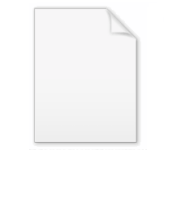
Kolmogorov-Smirnov test
Encyclopedia
In statistics
, the Kolmogorov
–Smirnov test (K–S test) is a nonparametric test for the equality of continuous, one-dimensional probability distribution
s that can be used to compare a sample
with a reference probability distribution (one-sample K–S test), or to compare two samples (two-sample K–S test). The Kolmogorov–Smirnov statistic quantifies a distance
between the empirical distribution function
of the sample and the cumulative distribution function
of the reference distribution, or between the empirical distribution functions of two samples. The null distribution
of this statistic is calculated under the null hypothesis
that the samples are drawn from the same distribution (in the two-sample case) or that the sample is drawn from the reference distribution (in the one-sample case). In each case, the distributions considered under the null hypothesis are continuous distributions but are otherwise unrestricted.
The two-sample KS test is one of the most useful and general nonparametric methods for comparing two samples, as it is sensitive to differences in both location and shape of the empirical cumulative distribution functions of the two samples.
The Kolmogorov–Smirnov test can be modified to serve as a goodness of fit
test. In the special case of testing for normality of the distribution, samples are standardized and compared with a standard normal distribution. This is equivalent to setting the mean and variance of the reference distribution equal to the sample estimates, and it is known that using these to define the specific reference distribution changes the null distribution of the test statistic: see below. Various studies have found that, even in this corrected form, the test is less powerful for testing normality than the Shapiro–Wilk test or Anderson–Darling test.
Fn for n iid
observations Xi is defined as

where
is the indicator function, equal to 1 if Xi ≤ x and equal to 0 otherwise.
The Kolmogorov–Smirnov statistic
for a given cumulative distribution function
F(x) is

where sup x is the supremum
of the set of distances. By the Glivenko–Cantelli theorem, if the sample comes from distribution F(x), then Dn converges to 0 almost surely
. Kolmogorov strengthened this result, by effectively providing the rate of this convergence (see below). The Donsker theorem provides yet a stronger result.
In practice, the statistic requires relatively large number of data to properly reject the null hypothesis.
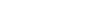
where B(t) is the Brownian bridge
. The cumulative distribution function
of K is given by

Both the form of the Kolmogorov–Smirnov test statistic and its asymptotic distribution under the null hypothesis were published by Andrey Kolmogorov
, while a table of the distribution was published by N.V. Smirnov. Recurrence relations for the distribution of the test statistic in finite samples are available.

in distribution
, where B(t) is the Brownian bridge
.
If F is continuous then under the null hypothesis
converges to the Kolmogorov distribution, which does not depend on F. This result may also be known as the Kolmogorov theorem; see Kolmogorov's theorem
for disambiguation.
The goodness-of-fit test or the Kolmogorov–Smirnov test is constructed by using the critical values of the Kolmogorov distribution. The null hypothesis is rejected at level
if

where Kα is found from

The asymptotic power
of this test is 1.
or other methods may be required, but tables have been prepared for some cases. Details for the required modifications to the test statistic and for the critical values for the normal distribution and the exponential distribution
have been published by Pearson & Hartley (1972, Table 54). Details for these distributions, with the addition of the Gumbel distribution, are also given by Shorak & Wellner (1986, p239). The Lilliefors test
represents a special case of this for the normal distribution.

where
and
are the empirical distribution function
s of the first and the second sample respectively.
The null hypothesis is rejected at level
if

