a number of edge cases make it difficult to settle on a single "correct" set of tags, even in a single language such as English. For example, it is hard to say whether "fire" is functioning as an adjective or a noun inthe big green fire truck
A second important example is the use/mention distinction, as in the following example, where "blue" is clearly not functioning as an adjective (the Brown Corpus tag set appends the suffix "-NC" in such cases):
the word "blue" has 4 letters.
Words in a language other than that of the "main" text, are commonly tagged as "foreign", usually in addition to a tag for the role the foreign word is actually playing in context.
There are also many cases where POS categories and "words" do not map one to one, for example:
David's
gonna
don't
vice versa
first-cut
cannot
pre- and post-secondary
look (a word) up
In the last example, "look" and "up" arguably function as a single verbal unit, despite the possibility of other words coming between them. Some tag sets (such as Penn) break hyphenated words, contractions, and possessives into separate tokens, thus avoiding some but far from all such problems.
It is unclear whether it is best to treat words such as "be", "have", and "do" as categories in their own right (as in the Brown Corpus), or as simply verbs (as in the LOB Corpus and the Penn Treebank
Treebank
A treebank or parsed corpus is a text corpus in which each sentence has been parsed, i.e. annotated with syntactic structure. Syntactic structure is commonly represented as a tree structure, hence the name Treebank...
). "be" has more forms than other English verbs, and occurs in quite different grammatical contexts, complicating the issue.
The most popular "tag set" for POS tagging for American English is probably the Penn tag set, developed in the Penn Treebank project. It is largely similar to the earlier Brown Corpus and LOB Corpus tag sets, though much smaller. In Europe, tag sets from the Eagles Guidelines
Eagles Guidelines
The Eagles Guidelines provide guidance for markup to be used with text corpora, particularly for identifying features relevant in computational linguistics and lexicography.From :...
see wide use, and include versions for multiple languages.
POS tagging work has been done in a variety of languages, and the set of POS tags used varies greatly with language. Tags usually are designed to include overt morphological distinctions (this makes the tag sets for heavily inflected languages such as Greek
Greek language
Greek is an independent branch of the Indo-European family of languages. Native to the southern Balkans, it has the longest documented history of any Indo-European language, spanning 34 centuries of written records. Its writing system has been the Greek alphabet for the majority of its history;...
and Latin
Latin
Latin is an Italic language originally spoken in Latium and Ancient Rome. It, along with most European languages, is a descendant of the ancient Proto-Indo-European language. Although it is considered a dead language, a number of scholars and members of the Christian clergy speak it fluently, and...
very large; and makes tagging words in agglutinative languages
Agglutinative language
An agglutinative language is a language that uses agglutination extensively: most words are formed by joining morphemes together. This term was introduced by Wilhelm von Humboldt in 1836 to classify languages from a morphological point of view...
such an Inuit
Inuit
The Inuit are a group of culturally similar indigenous peoples inhabiting the Arctic regions of Canada , Denmark , Russia and the United States . Inuit means “the people” in the Inuktitut language...
virtually impossible. However, Petrov, D. Das, and R. McDonald ("A Universal Part-of-Speech Tagset" http://arxiv.org/abs/1104.2086) have proposed a "universal" tag set, with 12 categories (for example, no subtypes of nouns, verbs, punctuation, etc; no distinction of "to" as an infinitive marker vs. preposition, etc). Whether a very small set of very broad tags, or a much larger set of more precise ones, is preferable, depends on the purpose at hand. Automatic tagging is easier on smaller tag-sets.
A different issue is that some cases are in fact ambiguous. Beatrice Santorini gives examples in "Part-of-speech Tagging Guidelines for the Penn Treebank Project," (3rd rev, June 1990 [ftp://ftp.cis.upenn.edu/pub/treebank/doc/tagguide.ps.gz]), including the following (p. 32) case in which entertaining can function either as an adjective or a verb, and there is no evident way to decide:
The Duchess was entertaining last night.
See also
- Semantic net
- Sliding window based part-of-speech taggingSliding window based part-of-speech taggingSliding window based part-of-speech tagging is used to part-of-speech tag a text.A high percentage of words in a natural language are words which out of context can be assigned more than one part of speech. The percentage of these ambiguous words is typically around 30%, although it depends greatly...
- Trigram taggerTrigram taggerA trigram tagger is a statistical part-of-speech tagger based on second order Markov models. It is trained on a text corpus as a method to predict the next word, taking the product of the probabilities of unigram, bigram and trigram...
- Word sense disambiguationWord sense disambiguationIn computational linguistics, word-sense disambiguation is an open problem of natural language processing, which governs the process of identifying which sense of a word is used in a sentence, when the word has multiple meanings...
External links
- Overview of available taggers
- Cypher A natural language transcoder that performs POS-tagging, morphological processing, lexical analysis, to produce RDFResource Description FrameworkThe Resource Description Framework is a family of World Wide Web Consortium specifications originally designed as a metadata data model...
and SPARQLSPARQLSPARQL is an RDF query language; its name is an acronym that stands for SPARQL Protocol and RDF Query Language. It was made a standard by the RDF Data Access Working Group of the World Wide Web Consortium, and considered as one of the key technologies of semantic web...
from natural language BROKEN LINK - Resources for Studying English Syntax Online
- CLAWS
- LingPipe Commercial Java natural language processing software including trainable part-of-speech taggers with first-best, n-best and per-tag confidence output.
- OpenNLP Tagger AL 2.0 Tagger based on maxent and perceptron classifiers
- CRFTagger Conditional Random Fields (CRFs) English POS Tagger
- JTextPro A Java-based Text Processing Toolkit
- Citar LGPL C++ Hidden Markov ModelHidden Markov modelA hidden Markov model is a statistical Markov model in which the system being modeled is assumed to be a Markov process with unobserved states. An HMM can be considered as the simplest dynamic Bayesian network. The mathematics behind the HMM was developed by L. E...
trigram POS tagger, a JavaJava (programming language)Java is a programming language originally developed by James Gosling at Sun Microsystems and released in 1995 as a core component of Sun Microsystems' Java platform. The language derives much of its syntax from C and C++ but has a simpler object model and fewer low-level facilities...
port named Jitar is also available - Ninja-PoST PHP port of GPoSTTL, based on Eric Brill's rule-based tagger
- ComplexityIntelligence, LLC Free and Commercial NLP Web Services for Part Of Speech Tagging (and Named Entity Recognition)
- Part-of-Speech tagging based on Soundex features
- FastTag - LGPL Java POS tagger based on Eric Brill's rule-based tagger
- jspos - LGPL Javascript port of FastTag
- Topia TermExtractor - Python implementation of the UPenn BioIE parts-of-speech algorithm