Note that the two-sample test checks whether the two data samples come from the same distribution. This does not specify what that common
distribution is (e.g. normal or not normal).
. This is not straightforward because the maximum difference between two joint cumulative distribution function
s is not generally the same as the maximum difference of any of the complementary distribution functions. Thus the maximum difference will differ depending on which of
or
or any of the other two possible arrangements is used. One might require that the result of the test used should not depend on which choice is made.
One approach to generalizing the Kolmogorov–Smirnov statistic to higher dimensions which meets the above concern is to compare the cdfs of the two samples with all possible orderings, and take the largest of the set of resulting K-S statistics. In d dimensions, there are 2d−1 such orderings. One such variation is due to Peacock (1983) and another to Fasano & Franceschini (1987): see Lopes et al. (2007) for a comparison and computational details. Critical values for the test statistic can be obtained by simulations, but depend on the dependence structure in the joint distribution.
Statistics
Statistics is the study of the collection, organization, analysis, and interpretation of data. It deals with all aspects of this, including the planning of data collection in terms of the design of surveys and experiments....
, the Kolmogorov
Andrey Kolmogorov
Andrey Nikolaevich Kolmogorov was a Soviet mathematician, preeminent in the 20th century, who advanced various scientific fields, among them probability theory, topology, intuitionistic logic, turbulence, classical mechanics and computational complexity.-Early life:Kolmogorov was born at Tambov...
–Smirnov test (K–S test) is a nonparametric test for the equality of continuous, one-dimensional probability distribution
Probability distribution
In probability theory, a probability mass, probability density, or probability distribution is a function that describes the probability of a random variable taking certain values....
s that can be used to compare a sample
Random sample
In statistics, a sample is a subject chosen from a population for investigation; a random sample is one chosen by a method involving an unpredictable component...
with a reference probability distribution (one-sample K–S test), or to compare two samples (two-sample K–S test). The Kolmogorov–Smirnov statistic quantifies a distance
Metric (mathematics)
In mathematics, a metric or distance function is a function which defines a distance between elements of a set. A set with a metric is called a metric space. A metric induces a topology on a set but not all topologies can be generated by a metric...
between the empirical distribution function
Empirical distribution function
In statistics, the empirical distribution function, or empirical cdf, is the cumulative distribution function associated with the empirical measure of the sample. This cdf is a step function that jumps up by 1/n at each of the n data points. The empirical distribution function estimates the true...
of the sample and the cumulative distribution function
Cumulative distribution function
In probability theory and statistics, the cumulative distribution function , or just distribution function, describes the probability that a real-valued random variable X with a given probability distribution will be found at a value less than or equal to x. Intuitively, it is the "area so far"...
of the reference distribution, or between the empirical distribution functions of two samples. The null distribution
Null distribution
In statistical hypothesis testing, the null distribution is the probability distribution of the test statistic when the null hypothesis is true.In an F-test, the null distribution is an F-distribution....
of this statistic is calculated under the null hypothesis
Null hypothesis
The practice of science involves formulating and testing hypotheses, assertions that are capable of being proven false using a test of observed data. The null hypothesis typically corresponds to a general or default position...
that the samples are drawn from the same distribution (in the two-sample case) or that the sample is drawn from the reference distribution (in the one-sample case). In each case, the distributions considered under the null hypothesis are continuous distributions but are otherwise unrestricted.
The two-sample KS test is one of the most useful and general nonparametric methods for comparing two samples, as it is sensitive to differences in both location and shape of the empirical cumulative distribution functions of the two samples.
The Kolmogorov–Smirnov test can be modified to serve as a goodness of fit
Goodness of fit
The goodness of fit of a statistical model describes how well it fits a set of observations. Measures of goodness of fit typically summarize the discrepancy between observed values and the values expected under the model in question. Such measures can be used in statistical hypothesis testing, e.g...
test. In the special case of testing for normality of the distribution, samples are standardized and compared with a standard normal distribution. This is equivalent to setting the mean and variance of the reference distribution equal to the sample estimates, and it is known that using these to define the specific reference distribution changes the null distribution of the test statistic: see below. Various studies have found that, even in this corrected form, the test is less powerful for testing normality than the Shapiro–Wilk test or Anderson–Darling test.
Kolmogorov–Smirnov statistic
The empirical distribution functionEmpirical distribution function
In statistics, the empirical distribution function, or empirical cdf, is the cumulative distribution function associated with the empirical measure of the sample. This cdf is a step function that jumps up by 1/n at each of the n data points. The empirical distribution function estimates the true...
Fn for n iid
Independent and identically distributed random variables
In probability theory and statistics, a sequence or other collection of random variables is independent and identically distributed if each random variable has the same probability distribution as the others and all are mutually independent....
observations Xi is defined as

where

The Kolmogorov–Smirnov statistic
Statistic
A statistic is a single measure of some attribute of a sample . It is calculated by applying a function to the values of the items comprising the sample which are known together as a set of data.More formally, statistical theory defines a statistic as a function of a sample where the function...
for a given cumulative distribution function
Cumulative distribution function
In probability theory and statistics, the cumulative distribution function , or just distribution function, describes the probability that a real-valued random variable X with a given probability distribution will be found at a value less than or equal to x. Intuitively, it is the "area so far"...
F(x) is

where sup x is the supremum
Supremum
In mathematics, given a subset S of a totally or partially ordered set T, the supremum of S, if it exists, is the least element of T that is greater than or equal to every element of S. Consequently, the supremum is also referred to as the least upper bound . If the supremum exists, it is unique...
of the set of distances. By the Glivenko–Cantelli theorem, if the sample comes from distribution F(x), then Dn converges to 0 almost surely
Almost surely
In probability theory, one says that an event happens almost surely if it happens with probability one. The concept is analogous to the concept of "almost everywhere" in measure theory...
. Kolmogorov strengthened this result, by effectively providing the rate of this convergence (see below). The Donsker theorem provides yet a stronger result.
In practice, the statistic requires relatively large number of data to properly reject the null hypothesis.
Kolmogorov distribution
The Kolmogorov distribution is the distribution of the random variableRandom variable
In probability and statistics, a random variable or stochastic variable is, roughly speaking, a variable whose value results from a measurement on some type of random process. Formally, it is a function from a probability space, typically to the real numbers, which is measurable functionmeasurable...
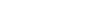
where B(t) is the Brownian bridge
Brownian bridge
A Brownian bridge is a continuous-time stochastic process B whose probability distribution is the conditional probability distribution of a Wiener process W given the condition that B = B = 0.The expected value of the bridge is zero, with variance t, implying that the most...
. The cumulative distribution function
Cumulative distribution function
In probability theory and statistics, the cumulative distribution function , or just distribution function, describes the probability that a real-valued random variable X with a given probability distribution will be found at a value less than or equal to x. Intuitively, it is the "area so far"...
of K is given by

Both the form of the Kolmogorov–Smirnov test statistic and its asymptotic distribution under the null hypothesis were published by Andrey Kolmogorov
Andrey Kolmogorov
Andrey Nikolaevich Kolmogorov was a Soviet mathematician, preeminent in the 20th century, who advanced various scientific fields, among them probability theory, topology, intuitionistic logic, turbulence, classical mechanics and computational complexity.-Early life:Kolmogorov was born at Tambov...
, while a table of the distribution was published by N.V. Smirnov. Recurrence relations for the distribution of the test statistic in finite samples are available.
Kolmogorov–Smirnov test
Under null hypothesis that the sample comes from the hypothesized distribution F(x),
in distribution
Convergence of random variables
In probability theory, there exist several different notions of convergence of random variables. The convergence of sequences of random variables to some limit random variable is an important concept in probability theory, and its applications to statistics and stochastic processes...
, where B(t) is the Brownian bridge
Brownian bridge
A Brownian bridge is a continuous-time stochastic process B whose probability distribution is the conditional probability distribution of a Wiener process W given the condition that B = B = 0.The expected value of the bridge is zero, with variance t, implying that the most...
.
If F is continuous then under the null hypothesis

Kolmogorov's theorem
Kolmogorov's theorem is any of several different results by Andrey Kolmogorov:In statistics* Kolmogorov–Smirnov testIn probability theory* Hahn–Kolmogorov theorem* Kolmogorov existence theorem* Kolmogorov continuity theorem...
for disambiguation.
The goodness-of-fit test or the Kolmogorov–Smirnov test is constructed by using the critical values of the Kolmogorov distribution. The null hypothesis is rejected at level


where Kα is found from

The asymptotic power
Statistical power
The power of a statistical test is the probability that the test will reject the null hypothesis when the null hypothesis is actually false . The power is in general a function of the possible distributions, often determined by a parameter, under the alternative hypothesis...
of this test is 1.
Test with estimated parameters
If either the form or the parameters of F(x) are determined from the data Xi the critical values determined in this way are invalid. In such cases, Monte CarloMonte Carlo method
Monte Carlo methods are a class of computational algorithms that rely on repeated random sampling to compute their results. Monte Carlo methods are often used in computer simulations of physical and mathematical systems...
or other methods may be required, but tables have been prepared for some cases. Details for the required modifications to the test statistic and for the critical values for the normal distribution and the exponential distribution
Exponential distribution
In probability theory and statistics, the exponential distribution is a family of continuous probability distributions. It describes the time between events in a Poisson process, i.e...
have been published by Pearson & Hartley (1972, Table 54). Details for these distributions, with the addition of the Gumbel distribution, are also given by Shorak & Wellner (1986, p239). The Lilliefors test
Lilliefors test
In statistics, the Lilliefors test, named after Hubert Lilliefors, professor of statistics at George Washington University, is an adaptation of the Kolmogorov–Smirnov test...
represents a special case of this for the normal distribution.
Two-sample Kolmogorov–Smirnov test
The Kolmogorov–Smirnov test may also be used to test whether two underlying one-dimensional probability distributions differ. In this case, the Kolmogorov–Smirnov statistic is
where


Empirical distribution function
In statistics, the empirical distribution function, or empirical cdf, is the cumulative distribution function associated with the empirical measure of the sample. This cdf is a step function that jumps up by 1/n at each of the n data points. The empirical distribution function estimates the true...
s of the first and the second sample respectively.
The null hypothesis is rejected at level


Note that the two-sample test checks whether the two data samples come from the same distribution. This does not specify what that common
distribution is (e.g. normal or not normal).
Setting confidence limits for the shape of a distribution function
While the Kolmogorov–Smirnov test is usually used to test whether a given F(x) is the underlying probability distribution of Fn(x), the procedure may be inverted to give confidence limits on F(x) itself. If one chooses a critical value of the test statistic Dα such that P(Dn > Dα) = α, then a band of width ±Dα around Fn(x) will entirely contain F(x) with probability 1 − α.The Kolmogorov–Smirnov statistic in more than one dimension
The Kolmogorov–Smirnov test statistic needs to be modified if a similar test is to be applied to multivariate dataMultivariate statistics
Multivariate statistics is a form of statistics encompassing the simultaneous observation and analysis of more than one statistical variable. The application of multivariate statistics is multivariate analysis...
. This is not straightforward because the maximum difference between two joint cumulative distribution function
Cumulative distribution function
In probability theory and statistics, the cumulative distribution function , or just distribution function, describes the probability that a real-valued random variable X with a given probability distribution will be found at a value less than or equal to x. Intuitively, it is the "area so far"...
s is not generally the same as the maximum difference of any of the complementary distribution functions. Thus the maximum difference will differ depending on which of


One approach to generalizing the Kolmogorov–Smirnov statistic to higher dimensions which meets the above concern is to compare the cdfs of the two samples with all possible orderings, and take the largest of the set of resulting K-S statistics. In d dimensions, there are 2d−1 such orderings. One such variation is due to Peacock (1983) and another to Fasano & Franceschini (1987): see Lopes et al. (2007) for a comparison and computational details. Critical values for the test statistic can be obtained by simulations, but depend on the dependence structure in the joint distribution.
See also
- Cramér–von Mises test
- Jarque–Bera testJarque–Bera testIn statistics, the Jarque–Bera test is a goodness-of-fit test of whether sample data have the skewness and kurtosis matching a normal distribution. The test is named after Carlos Jarque and Anil K. Bera...
- Kuiper's testKuiper's testKuiper's test is used in statistics to test that whether a given distribution, or family of distributions, is contradicted by evidence from a sample of data. It is named after Dutch mathematician Nicolaas Kuiper....
- Siegel–Tukey test
- Dvoretzky–Kiefer–Wolfowitz inequalityDvoretzky–Kiefer–Wolfowitz inequalityIn the theory of probability and statistics, the Dvoretzky–Kiefer–Wolfowitz inequality predicts how close an empirically determined distribution function will be to the distribution function from which the empirical samples are drawn...
External links
- Short introduction
- KS test explanation
- JavaScript implementation of one- and two-sided tests
- Online calculator with the K-S test
- Open-source C++ code to compute the Kolmogorov distribution and perform the K-S test
- Paper on Evaluating Kolmogorov’s Distribution; contains C implementation. This is the method used in MatlabMATLABMATLAB is a numerical computing environment and fourth-generation programming language. Developed by MathWorks, MATLAB allows matrix manipulations, plotting of functions and data, implementation of algorithms, creation of user interfaces, and interfacing with programs written in other languages,...
.